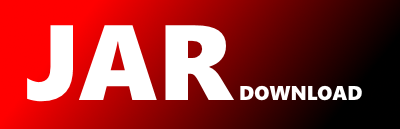
.simplednn.0.5.3.source-code.HANClassifierTest.kt Maven / Gradle / Ivy
Go to download
Show more of this group Show more artifacts with this name
Show all versions of simplednn Show documentation
Show all versions of simplednn Show documentation
SimpleDNN is a machine learning lightweight open-source library written in Kotlin whose purpose is to
support the development of feed-forward and recurrent Artificial Neural Networks.
/* Copyright 2016-present The KotlinNLP Authors. All Rights Reserved.
*
* This Source Code Form is subject to the terms of the Mozilla Public
* License, v. 2.0. If a copy of the MPL was not distributed with this
* file, you can obtain one at http://mozilla.org/MPL/2.0/.
* ------------------------------------------------------------------*/
import com.kotlinnlp.simplednn.core.functionalities.activations.Softmax
import com.kotlinnlp.simplednn.dataset.*
import com.kotlinnlp.simplednn.core.functionalities.activations.Tanh
import com.kotlinnlp.simplednn.core.functionalities.updatemethods.adam.ADAMMethod
import com.kotlinnlp.simplednn.core.layers.LayerType
import com.kotlinnlp.simplednn.core.optimizer.ParamsOptimizer
import com.kotlinnlp.simplednn.deeplearning.attentionnetwork.han.HAN
import com.kotlinnlp.simplednn.deeplearning.attentionnetwork.han.HANEncoder
import com.kotlinnlp.simplednn.deeplearning.attentionnetwork.han.HANParameters
import com.kotlinnlp.simplednn.deeplearning.attentionnetwork.han.toHierarchySequence
import com.kotlinnlp.simplednn.helpers.training.utils.ExamplesIndices
import com.kotlinnlp.simplednn.simplemath.ndarray.dense.DenseNDArray
import com.kotlinnlp.progressindicator.ProgressIndicatorBar
import com.kotlinnlp.simplednn.deeplearning.embeddings.EmbeddingsMap
import utils.CorpusReader
import utils.exampleextractor.ClassificationExampleExtractor
fun main(args: Array) {
println("Start 'HAN Classifier Test'")
val dataset = CorpusReader>().read(
corpusPath = Configuration.loadFromFile().han_classifier.datasets_paths, // same for validation and test
exampleExtractor = ClassificationExampleExtractor(outputSize = 2),
perLine = false)
HANClassifierTest(dataset).start()
println("End.")
}
/**
* Train a HAN classifier with the IMDB movies dataset of encoded documents within a task of sentiment classification (2
* classes) and validate it.
*/
class HANClassifierTest(val dataset: Corpus>) {
/**
* The partition of training set used to train the classifier (the remaining part is used as validation set).
*/
private val trainingSetPartition: Double = 0.9
/**
* The number of epochs for the training.
*/
private val epochs: Int = 10
/**
* The size of the embeddings (used also for the attention arrays).
*/
private val embeddingsSize: Int = 50
/**
* The embeddings associated to each token.
*/
private val embeddings = EmbeddingsMap(size = this.embeddingsSize)
/**
* The [HANEncoder] used as classifier (Softmax output activation).
*/
private val classifier: HANEncoder = this.buildClassifier()
/**
* When timing started.
*/
private var startTime: Long = 0
/**
* Start the test.
*/
fun start() {
println("\n-- TRAINING")
this.train()
println("\n-- TEST")
this.validate(validationSet = this.dataset.test)
}
/**
* @return the HAN classifier
*/
private fun buildClassifier(): HANEncoder {
val model = HAN(
hierarchySize = 1,
inputSize = this.embeddingsSize,
inputType = LayerType.Input.Dense,
biRNNsActivation = Tanh(),
biRNNsConnectionType = LayerType.Connection.RAN,
attentionSize = this.embeddingsSize,
outputSize = 2,
outputActivation = Softmax())
return HANEncoder(model)
}
/**
* Train the HAN classifier, validating each epoch.
