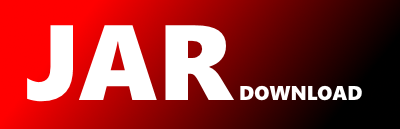
.simplednn.0.5.3.source-code.VectorsAverageBiaffineTest.kt Maven / Gradle / Ivy
/* Copyright 2016-present The KotlinNLP Authors. All Rights Reserved.
*
* This Source Code Form is subject to the terms of the Mozilla Public
* License, v. 2.0. If a copy of the MPL was not distributed with this
* file, you can obtain one at http://mozilla.org/MPL/2.0/.
* ------------------------------------------------------------------*/
import com.kotlinnlp.simplednn.core.functionalities.updatemethods.adam.ADAMMethod
import com.kotlinnlp.simplednn.core.optimizer.ParamsOptimizer
import com.kotlinnlp.simplednn.dataset.Shuffler
import com.kotlinnlp.simplednn.deeplearning.mergelayers.biaffine.BiaffineLayerParameters
import com.kotlinnlp.simplednn.deeplearning.mergelayers.biaffine.BiaffineLayerStructure
import com.kotlinnlp.simplednn.helpers.training.utils.ExamplesIndices
import com.kotlinnlp.simplednn.simplemath.ndarray.dense.DenseNDArray
import com.kotlinnlp.simplednn.simplemath.ndarray.dense.DenseNDArrayFactory
import java.io.File
fun main(args: Array) {
println("Start 'Vectors Average Biaffine Test'")
val corpusPath = Configuration.loadFromFile().vectors_average.datasets_paths
VectorsAverageBiaffineTest(corpusPath.training).start()
println("\nEnd.")
}
typealias Example = Triple
/**
*
*/
class VectorsAverageBiaffineTest(private val trainingSetPath: String) {
/**
*
*/
private val shuffler = Shuffler()
/**
*
*/
private val biaffineLayer = BiaffineLayerStructure(
params = BiaffineLayerParameters(
inputSize1 = 5,
inputSize2 = 5,
outputSize = 5))
/**
*
*/
private val paramsErrors = this.biaffineLayer.params.copy()
/**
*
*/
private val optimizer = ParamsOptimizer(
params = this.biaffineLayer.params,
updateMethod = ADAMMethod(stepSize = 0.001, beta1 = 0.99, beta2 = 0.99999))
/**
*
*/
fun start() {
val dataset: ArrayList = this.loadExamples()
val testSetSize: Int = Math.round(dataset.size * 0.1).toInt()
val testSet = dataset.subList(fromIndex = 0, toIndex = testSetSize)
val trainingSet = dataset.subList(fromIndex = testSetSize, toIndex = dataset.size)
val epochs = 25
println("\n-- TRAINING ON ${trainingSet.size} EXAMPLES")
(0 until epochs).forEach { epoch ->
println("\nEpoch ${epoch + 1} of $epochs")
this.trainEpoch(trainingExamples = trainingSet)
println("\nValidation on ${testSet.size} examples")
println("Accuracy: %.2f%%".format(100 * this.validate(testSet)))
}
}
/**
*
*/
private fun loadExamples(): ArrayList {
val examples = arrayListOf()
File(this.trainingSetPath).forEachLine { line ->
val data: List = line.split(",").map { it.toDouble() }
val input1 = DenseNDArrayFactory.arrayOf(data.subList(0, 5).toDoubleArray())
val input2 = DenseNDArrayFactory.arrayOf(data.subList(5, 10).toDoubleArray())
val output = DenseNDArrayFactory.arrayOf(data.subList(10, 15).toDoubleArray())
examples.add(Triple(input1, input2, output))
}
return examples
}
/**
*
*/
private fun trainEpoch(trainingExamples: List) {
this.loopExamples(trainingExamples) { example ->
this.optimizer.newEpoch()
this.trainExample(example)
}
}
/**
*
*/
private fun validate(testExamples: List): Double {
var correctPredictions = 0
this.loopExamples(testExamples) { example ->
val output: DenseNDArray = this.predict(example)
if (example.third.equals(output, tolerance = 0.01)) {
correctPredictions++
}
}
return correctPredictions.toDouble() / testExamples.size
}
/**
*
*/
private fun trainExample(example: Example) {
this.optimizer.newBatch()
this.optimizer.newExample()
this.biaffineLayer.setErrors(errors = this.predict(example).sub(example.third))
this.biaffineLayer.backward(this.paramsErrors, propagateToInput = false, mePropK = null)
this.optimizer.accumulate(this.paramsErrors)
this.optimizer.update()
}
/**
*
*/
private fun predict(example: Example): DenseNDArray {
this.biaffineLayer.setInput1(example.first)
this.biaffineLayer.setInput2(example.second)
this.biaffineLayer.forward()
return this.biaffineLayer.outputArray.values
}
/**
*
*/
private fun loopExamples(examples: List, callback: (example: Example) -> Unit) {
for (exampleIndex in ExamplesIndices(examples.size, shuffler = this.shuffler)) {
callback(examples[exampleIndex])
}
}
}
© 2015 - 2025 Weber Informatics LLC | Privacy Policy