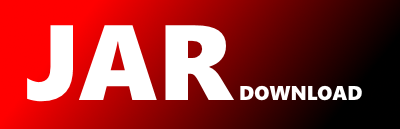
cc.kave.repackaged.jayes.inference.junctionTree.JunctionTreeAlgorithm Maven / Gradle / Ivy
Go to download
Show more of this group Show more artifacts with this name
Show all versions of cc.kave.repackaged.jayes Show documentation
Show all versions of cc.kave.repackaged.jayes Show documentation
Repackaging of Jayes (Eclipse Code Recommenders) to make it available in Maven.
The newest version!
/**
* Copyright (c) 2011 Michael Kutschke. All rights reserved. This program and the accompanying
* materials are made available under the terms of the Eclipse Public License v1.0 which accompanies
* this distribution, and is available at http://www.eclipse.org/legal/epl-v10.html Contributors:
* Michael Kutschke - initial API and implementation.
*/
package cc.kave.repackaged.jayes.inference.junctionTree;
import static cc.kave.repackaged.jayes.util.Pair.newPair;
import java.util.ArrayList;
import java.util.Arrays;
import java.util.Collections;
import java.util.HashMap;
import java.util.HashSet;
import java.util.IdentityHashMap;
import java.util.List;
import java.util.ListIterator;
import java.util.Map;
import java.util.Map.Entry;
import java.util.Set;
import cc.kave.repackaged.jayes.BayesNet;
import cc.kave.repackaged.jayes.BayesNode;
import cc.kave.repackaged.jayes.factor.AbstractFactor;
import cc.kave.repackaged.jayes.factor.arraywrapper.DoubleArrayWrapper;
import cc.kave.repackaged.jayes.factor.arraywrapper.IArrayWrapper;
import cc.kave.repackaged.jayes.inference.AbstractInferer;
import cc.kave.repackaged.jayes.internal.util.ArrayUtils;
import cc.kave.repackaged.jayes.util.Graph;
import cc.kave.repackaged.jayes.util.MathUtils;
import cc.kave.repackaged.jayes.util.NumericalInstabilityException;
import cc.kave.repackaged.jayes.util.Pair;
import cc.kave.repackaged.jayes.util.Graph.Edge;
import cc.kave.repackaged.jayes.util.sharing.CanonicalArrayWrapperManager;
import cc.kave.repackaged.jayes.util.sharing.CanonicalIntArrayManager;
import cc.kave.repackaged.jayes.util.triangulation.MinFillIn;
public class JunctionTreeAlgorithm extends AbstractInferer {
protected Map sepSets;
protected Graph junctionTree;
protected AbstractFactor[] nodePotentials;
// need IdentityHashmap here because an Edge and
// it's backward Edge are considered equal
// (which is also needed for simplicity)
protected IdentityHashMap preparedMultiplications;
// mapping from variables to clusters that contain them
protected int[][] concernedClusters;
protected AbstractFactor[] queryFactors;
protected int[][] preparedQueries;
protected boolean[] isBeliefValid;
protected List> initializations;
protected int[][] queryFactorReverseMapping;
// used for computing evidence collection skip
protected Set clustersHavingEvidence;
protected boolean[] isObserved;
protected double[] scratchpad;
protected JunctionTreeBuilder junctionTreeBuilder = JunctionTreeBuilder.forHeuristic(new MinFillIn());
public void setJunctionTreeBuilder(JunctionTreeBuilder bldr) {
this.junctionTreeBuilder = bldr;
}
@Override
public double[] getBeliefs(final BayesNode node) {
if (!beliefsValid) {
beliefsValid = true;
updateBeliefs();
}
final int nodeId = node.getId();
if (!isBeliefValid[nodeId]) {
isBeliefValid[nodeId] = true;
if (!evidence.containsKey(node)) {
validateBelief(nodeId);
} else {
Arrays.fill(beliefs[nodeId], 0);
beliefs[nodeId][node.getOutcomeIndex(evidence.get(node))] = 1;
}
}
return super.getBeliefs(node);
}
private void validateBelief(final int nodeId) {
final AbstractFactor f = queryFactors[nodeId];
// TODO change beliefs to ArrayWrappers
f.sumPrepared(new DoubleArrayWrapper(beliefs[nodeId]), preparedQueries[nodeId]);
if (f.isLogScale()) {
MathUtils.exp(beliefs[nodeId]);
}
try {
beliefs[nodeId] = MathUtils.normalize(beliefs[nodeId]);
} catch (final IllegalArgumentException exception) {
throw new NumericalInstabilityException("Numerical instability detected for evidence: " + evidence
