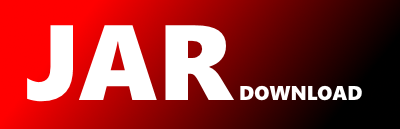
cc.kave.repackaged.jayes.factor.FactorFactory Maven / Gradle / Ivy
Go to download
Show more of this group Show more artifacts with this name
Show all versions of cc.kave.repackaged.jayes Show documentation
Show all versions of cc.kave.repackaged.jayes Show documentation
Repackaging of Jayes (Eclipse Code Recommenders) to make it available in Maven.
The newest version!
/*******************************************************************************
* Copyright (c) 2012 Michael Kutschke.
* All rights reserved. This program and the accompanying materials
* are made available under the terms of the Eclipse Public License v1.0
* which accompanies this distribution, and is available at
* http://www.eclipse.org/legal/epl-v10.html
*
* Contributors:
* Michael Kutschke - initial API and implementation
******************************************************************************/
package cc.kave.repackaged.jayes.factor;
import java.util.ArrayList;
import java.util.List;
import cc.kave.repackaged.jayes.BayesNet;
import cc.kave.repackaged.jayes.factor.arraywrapper.DoubleArrayWrapper;
import cc.kave.repackaged.jayes.factor.arraywrapper.FloatArrayWrapper;
import cc.kave.repackaged.jayes.factor.arraywrapper.IArrayWrapper;
import cc.kave.repackaged.jayes.internal.util.ArrayUtils;
import cc.kave.repackaged.jayes.util.MathUtils;
public class FactorFactory {
protected BayesNet net;
private boolean useLogScale = false;
private IArrayWrapper prototype = new DoubleArrayWrapper(0.0); //TODO is a length of 1 here still necessary?
/**
* sets the floating point precision to use.
*
* @param contentType
* possible values: double.class, Double.class, float.class, Float.class
*/
public void setFloatingPointType(Class> contentType) {
if (contentType == double.class || contentType == Double.class) {
prototype = new DoubleArrayWrapper(0.0);
} else if (contentType == float.class || contentType == Float.class) {
prototype = new FloatArrayWrapper(0.0f);
} else {
throw new IllegalArgumentException("wrong type, expected double, Double, float or Float, but got: "
+ contentType);
}
}
public void setReferenceNetwork(BayesNet net) {
this.net = net;
}
/**
* using logarithmic values for computation is a lot slower, but also a lot more numerically stable
*
* @param useLogScale use log scale
*/
public void setUseLogScale(boolean useLogScale) {
this.useLogScale = useLogScale;
}
/**
* creates a factor, the class of which is dependent on different criteria defined in the concrete subclasses. The
* default behavior is to return a DenseFactor.
*
* @param vars input vars
* @param multiplicationPartners multiplication partners
* @return the resulting factor
*/
public AbstractFactor create(List vars, List multiplicationPartners) {
final int[] dimensions = getDimensionSizes(vars);
AbstractFactor[] partners = multiplicationPartners.toArray(new AbstractFactor[0]);
if (SparseFactor.isSuitable(MathUtils.product(dimensions), partners)) {
SparseFactor f = new SparseFactor();
initializeFactor(vars, dimensions, f);
f.sparsify(partners);
return f;
} else {
DenseFactor f2 = new DenseFactor();
initializeFactor(vars, dimensions, f2);
return f2;
}
}
private void initializeFactor(List vars,
final int[] dimensions, AbstractFactor f) {
f.setValues(prototype.clone());
f.setDimensions(dimensions);
f.setDimensionIDs(ArrayUtils.toIntArray(vars));
if (useLogScale) {
f.setLogScale(true);
}
}
private int[] getDimensionSizes(List vars) {
final List dimensions = new ArrayList();
for (final Integer dim : vars) {
dimensions.add(net.getNode(dim).getOutcomeCount());
}
return ArrayUtils.toIntArray(dimensions);
}
// weird signature to allow overriding for testing mixed log scale computation
// (just because we can't configure it right now, we still want to support it)
protected boolean getUseLogScale(AbstractFactor f) {
return useLogScale;
}
public static FactorFactory defaultFactory() {
return new FactorFactory();
}
}
© 2015 - 2025 Weber Informatics LLC | Privacy Policy