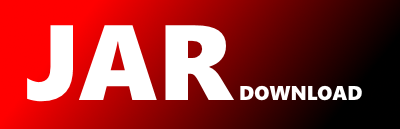
umontreal.iro.lecuyer.probdist.KolmogorovSmirnovDistQuick Maven / Gradle / Ivy
Go to download
Show more of this group Show more artifacts with this name
Show all versions of ssj Show documentation
Show all versions of ssj Show documentation
SSJ is a Java library for stochastic simulation, developed under the direction of Pierre L'Ecuyer,
in the Département d'Informatique et de Recherche Opérationnelle (DIRO), at the Université de Montréal.
It provides facilities for generating uniform and nonuniform random variates, computing different
measures related to probability distributions, performing goodness-of-fit tests, applying quasi-Monte
Carlo methods, collecting (elementary) statistics, and programming discrete-event simulations with both
events and processes.
The newest version!
/*
* Class: KolmogorovSmirnovDistQuick
* Description: Kolmogorov-Smirnov 2-sided 1-sample distribution
* Environment: Java
* Software: SSJ
* Copyright (C) 2001 Pierre L'Ecuyer and Université de Montréal
* Organization: DIRO, Université de Montréal
* @author Richard Simard
* @since January 2010
* SSJ is free software: you can redistribute it and/or modify it under
* the terms of the GNU General Public License (GPL) as published by the
* Free Software Foundation, either version 3 of the License, or
* any later version.
* SSJ is distributed in the hope that it will be useful,
* but WITHOUT ANY WARRANTY; without even the implied warranty of
* MERCHANTABILITY or FITNESS FOR A PARTICULAR PURPOSE. See the
* GNU General Public License for more details.
* A copy of the GNU General Public License is available at
GPL licence site.
*/
package umontreal.iro.lecuyer.probdist;
import umontreal.iro.lecuyer.util.*;
import umontreal.iro.lecuyer.functions.MathFunction;
/**
* Extends the class {@link KolmogorovSmirnovDist} for the distribution.
* The methods of this class are much faster than those of class
* {@link KolmogorovSmirnovDist}.
*
*/
public class KolmogorovSmirnovDistQuick extends KolmogorovSmirnovDist {
/*
For n <= NEXACT, we use exact algorithms: the Durbin matrix and
the Pomeranz algorithms. For n > NEXACT, we use asymptotic methods
except for x close to 0 where we still use the method of Durbin
for n <= NKOLMO. For n > NKOLMO, we use asymptotic methods only and
so the precision is less for x close to 0.
We could increase the limit NKOLMO to 10^6 to get better precision
for x close to 0, but at the price of a slower speed.
*/
private static final int NKOLMO = 100000;
private static class Function implements MathFunction {
protected int n;
protected double u;
public Function (int n, double u) {
this.n = n;
this.u = u;
}
public double evaluate (double x) {
return u - cdf(n,x);
}
}
/**
* Constructs a distribution with parameter n.
*
*/
public KolmogorovSmirnovDistQuick (int n) {
super (n);
}
public double density (double x) {
return density (n, x);
}
public double cdf (double x) {
return cdf (n, x);
}
public double barF (double x) {
return barF (n, x);
}
public double inverseF (double u) {
return inverseF (n, u);
}
/**
* Computes the density for the distribution with parameter n.
*
*/
public static double density (int n, double x) {
double Res = densConnue(n,x);
if (Res != -1.0)
return Res;
final double EPS = 1.0 / Num.TWOEXP[6];
Res = (cdf(n, x + EPS) - cdf(n, x - EPS)) / (2.0 * EPS);
if (Res <= 0.0)
return 0.0;
return Res;
}
private static double Pelz (int n, double x) {
/* Approximating the Lower Tail-Areas of the Kolmogorov-Smirnov One-Sample
Statistic,
Wolfgang Pelz and I. J. Good,
Journal of the Royal Statistical Society, Series B.