+ " and node : " + nodeId
+ ", consider using logarithmic scale computation (configurable in FactorFactory)", exception);
}
}
@Override
protected void updateBeliefs() {
Arrays.fill(isBeliefValid, false);
doUpdateBeliefs();
}
private void doUpdateBeliefs() {
incorporateAllEvidence();
int propagationRoot = findPropagationRoot();
replayFactorInitializations();
collectEvidence(propagationRoot, skipCollection(propagationRoot));
distributeEvidence(propagationRoot, skipDistribution(propagationRoot));
}
private void replayFactorInitializations() {
for (final Pair init : initializations) {
init.getFirst().copyValues(init.getSecond());
}
}
private void incorporateAllEvidence() {
for (Pair init : initializations) {
init.getFirst().resetSelections();
}
clustersHavingEvidence.clear();
Arrays.fill(isObserved, false);
for (BayesNode n : evidence.keySet()) {
incorporateEvidence(n);
}
}
private void incorporateEvidence(final BayesNode node) {
int n = node.getId();
isObserved[n] = true;
// get evidence to all concerned factors (includes home cluster)
for (final Integer concernedCluster : concernedClusters[n]) {
nodePotentials[concernedCluster].select(n, node.getOutcomeIndex(evidence.get(node)));
clustersHavingEvidence.add(concernedCluster);
}
}
private int findPropagationRoot() {
int propagationRoot = 0;
for (BayesNode n : evidence.keySet()) {
propagationRoot = concernedClusters[n.getId()][0];
}
return propagationRoot;
}
/**
* checks which nodes need not be processed during collectEvidence (because of preprocessing). These are those nodes
* without evidence which are leaves or which only have non-evidence descendants
*
* @param root
* the node to start the check from
* @return a set of the nodes not needing a call of collectEvidence
*/
private Set skipCollection(final int root) {
final Set skipped = new HashSet(nodePotentials.length);
recursiveSkipCollection(root, new HashSet(nodePotentials.length), skipped);
return skipped;
}
private void recursiveSkipCollection(final int node, final Set visited, final Set skipped) {
visited.add(node);
boolean areAllDescendantsSkipped = true;
for (final Edge e : junctionTree.getIncidentEdges(node)) {
if (!visited.contains(e.getSecond())) {
recursiveSkipCollection(e.getSecond(), visited, skipped);
if (!skipped.contains(e.getSecond())) {
areAllDescendantsSkipped = false;
}
}
}
if (areAllDescendantsSkipped && !clustersHavingEvidence.contains(node)) {
skipped.add(node);
}
}
/**
* checks which nodes do not need to be visited during evidence distribution. These are exactly those nodes which
* are
*
* - not the query factor of a non-evidence variable
* - AND have no descendants that cannot be skipped
*
*
* @param distNode
* @return
*/
private Set skipDistribution(final int distNode) {
final Set skipped = new HashSet(nodePotentials.length);
recursiveSkipDistribution(distNode, new HashSet(nodePotentials.length), skipped);
return skipped;
}
private void recursiveSkipDistribution(final int node, final Set visited, final Set skipped) {
visited.add(node);
boolean areAllDescendantsSkipped = true;
for (final Edge e : junctionTree.getIncidentEdges(node)) {
if (!visited.contains(e.getSecond())) {
recursiveSkipDistribution(e.getSecond(), visited, skipped);
if (!skipped.contains(e.getSecond())) {
areAllDescendantsSkipped = false;
}
}
}
if (areAllDescendantsSkipped && !isQueryFactorOfUnobservedVariable(node)) {
skipped.add(node);
}
}
private boolean isQueryFactorOfUnobservedVariable(final int node) {
for (int i : queryFactorReverseMapping[node]) {
if (!isObserved[i]) {
return true;
}
}
return false;
}
private void collectEvidence(final int cluster, final Set marked) {
marked.add(cluster);
for (final Edge e : junctionTree.getIncidentEdges(cluster)) {
if (!marked.contains(e.getSecond())) {
collectEvidence(e.getSecond(), marked);
messagePass(e.getBackEdge());
}
}
}
private void distributeEvidence(final int cluster, final Set marked) {
marked.add(cluster);
