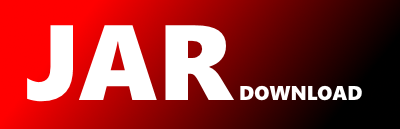
hex.glm.GLMUtils Maven / Gradle / Ivy
package hex.glm;
import water.DKV;
import water.Key;
import water.MemoryManager;
import water.fvec.Frame;
import water.fvec.Vec;
import water.util.ArrayUtils;
import water.util.FrameUtils;
import water.util.TwoDimTable;
import java.util.ArrayList;
import java.util.Arrays;
import java.util.List;
import java.util.stream.Collectors;
import java.util.stream.Stream;
import static hex.glm.GLMModel.GLMParameters.Family.gaussian;
import static water.fvec.Vec.T_NUM;
import static water.fvec.Vec.T_STR;
public class GLMUtils {
/***
* From the gamColnames, this method attempts to translate to the column indices in adaptFrame.
* @param adaptFrame
* @param gamColnames
* @return
*/
public static int[][] extractAdaptedFrameIndices(Frame adaptFrame, String[][] gamColnames, int numOffset) {
String[] frameNames = adaptFrame.names();
List allColNames = new ArrayList<>();
for (String name:frameNames)
allColNames.add(name);
int[][] gamColIndices = new int[gamColnames.length][];
int numFrame = gamColnames.length;
for (int frameNum=0; frameNum < numFrame; frameNum++) {
int numCols = gamColnames[frameNum].length;
gamColIndices[frameNum] = MemoryManager.malloc4(numCols);
for (int index=0; index < numCols; index++) {
gamColIndices[frameNum][index] = numOffset+allColNames.indexOf(gamColnames[frameNum][index]);
}
}
return gamColIndices;
}
public static GLMModel.GLMParameters[] genGLMParameters(GLMModel.GLMParameters parms, String[] validPreds,
String[] predictorNames) {
int numPreds = validPreds.length;
if (numPreds > 0) {
GLMModel.GLMParameters[] params = new GLMModel.GLMParameters[numPreds];
String[] frameNames = parms.train().names();
List predList = Stream.of(predictorNames).collect(Collectors.toList());
if (parms._weights_column != null)
predList.add(parms._weights_column);
String[] ignoredCols = Arrays.stream(frameNames).filter(x -> !predList.contains(x)).collect(Collectors.toList()).toArray(new String[0]);
for (int index=0; index < numPreds; index++) {
params[index] = new GLMModel.GLMParameters(gaussian);
params[index]._response_column = validPreds[index];
params[index]._train = parms.train()._key;
params[index]._lambda = new double[]{0.0};
params[index]._alpha = new double[]{0.0};
params[index]._compute_p_values = true;
params[index]._ignored_columns = ignoredCols;
params[index]._weights_column = parms._weights_column;
}
return params;
} else {
return null;
}
}
public static void removePredictors(GLMModel.GLMParameters parms, Frame train) {
List nonPredictors = Arrays.stream(parms.getNonPredictors()).collect(Collectors.toList());
String[] colNames = parms.train().names();
List removeCols = Arrays.stream(colNames).filter(x -> !nonPredictors.contains(x)).collect(Collectors.toList());
for (String removeC : removeCols)
train.remove(removeC);
}
public static Frame expandedCatCS(Frame beta_constraints, GLMModel.GLMParameters parms) {
byte[] csByteType = new byte[]{T_STR, T_NUM, T_NUM};
String[] bsColNames = beta_constraints.names();
Frame betaCSCopy = beta_constraints.deepCopy(Key.make().toString());
betaCSCopy.replace(0, betaCSCopy.vec(0).toStringVec()).remove();
DKV.put(betaCSCopy);
FrameUtils.ExpandCatBetaConstraints expandCatBS = new FrameUtils.ExpandCatBetaConstraints(beta_constraints,
parms.train()).doAll(csByteType, betaCSCopy, true);