*/
private fun train() {
val optimizer = ParamsOptimizer(params = this.classifier.model.params, updateMethod = ADAMMethod(stepSize = 0.005))
val shuffler = Shuffler(enablePseudoRandom = true, seed = 743)
val trainingSize = Math.round(this.dataset.training.size * this.trainingSetPartition).toInt()
val trainingSet = ArrayList(this.dataset.training.subList(0, trainingSize))
val validationSet = ArrayList(this.dataset.training.subList(trainingSize, this.dataset.training.size))
println("Using %d/%d examples as training set and %d/%d as validation set.".format(
trainingSize, this.dataset.training.size, this.dataset.training.size - trainingSize, this.dataset.training.size))
(0 until this.epochs).forEach {
println("\nEpoch ${it + 1}")
this.trainEpoch(optimizer = optimizer, trainingSet = trainingSet, shuffler = shuffler)
println("Epoch validation")
this.validate(validationSet = validationSet)
}
}
/**
* Train the HAN classifier over one epoch.
*
* @param optimizer the optimizer for the classifier
* @param trainingSet the training set
* @param shuffler the [Shuffler] to shuffle examples before training
*/
private fun trainEpoch(optimizer: ParamsOptimizer,
trainingSet: ArrayList>,
shuffler: Shuffler) {
val progress = ProgressIndicatorBar(trainingSet.size)
this.startTiming()
for (exampleIndex in ExamplesIndices(size = trainingSet.size, shuffler = shuffler)) {
progress.tick()
val example = trainingSet[exampleIndex]
val inputSequence = this.extractInputSequence(example)
val output: DenseNDArray = this.classifier.forward(inputSequence.toHierarchySequence())
this.classifier.backward(outputErrors = output.assignSub(example.outputGold), propagateToInput = false)
optimizer.accumulate(this.classifier.getParamsErrors(copy = false))
optimizer.update()
}
println("Elapsed time: %s".format(this.formatElapsedTime()))
}
/**
* Validate the HAN classifier with the example of the given [validationSet].
*
* @param validationSet the validation set
*/
private fun validate(validationSet: ArrayList>) {
var correctPredictions = 0
val progress = ProgressIndicatorBar(validationSet.size)
val exampleIndices = ExamplesIndices(
size = validationSet.size,
shuffler = Shuffler(enablePseudoRandom = true, seed = 1)
)
this.startTiming()
for (exampleIndex in exampleIndices) {
progress.tick()
correctPredictions += this.validateExample(example = validationSet[exampleIndex])
}
println("Elapsed time: %s".format(this.formatElapsedTime()))
println("Accuracy: %.2f%%".format(100.0 * correctPredictions / validationSet.size))
}
/**
* Validate the HAN classifier with the given [example].
*
* @param example an example of the validation dataset
*
* @return 1 if the prediction is correct, 0 otherwise
*/
private fun validateExample(example: SimpleExample): Int {
val inputSequence = this.extractInputSequence(example)
val output: DenseNDArray = this.classifier.forward(inputSequence.toHierarchySequence())
return if (this.predictionIsCorrect(output, example.outputGold)) 1 else 0
}
/**
* @param example an example of the dataset
*
* @return an array of embeddings vectors associated to each feature (casted to Int) of the [example]
*/
private fun extractInputSequence(example: SimpleExample): Array {
return Array(
size = example.features.length,
init = { i ->
val wordIndex = example.features[i].toInt()
this.embeddings.getOrSet(wordIndex).array.values
}
)
}
/**
* @param output an output prediction of the HAN classifier
* @param goldOutput the expected gold output
*
* @return a Boolean indicating if the [output] matches the [goldOutput]
*/
private fun predictionIsCorrect(output: DenseNDArray, goldOutput: DenseNDArray): Boolean {
return output.argMaxIndex() == goldOutput.argMaxIndex()
}
/**
* Start registering time.
*/
private fun startTiming() {
this.startTime = System.currentTimeMillis()
}
/**
* @return the formatted string with elapsed time in seconds and minutes.
*/
private fun formatElapsedTime(): String {
val elapsedTime = System.currentTimeMillis() - this.startTime
val elapsedSecs = elapsedTime / 1000.0
return "%.3f s (%.1f min)".format(elapsedSecs, elapsedSecs / 60.0)
}
}
© 2015 - 2025 Weber Informatics LLC | Privacy Policy