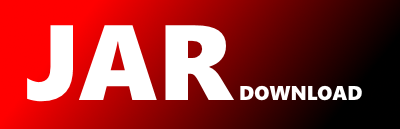
umontreal.iro.lecuyer.randvar.PoissonTIACGen Maven / Gradle / Ivy
Go to download
SSJ is a Java library for stochastic simulation, developed under the direction of Pierre L'Ecuyer,
in the Département d'Informatique et de Recherche Opérationnelle (DIRO), at the Université de Montréal.
It provides facilities for generating uniform and nonuniform random variates, computing different
measures related to probability distributions, performing goodness-of-fit tests, applying quasi-Monte
Carlo methods, collecting (elementary) statistics, and programming discrete-event simulations with both
events and processes.
The newest version!
Please wait ...