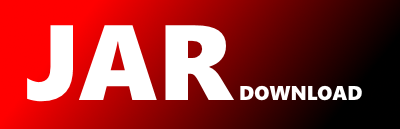
ai.djl.spark.task.binary.BinaryPredictor.scala Maven / Gradle / Ivy
Go to download
Show more of this group Show more artifacts with this name
Show all versions of spark Show documentation
Show all versions of spark Show documentation
Apache Spark integration for DJL
The newest version!
/*
* Copyright 2023 Amazon.com, Inc. or its affiliates. All Rights Reserved.
*
* Licensed under the Apache License, Version 2.0 (the "License"). You may not use this file except in compliance
* with the License. A copy of the License is located at
*
* http://aws.amazon.com/apache2.0/
*
* or in the "license" file accompanying this file. This file is distributed on an "AS IS" BASIS, WITHOUT WARRANTIES
* OR CONDITIONS OF ANY KIND, either express or implied. See the License for the specific language governing permissions
* and limitations under the License.
*/
package ai.djl.spark.task.binary
import ai.djl.spark.task.BasePredictor
import ai.djl.spark.translator.binary.NpBinaryTranslator
import org.apache.spark.ml.param.Param
import org.apache.spark.ml.param.shared.{HasInputCol, HasOutputCol}
import org.apache.spark.ml.util.Identifiable
import org.apache.spark.sql.types._
import org.apache.spark.sql.{DataFrame, Dataset, Row}
/**
* BinaryPredictor performs prediction on binary input.
*
* @param uid An immutable unique ID for the object and its derivatives.
*/
class BinaryPredictor(override val uid: String) extends BasePredictor[Array[Byte], Array[Byte]]
with HasInputCol with HasOutputCol {
def this() = this(Identifiable.randomUID("BinaryPredictor"))
final val batchifier = new Param[String](this, "batchifier",
"The batchifier. Valid values include none (default), stack, and padding.")
private var inputColIndex : Int = _
/**
* Sets the inputCol parameter.
*
* @param value the value of the parameter
*/
def setInputCol(value: String): this.type = set(inputCol, value)
/**
* Sets the outputCol parameter.
*
* @param value the value of the parameter
*/
def setOutputCol(value: String): this.type = set(outputCol, value)
/**
* Sets the batchifier parameter.
*
* @param value the value of the parameter
*/
def setBatchifier(value: String): this.type = set(batchifier, value)
setDefault(inputClass, classOf[Array[Byte]])
setDefault(outputClass, classOf[Array[Byte]])
setDefault(batchifier, "none")
/**
* Performs prediction on the provided dataset.
*
* @param dataset input dataset
* @return output dataset
*/
def predict(dataset: Dataset[_]): DataFrame = {
transform(dataset)
}
/** @inheritdoc */
override def transform(dataset: Dataset[_]): DataFrame = {
setDefault(translator, new NpBinaryTranslator($(batchifier)))
inputColIndex = dataset.schema.fieldIndex($(inputCol))
super.transform(dataset)
}
/** @inheritdoc */
override protected def transformRows(iter: Iterator[Row]): Iterator[Row] = {
val predictor = model.newPredictor($(translator))
iter.map(row => {
Row.fromSeq(row.toSeq ++ Array[Any](predictor.predict(row.getAs[Array[Byte]](inputColIndex))))
})
}
/** @inheritdoc */
override def transformSchema(schema: StructType): StructType = {
validateInputType(schema($(inputCol)))
val outputSchema = StructType(schema.fields ++
Array(StructField($(outputCol), BinaryType)))
outputSchema
}
def validateInputType(input: StructField): Unit = {
require(input.dataType == BinaryType,
s"Input column ${input.name} type must be BinaryType but got ${input.dataType}.")
}
}
© 2015 - 2024 Weber Informatics LLC | Privacy Policy