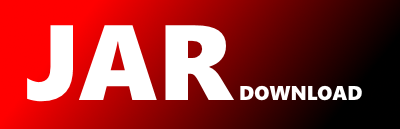
au.csiro.pathling.sql.Plans.scala Maven / Gradle / Ivy
Go to download
Show more of this group Show more artifacts with this name
Show all versions of terminology Show documentation
Show all versions of terminology Show documentation
Interact with a FHIR terminology server from Spark.
/*
* Copyright 2022 Commonwealth Scientific and Industrial Research
* Organisation (CSIRO) ABN 41 687 119 230.
*
* Licensed under the Apache License, Version 2.0 (the "License");
* you may not use this file except in compliance with the License.
* You may obtain a copy of the License at
*
* http://www.apache.org/licenses/LICENSE-2.0
*
* Unless required by applicable law or agreed to in writing, software
* distributed under the License is distributed on an "AS IS" BASIS,
* WITHOUT WARRANTIES OR CONDITIONS OF ANY KIND, either express or implied.
* See the License for the specific language governing permissions and
* limitations under the License.
*/
package au.csiro.pathling.sql
import org.apache.spark.rdd.RDD
import org.apache.spark.sql._
import org.apache.spark.sql.catalyst.InternalRow
import org.apache.spark.sql.catalyst.encoders.RowEncoder
import org.apache.spark.sql.catalyst.expressions.codegen.GenerateUnsafeRowJoiner
import org.apache.spark.sql.catalyst.expressions.{Attribute, Expression, ExpressionSet, NamedExpression, UnsafeRow}
import org.apache.spark.sql.catalyst.plans.logical.LogicalPlan
import org.apache.spark.sql.catalyst.plans.physical.Partitioning
import org.apache.spark.sql.catalyst.trees.UnaryLike
import org.apache.spark.sql.execution._
import org.apache.spark.sql.types.{StructField, StructType}
import scala.collection.JavaConverters._
/**
* This wrapper is needed to make sure that serializing expression is not de-aliased (and it remains
* a valid encoder).
*
* @param value the expression to wrap
*/
case class ExpressionWrapper(value: Seq[NamedExpression])
object MapWithPartitionPreview {
/**
* Creates a `MapWithPartitionPreview` from Java API objects.
*
* @param deserializer the expression to use to extract the value to be mapped from the result
* of the child plan. The type of this value depends on the type of the
* expression and is a 'raw' spark SQL representation (e.g. InternalRow for
* structs, etc).
* @param decoder the `ObjectDecoder` to convert the 'raw' value of the `deserializer`
* expression to a Java/Scala type `[I]` expected by the `previewMapper`
* @param previewMapper the `MapWithPartitionPreview` to use for state creation and mapping
* @param resultField the `StructField` that describes the name of the type of the column with
* the mapper result in the output dataset.
* @param child the child `LogicalPlan`
* @tparam I type of the mapper input
* @tparam R type of the mapper result
* @tparam S type of the per partition state object
* @return
*/
def fromJava[I, R, S](deserializer: Expression, decoder: ObjectDecoder[I],
previewMapper: MapperWithPreview[I, R, S],
resultField: StructField,
child: LogicalPlan): MapWithPartitionPreview = {
val elementSchema = new StructType(Array(resultField))
val encoder = RowEncoder(elementSchema)
val serializer: Seq[NamedExpression] = encoder.serializer
val preview: Iterator[I] => S = it => previewMapper.preview(it.asJava)
val mapper: (I, S) => R = previewMapper.call
val expressionDecoder: Any => I = decoder.decode
MapWithPartitionPreview(ExpressionWrapper(serializer),
expressionDecoder.asInstanceOf[Any => Any],
deserializer,
preview.asInstanceOf[Iterator[Any] => Any],
mapper.asInstanceOf[(Any, Any) => Any],
child)
}
}
/**
* A logical plan for the operation that appends the column with the result of mapping the value of
* a `deserializer` expression with the `mapper` function for each row to the child dataset.
*
* In addition to the value to map, the `mapper` function also receives an arbitrary state object
* created by the `preview` function based all values of `deserializer` expression from all rows in
* the current partition.
*
* The raw Spark values deserialized from rows with the `deserializer` expression are converted
* to Java/Scala objects with the `decoder` function, before being passed to `preview` and `mapper`
* respectively.
*
* @impNote This is based on {`org.apache.spark.sql.catalyst.plans.logical.AppendColumns`}.