Frame csWithEnum = expandCatBS.outputFrame(Key.make(), bsColNames, null);
betaCSCopy.delete();
return csWithEnum;
}
public static boolean findEnumInBetaCS(Frame betaCS, GLMModel.GLMParameters parms) {
List colNames = Arrays.asList(parms.train().names());
String[] types = parms.train().typesStr();
Vec v = betaCS.vec("names");
int nRow = (int) betaCS.numRows();
for (int index=0; index= 0 && "Enum".equals(types[colIndex]))
return true;
}
return false;
}
public static GLM.GLMGradientInfo copyGInfo(GLM.GLMGradientInfo ginfo) {
double[] gradient = ginfo._gradient.clone();
GLM.GLMGradientInfo tempGinfo = new GLM.GLMGradientInfo(ginfo._likelihood, ginfo._objVal, gradient);
return tempGinfo;
}
public static TwoDimTable combineScoringHistory(TwoDimTable glmSc1, TwoDimTable earlyStopSc2) {
String[] esColTypes = earlyStopSc2.getColTypes();
String[] esColFormats = earlyStopSc2.getColFormats();
List finalColHeaders = new ArrayList<>(Arrays.asList(glmSc1.getColHeaders()));
final List earlyStopScHeaders = new ArrayList<>(Arrays.asList(earlyStopSc2.getColHeaders()));
final int overlapSize = 3; // for "Timestamp", "Duration", "Iterations
int earlyStopSCIterIndex = earlyStopScHeaders.indexOf("Iterations");
int indexOfIter = finalColHeaders.indexOf("iteration");
if (indexOfIter < 0)
indexOfIter = finalColHeaders.indexOf("iterations");
List finalColTypes = new ArrayList<>(Arrays.asList(glmSc1.getColTypes()));
List finalColFormats = new ArrayList<>(Arrays.asList(glmSc1.getColFormats()));
List earlyStopColIndices = new ArrayList<>();
int colCounter = 0;
for (String colName : earlyStopSc2.getColHeaders()) { // collect final table colHeaders, RowHeaders, ColFormats, ColTypes
if (!finalColHeaders.contains(colName.toLowerCase())) {
finalColHeaders.add(colName);
finalColTypes.add(esColTypes[colCounter]);
finalColFormats.add(esColFormats[colCounter]);
earlyStopColIndices.add(colCounter);
}
colCounter++;
}
final int tableSize = finalColHeaders.size();
String[] rowHeaders = generateRowHeaders(glmSc1, earlyStopSc2, indexOfIter, earlyStopSCIterIndex);
TwoDimTable res = new TwoDimTable("Scoring History", "",
rowHeaders, finalColHeaders.toArray(new String[tableSize]), finalColTypes.toArray(new String[tableSize]),
finalColFormats.toArray(new String[tableSize]), "");
res = combineTableContents(glmSc1, earlyStopSc2, res, earlyStopColIndices, indexOfIter, earlyStopSCIterIndex,
overlapSize);
return res;
}
public static String[] generateRowHeaders(TwoDimTable glmSc1, TwoDimTable earlyStopSc2, int glmIterIndex,
int earlyStopIterIndex) {
int glmRowSize = glmSc1.getRowDim();
int earlyStopRowSize = earlyStopSc2.getRowDim();
List iterList = new ArrayList<>();
for (int index = 0; index < glmRowSize; index++)
iterList.add((Integer) glmSc1.get(index, glmIterIndex));
for (int index = 0; index < earlyStopRowSize; index++) {
Integer iter = (Integer) earlyStopSc2.get(index, earlyStopIterIndex);
if (!iterList.contains(iter))
iterList.add(iter);
}
String[] rowHeader = new String[iterList.size()];
for (int index=0; index < rowHeader.length; index++)
rowHeader[index] = "";
return rowHeader;
}
// glmSc1 is updated for every iteration while earlyStopSc2 is updated per scoring interval. Hence, glmSc1 is
// very likely to be longer than earlyStopSc2. We only add earlyStopSc2 to the table when the iteration
// indices align with each other.