Vol. 38, No. 2 (1976), pp. 152-156
*/
final int JMAX = 20;
final double EPS = 1.0e-10;
final double RACN = Math.sqrt ((double) n);
final double z = RACN * x;
final double z2 = z * z;
final double z4 = z2 * z2;
final double z6 = z4 * z2;
final double C2PI = 2.506628274631001; // sqrt(2*Pi)
final double DPI2 = 1.2533141373155001; // sqrt(Pi/2)
final double PI2 = Math.PI * Math.PI;
final double PI4 = PI2 * PI2;
final double w = PI2 / (2.0 * z * z);
double ti, term, tom;
double sum;
int j;
term = 1;
j = 0;
sum = 0;
while (j <= JMAX && term > EPS * sum) {
ti = j + 0.5;
term = Math.exp (-ti * ti * w);
sum += term;
j++;
}
sum *= C2PI / z;
term = 1;
tom = 0;
j = 0;
while (j <= JMAX && Math.abs (term) > EPS * Math.abs (tom)) {
ti = j + 0.5;
term = (PI2 * ti * ti - z2) * Math.exp (-ti * ti * w);
tom += term;
j++;
}
sum += tom * DPI2 / (RACN * 3.0 * z4);
term = 1;
tom = 0;
j = 0;
while (j <= JMAX && Math.abs (term) > EPS * Math.abs (tom)) {
ti = j + 0.5;
term = 6 * z6 + 2 * z4 + PI2 * (2 * z4 - 5 * z2) * ti * ti +
PI4 * (1 - 2 * z2) * ti * ti * ti * ti;
term *= Math.exp (-ti * ti * w);
tom += term;
j++;
}
sum += tom * DPI2 / (n * 36.0 * z * z6);
term = 1;
tom = 0;
j = 1;
while (j <= JMAX && term > EPS * tom) {
ti = j;
term = PI2 * ti * ti * Math.exp (-ti * ti * w);
tom += term;
j++;
}
sum -= tom * DPI2 / (n * 18.0 * z * z2);
term = 1;
tom = 0;
j = 0;
while (j <= JMAX && Math.abs (term) > EPS * Math.abs (tom)) {
ti = j + 0.5;
ti = ti * ti;
term = -30 * z6 - 90 * z6 * z2 + PI2 * (135 * z4 - 96 * z6) * ti +
PI4 * (212 * z4 - 60 * z2) * ti * ti +
PI2 * PI4 * ti * ti * ti * (5 - 30 * z2);
term *= Math.exp (-ti * w);
tom += term;
j++;
}
sum += tom * DPI2 / (RACN * n * 3240.0 * z4 * z6);
term = 1;
tom = 0;
j = 1;
while (j <= JMAX && Math.abs (term) > EPS * Math.abs (tom)) {
ti = j * j;
term = (3 * PI2 * ti * z2 - PI4 * ti * ti) * Math.exp (-ti * w);
tom += term;
j++;
}
sum += tom * DPI2 / (RACN * n * 108.0 * z6);
return sum;
}
//========================================================================
private static void CalcFloorCeil (
int n, // sample size
double t, // = nx
double[] A, // A_i
double[] Atflo, // floor (A_i - t)
double[] Atcei // ceiling (A_i + t)
)
{
// Precompute A_i, floors, and ceilings for limits of sums in the
// Pomeranz algorithm
int i;
int ell = (int) t; // floor (t)
double z = t - ell; // t - floor (t)
double w = Math.ceil (t) - t;
if (z > 0.5) {
for (i = 2; i <= 2 * n + 2; i += 2)
Atflo[i] = i / 2 - 2 - ell;
for (i = 1; i <= 2 * n + 2; i += 2)
Atflo[i] = i / 2 - 1 - ell;
for (i = 2; i <= 2 * n + 2; i += 2)
Atcei[i] = i / 2 + ell;
for (i = 1; i <= 2 * n + 2; i += 2)
Atcei[i] = i / 2 + 1 + ell;
} else if (z > 0.0) {
for (i = 1; i <= 2 * n + 2; i++)
Atflo[i] = i / 2 - 1 - ell;
for (i = 2; i <= 2 * n + 2; i++)
Atcei[i] = i / 2 + ell;
Atcei[1] = 1 + ell;
} else { // z == 0
for (i = 2; i <= 2 * n + 2; i += 2)
Atflo[i] = i / 2 - 1 - ell;
for (i = 1; i <= 2 * n + 2; i += 2)
Atflo[i] = i / 2 - ell;
for (i = 2; i <= 2 * n + 2; i += 2)
Atcei[i] = i / 2 - 1 + ell;
for (i = 1; i <= 2 * n + 2; i += 2)
Atcei[i] = i / 2 + ell;
}
if (w < z)
z = w;
A[0] = A[1] = 0;
A[2] = z;
A[3] = 1 - A[2];
for (i = 4; i <= 2 * n + 1; i++)
A[i] = A[i - 2] + 1;
A[2 * n + 2] = n;
}
//========================================================================
private static double Pomeranz (int n, double x)
{
// The Pomeranz algorithm to compute the KS distribution
final double EPS = 1.0e-15;
final int ENO = 350;
final double RENO = Math.scalb (1.0, ENO); // for renormalization of V
int coreno; // counter: how many renormalizations
final double t = n * x;
double w, sum, minsum;
int i, j, k, s;
int r1, r2; // Indices i and i-1 for V[i][]
int jlow, jup, klow, kup, kup0;
double[] A = new double[2 * n + 3];
double[] Atflo = new double[2 * n + 3];
double[] Atcei = new double[2 * n + 3];
double[][] V = new double[2][n + 2];
double[][] H = new double[4][n + 2]; // = pow(w, j) / Factorial(j)
CalcFloorCeil (n, t, A, Atflo, Atcei);
for (j = 1; j <= n + 1; j++)
V[0][j] = 0;
for (j = 2; j <= n + 1; j++)
V[1][j] = 0;
V[1][1] = RENO;
coreno = 1;
// Precompute H[][] = (A[j] - A[j-1]^k / k!