* Originally it extended {`org.apache.spark.sql.catalyst.plans.logical.UnaryNode`}
* but that was causing problems in Databricks environments (it appears they are using a
* customized version of catalyst where UnaryNode is an abstract class rather than a trait.
* So now instead we are inheriting directly from
* {`org.apache.spark.sql.catalyst.plans.logical.LogicalPlan`}
* and mixing in {`org.apache.spark.sql.catalyst.plans.logical.UnaryLike`} just as
* {`org.apache.spark.sql.catalyst.plans.logical.UnaryNode`} does.
* @param serializer the function that converts the mapper result to the new column to be appended
* to the child produced dataset.
* @param decoder the function that converts the 'raw' spark sql object produced by
* `deserializer` to a Java/Scala type expected by `mapper`
* @param deserializer The expression to use to extract the value to be mapped from the result of
* the child plan. The type of this value depends on the type of the expression
* and is a 'raw' spark SQL representation (e.g. `InternalRow` for structs,
* etc).
* @param preview the function to produce the state for the `mapper` from all the rows in the
* partition
* @param mapper The function to map the value extracted from each row and the per-partition
* state to the result. Currently the result needs to be a 'raw' spark sql type
* that can be used with `Row(...)`.
* @param child the child `LogicalPlan`
*
*/
case class MapWithPartitionPreview(serializer: ExpressionWrapper, decoder: Any => Any,
deserializer: Expression,
preview: Iterator[Any] => Any,
mapper: (Any, Any) => Any,
child: LogicalPlan)
extends LogicalPlan with UnaryLike[LogicalPlan] {
override def output: Seq[Attribute] = child.output ++ newColumns
def newColumns: Seq[Attribute] = serializer.value.map(_.toAttribute)
override protected def withNewChildInternal(newChild: LogicalPlan): LogicalPlan = {
MapWithPartitionPreview(serializer, decoder, deserializer, preview, mapper, newChild)
}
override protected lazy val validConstraints: ExpressionSet = child.constraints
}
/**
* A physical plan for executing `MapWithPartitionPreview`.
*
* This is based on `AppendColumnsExec`.
*
* @param serializer the function that converts the mapper result to the new column to be
* appended to the child produced dataset
* @param expressionDecoder the function that converts 'raw' spark sql object produced by the
* `deserializer` to a Java/Scala type expected by `mapper`
* @param deserializer The expression to use to extract the value to be mapped from the result
* of the child plan. The type of this value depends on the type of the
* expression and is a 'raw' spark SQL representation (e.g. `InternalRow`
* for structs, etc).
* @param preview the function to produce the state for the `mapper` from all the rows in
* the partition
* @param mapper The function to map the value extracted from each row and the
* per-partition state to the result. Currently the result needs to be a
* 'raw' spark sql type, that can be used with `Row(...)`.
* @param child the child `SparkPlan`
*/
case class MapWithPartitionPreviewExec(deserializer: Expression,
expressionDecoder: Any => Any,
serializer: Seq[NamedExpression],
preview: Iterator[Any] => Any,
mapper: (Any, Any) => Any,
child: SparkPlan)
extends UnaryExecNode {
def elementSchema: StructType = serializer.map(_.toAttribute).toStructType
override def outputPartitioning: Partitioning = child.outputPartitioning
override protected def doExecute(): RDD[InternalRow] = {
child.execute().mapPartitions { it =>
val getObject = ObjectOperator.deserializeRowToObject(deserializer, child.output)
// Cache all rows in the partition and the decoded objects.
val allRowsAndObjects = it.map(r => (r.copy(), expressionDecoder(getObject(r)))).toSeq
// Pass the decoded objects to the `preview` function to create the per partition state.
val state = preview(allRowsAndObjects.map(_._2).toIterator)
val combiner = GenerateUnsafeRowJoiner.create(child.schema, elementSchema)
val outputObject = ObjectOperator.serializeObjectToRow(serializer)
// Map decoded object and the state with mapper` and append the resulting columns to input
// rows.
allRowsAndObjects.toIterator.map { case (row, obj) =>
val newColumns = outputObject(Row(mapper(obj, state)))
combiner.join(row.asInstanceOf[UnsafeRow], newColumns): InternalRow
}
}
}
override def output: Seq[Attribute] = child.output ++ serializer.map(_.toAttribute)
override protected def withNewChildInternal(newChild: SparkPlan): SparkPlan = {
MapWithPartitionPreviewExec(deserializer, expressionDecoder, serializer, preview, mapper,
newChild)
}
}
© 2015 - 2024 Weber Informatics LLC | Privacy Policy