public static TwoDimTable combineTableContents(final TwoDimTable glmSc1, final TwoDimTable earlyStopSc2,
TwoDimTable combined, final List earlyStopColIndices,
final int indexOfIter, final int indexOfIterEarlyStop,
final int overlapSize) {
final int rowSize = glmSc1.getRowDim(); // array size from GLM Scoring, contains more iterations
final int rowSize2 = earlyStopSc2.getRowDim(); // array size from scoringHistory
final int glmColSize = glmSc1.getColDim();
final int earlyStopColSize = earlyStopColIndices.size();
int sc2RowIndex = 0;
int glmRowIndex = 0;
int rowIndex = 0;
List iterRecorded = new ArrayList<>();
while ((sc2RowIndex < rowSize2) && (glmRowIndex < rowSize)) {
int glmScIter = (int) glmSc1.get(glmRowIndex, indexOfIter);
int earlyStopScIter = (int) earlyStopSc2.get(sc2RowIndex, indexOfIterEarlyStop);
if (glmScIter == earlyStopScIter) {
if (!iterRecorded.contains(glmScIter)) {
addOneRow2ScoringHistory(glmSc1, earlyStopSc2, glmColSize, earlyStopColSize, glmRowIndex, sc2RowIndex,
rowIndex, true, true, earlyStopColIndices, combined, overlapSize);
iterRecorded.add(glmScIter);
}
sc2RowIndex++;
glmRowIndex++;
} else if (glmScIter < earlyStopScIter) { // add GLM scoring history
if (!iterRecorded.contains(glmScIter)) {
addOneRow2ScoringHistory(glmSc1, earlyStopSc2, glmColSize, earlyStopColSize, glmRowIndex, sc2RowIndex, rowIndex,
true, false, earlyStopColIndices, combined, overlapSize);
iterRecorded.add(glmScIter);
}
glmRowIndex++;
} else if (glmScIter > earlyStopScIter) { // add GLM scoring history
if (!iterRecorded.contains(earlyStopScIter)) {
addOneRow2ScoringHistory(glmSc1, earlyStopSc2, glmColSize, earlyStopColSize, glmRowIndex, sc2RowIndex, rowIndex,
false, true, earlyStopColIndices, combined, overlapSize);
iterRecorded.add(earlyStopScIter);
}
sc2RowIndex++;
}
rowIndex++;
}
for (int index = glmRowIndex; index < rowSize; index++) { // add left over glm scoring history
int iter = (int) glmSc1.get(index, indexOfIter);
if (!iterRecorded.contains(iter) && iterRecorded.get(iterRecorded.size()-1) < iter) {
addOneRow2ScoringHistory(glmSc1, earlyStopSc2, glmColSize, earlyStopColSize, index, -1,
rowIndex++, true, false, earlyStopColIndices, combined, overlapSize);
iterRecorded.add(iter);
}
}
for (int index = sc2RowIndex; index < rowSize2; index++) { // add left over scoring history
int iter = (int) earlyStopSc2.get(index, indexOfIterEarlyStop);
if (!iterRecorded.contains(iter) && iterRecorded.get(iterRecorded.size()-1) < iter) {
addOneRow2ScoringHistory(glmSc1, earlyStopSc2, glmColSize, earlyStopColSize, -1, index, rowIndex++,
false, true, earlyStopColIndices, combined, overlapSize);
iterRecorded.add(iter);
}
}
return combined;
}
public static void addOneRow2ScoringHistory(final TwoDimTable glmSc1, final TwoDimTable earlyStopSc2, int glmColSize,
int earlyStopColSize, int glmRowIndex, int earlyStopRowIndex, int rowIndex,
boolean addGlmSC, boolean addEarlyStopSC,
final List earlyStopColIndices, TwoDimTable combined,
final int overlapSize) {
if (addGlmSC)
for (int glmIndex = 0; glmIndex < glmColSize; glmIndex++)
combined.set(rowIndex, glmIndex, glmSc1.get(glmRowIndex, glmIndex));
if (addEarlyStopSC)
for (int earlyStopIndex = 0; earlyStopIndex < earlyStopColSize; earlyStopIndex++) {
if (!addGlmSC && earlyStopIndex < overlapSize)
combined.set(rowIndex, earlyStopIndex, earlyStopSc2.get(earlyStopRowIndex, earlyStopIndex));