H[0][0] = 1;
w = 2.0 * A[2] / n;
for (j = 1; j <= n + 1; j++)
H[0][j] = w * H[0][j - 1] / j;
H[1][0] = 1;
w = (1.0 - 2.0 * A[2]) / n;
for (j = 1; j <= n + 1; j++)
H[1][j] = w * H[1][j - 1] / j;
H[2][0] = 1;
w = A[2] / n;
for (j = 1; j <= n + 1; j++)
H[2][j] = w * H[2][j - 1] / j;
H[3][0] = 1;
for (j = 1; j <= n + 1; j++)
H[3][j] = 0;
r1 = 0;
r2 = 1;
for (i = 2; i <= 2 * n + 2; i++) {
jlow = (int) (2 + Atflo[i]);
if (jlow < 1)
jlow = 1;
jup = (int) (Atcei[i]);
if (jup > n + 1)
jup = n + 1;
klow = (int) (2 + Atflo[i - 1]);
if (klow < 1)
klow = 1;
kup0 = (int) (Atcei[i - 1]);
// Find to which case it corresponds
w = (A[i] - A[i - 1]) / n;
s = -1;
for (j = 0; j < 4; j++) {
if (Math.abs (w - H[j][1]) <= EPS) {
s = j;
break;
}
}
minsum = RENO;
r1 = (r1 + 1) & 1; // i - 1
r2 = (r2 + 1) & 1; // i
for (j = jlow; j <= jup; j++) {
kup = kup0;
if (kup > j)
kup = j;
sum = 0;
for (k = kup; k >= klow; k--)
sum += V[r1][k] * H[s][j - k];
V[r2][j] = sum;
if (sum < minsum)
minsum = sum;
}
if (minsum < 1.0e-280) {
// V is too small: renormalize to avoid underflow of probabilities
for (j = jlow; j <= jup; j++)
V[r2][j] *= RENO;
coreno++; // keep track of log of RENO
}
}
sum = V[r2][n + 1];
w = Num.lnFactorial (n) - coreno * ENO * Num.LN2 + Math.log (sum);
if (w >= 0.)
return 1.;
return Math.exp (w);
}
//========================================================================
/**
* Computes the distribution function
* u = P[Dn <= x] with
* parameter n, using the program described in.
* This method uses Pomeranz's recursion algorithm and the Durbin matrix algorithm for
* n <= 500, which returns at least 13 decimal digits of precision. It uses
* the Pelz-Good asymptotic expansion in the central part of
* the range for n > 500 and returns at least 7 decimal digits of precision
* everywhere for
* 500 < n <= 100000. For
* n > 100000, it returns
* at least 5 decimal digits of precision for all
* u > 10-16, and a
* few correct decimals when
* u <= 10-16.
* This method is much faster than method cdf of
* {@link KolmogorovSmirnovDist} for moderate or large n.
* Restriction: n >= 1.
*
*/
public static double cdf (int n, double x) {
double u = cdfConnu (n, x);
if (u >= 0.0)
return u;
final double w = n * x * x;
if (n <= NEXACT) {
if (w < 0.754693)
return DurbinMatrix (n, x);
if (w < 4.0)
return Pomeranz (n, x);
return 1.0 - barF (n, x);
}
if ((w * x * n <= 7.0) && (n <= NKOLMO))
return DurbinMatrix(n, x);
return Pelz (n, x);
}
/**
* Computes the complementary distribution
*
* P[Dn >= x] with parameter n,
* in a form that is more precise in the upper tail,
* using the program described in.
* It returns at least 10 decimal digits of precision everywhere for all
* n <= 500,
* at least 6 decimal digits of precision for
* 500 < n <= 200000,
* and a few correct decimal digits (1 to 5) for
* n > 200000.
* This method is much faster and more precise for x close to 1, than
* method barF of
* {@link KolmogorovSmirnovDist} for moderate or large n.
* Restriction: n >= 1.
*
*/
public static double barF (int n, double x) {
double v = barFConnu (n, x);
if (v >= 0.0)
return v;
final double w = n * x * x;
if (n <= NEXACT) {
if (w < 4.0)
return 1.0 - cdf (n, x);
else
return 2.0 * KolmogorovSmirnovPlusDist.KSPlusbarUpper(n, x);
}
if (w >= 2.65)
return 2.0 * KolmogorovSmirnovPlusDist.KSPlusbarUpper (n, x);
return 1.0 - cdf (n, x);
}
/**
* Computes the inverse
* x = F-1(u) of the
* distribution F(x) with parameter n.
*
*/
public static double inverseF (int n, double u) {
double Res = inverseConnue(n,u);
if (Res != -1.0)
return Res;
Function f = new Function (n,u);
return RootFinder.brentDekker (0.5/n, 1.0, f, 1e-5);
}
}
© 2015 - 2025 Weber Informatics LLC | Privacy Policy