combined.set(rowIndex, earlyStopIndex + glmColSize, earlyStopSc2.get(earlyStopRowIndex,
earlyStopColIndices.get(earlyStopIndex)));
}
}
public static void updateGradGam(double[] gradient, double[][][] penalty_mat, int[][] gamBetaIndices, double[] beta,
int[] activeCols) { // update gradient due to gam smoothness constraint
int numGamCol = gamBetaIndices.length; // number of predictors used for gam
for (int gamColInd = 0; gamColInd < numGamCol; gamColInd++) { // update each gam col separately
int penaltyMatSize = penalty_mat[gamColInd].length;
for (int betaInd = 0; betaInd < penaltyMatSize; betaInd++) { // derivative of each beta in penalty matrix
int currentBetaIndex = gamBetaIndices[gamColInd][betaInd];
if (activeCols != null) {
currentBetaIndex = ArrayUtils.find(activeCols, currentBetaIndex);
}
if (currentBetaIndex >= 0) { // only add if coefficient is active
double tempGrad = 2 * beta[currentBetaIndex] * penalty_mat[gamColInd][betaInd][betaInd];
for (int rowInd = 0; rowInd < penaltyMatSize; rowInd++) {
if (rowInd != betaInd) {
int currBetaInd = gamBetaIndices[gamColInd][rowInd];
if (activeCols != null) {
currBetaInd = ArrayUtils.find(activeCols, currBetaInd);
}
if (currBetaInd >= 0)
tempGrad += beta[currBetaInd] * penalty_mat[gamColInd][betaInd][rowInd];
}
}
gradient[currentBetaIndex] += tempGrad;
}
}
}
}
// Note that gradient is [ncoeff][nclass].
public static void updateGradGamMultinomial(double[][] gradient, double[][][] penaltyMat, int[][] gamBetaIndices,
double[][] beta) {
int numClass = beta[0].length;
int numGamCol = gamBetaIndices.length;
for (int classInd = 0; classInd < numClass; classInd++) {
for (int gamInd = 0; gamInd < numGamCol; gamInd++) {
int numKnots = gamBetaIndices[gamInd].length;
for (int rowInd = 0; rowInd < numKnots; rowInd++) { // calculate dpenalty/dbeta rowInd
double temp = 0.0;
int betaIndR = gamBetaIndices[gamInd][rowInd]; // dGradient/dbeta_betaIndR
for (int colInd = 0; colInd < numKnots; colInd++) {
int betaIndC = gamBetaIndices[gamInd][colInd];
temp += (betaIndC==betaIndR)?(2*penaltyMat[gamInd][rowInd][colInd]*beta[betaIndC][classInd])
:penaltyMat[gamInd][rowInd][colInd]*beta[betaIndC][classInd];
}
gradient[betaIndR][classInd] += temp;
}
}
}
}
public static double calSmoothNess(double[] beta, double[][][] penaltyMatrix, int[][] gamColIndices) {
int numGamCols = gamColIndices.length;
double smoothval = 0;
for (int gamCol=0; gamCol < numGamCols; gamCol++) {
smoothval += ArrayUtils.innerProductPartial(beta, gamColIndices[gamCol],
ArrayUtils.multArrVecPartial(penaltyMatrix[gamCol], beta, gamColIndices[gamCol]));
}
return smoothval;
}
/**
*
* @param beta multinomial number of class by number of predictors
* @param penaltyMatrix
* @param gamColIndices
* @return
*/
public static double calSmoothNess(double[][] beta, double[][][] penaltyMatrix, int[][] gamColIndices) {
int numClass = beta.length;
double smoothval=0;
for (int classInd=0; classInd < numClass; classInd++) {
smoothval += calSmoothNess(beta[classInd], penaltyMatrix, gamColIndices);
}
return smoothval;
}
public static String[] genDfbetasNames(GLMModel model) {
double[] stdErr = model._output.stdErr();
String[] names = Arrays.stream(model._output.coefficientNames()).map(x -> "DFBETA_"+x).toArray(String[]::new);
List namesList = new ArrayList<>();
int numCoeff = names.length;
for (int index=0; index
© 2015 - 2025 Weber Informatics LLC | Privacy Policy