for (final Edge e : junctionTree.getIncidentEdges(cluster)) {
if (!marked.contains(e.getSecond())) {
messagePass(e);
distributeEvidence(e.getSecond(), marked);
}
}
}
private void messagePass(final Edge sepSetEdge) {
final AbstractFactor sepSet = sepSets.get(sepSetEdge);
if (!needMessagePass(sepSet)) {
return;
}
final IArrayWrapper newSepValues = sepSet.getValues();
System.arraycopy(newSepValues.toDoubleArray(), 0, scratchpad, 0, newSepValues.length());
final int[] preparedOp = preparedMultiplications.get(sepSetEdge.getBackEdge());
nodePotentials[sepSetEdge.getFirst()].sumPrepared(newSepValues, preparedOp);
if (isOnlyFirstLogScale(sepSetEdge)) {
MathUtils.exp(newSepValues);
}
if (areBothEndsLogScale(sepSetEdge)) {
MathUtils.secureSubtract(newSepValues.toDoubleArray(), scratchpad, scratchpad);
} else {
MathUtils.secureDivide(newSepValues.toDoubleArray(), scratchpad, scratchpad);
}
if (isOnlySecondLogScale(sepSetEdge)) {
MathUtils.log(scratchpad);
}
// TODO scratchpad -> ArrayWrapper
nodePotentials[sepSetEdge.getSecond()].multiplyPrepared(new DoubleArrayWrapper(scratchpad),
preparedMultiplications.get(sepSetEdge));
}
/*
* we don't get additional information if all variables in the sepSet are
* observed, so skip message pass
*/
private boolean needMessagePass(final AbstractFactor sepSet) {
for (final int var : sepSet.getDimensionIDs()) {
if (!isObserved[var]) {
return true;
}
}
return false;
}
private boolean isOnlyFirstLogScale(final Edge edge) {
return nodePotentials[edge.getFirst()].isLogScale() && !nodePotentials[edge.getSecond()].isLogScale();
}
private boolean isOnlySecondLogScale(final Edge edge) {
return !nodePotentials[edge.getFirst()].isLogScale() && nodePotentials[edge.getSecond()].isLogScale();
}
@Override
public void setNetwork(final BayesNet net) {
super.setNetwork(net);
initializeFields(net.getNodes().size());
JunctionTree jtree = buildJunctionTree(net);
int[] homeClusters = computeHomeClusters(net, jtree.getClusters());
initializeClusterFactors(net, jtree.getClusters(), homeClusters);
initializeSepsetFactors(jtree.getSepSets());
determineConcernedClusters();
setQueryFactors();
initializePotentialValues();
multiplyCPTsIntoPotentials(net, homeClusters);
prepareMultiplications();
prepareScratch();
invokeInitialBeliefUpdate();
storePotentialValues();
}
@SuppressWarnings("unchecked")
private void determineConcernedClusters() {
concernedClusters = new int[queryFactors.length][];
List[] temp = new List[concernedClusters.length];
for (int i = 0; i < temp.length; i++) {
temp[i] = new ArrayList();
}
for (int i = 0; i < nodePotentials.length; i++) {
int[] dimensionIDs = nodePotentials[i].getDimensionIDs();
for (final int var : dimensionIDs) {
temp[var].add(i);
}
}
for (int i = 0; i < temp.length; i++) {
concernedClusters[i] = ArrayUtils.toIntArray(temp[i]);
}
}
private void initializeFields(int numNodes) {
isBeliefValid = new boolean[beliefs.length];
Arrays.fill(isBeliefValid, false);
queryFactors = new AbstractFactor[numNodes];
preparedQueries = new int[numNodes][];
sepSets = new HashMap();
preparedMultiplications = new IdentityHashMap();
initializations = new ArrayList>();
clustersHavingEvidence = new HashSet();
isObserved = new boolean[numNodes];
}
private JunctionTree buildJunctionTree(BayesNet net) {
final JunctionTree jtree = junctionTreeBuilder.buildJunctionTree(net);
this.junctionTree = jtree.getGraph();
return jtree;
}
private int[] computeHomeClusters(BayesNet net, final List> clusters) {
int[] homeClusters = new int[net.getNodes().size()];
for (final BayesNode node : net.getNodes()) {
final List nodeAndParents = getNodeAndParentIds(node);
for (final ListIterator> clusterIt = clusters.listIterator(); clusterIt.hasNext();) {
if (clusterIt.next().containsAll(nodeAndParents)) {
homeClusters[node.getId()] = clusterIt.nextIndex() - 1;
break;
}
}
}
return homeClusters;
}
private List getNodeAndParentIds(final BayesNode n) {
final List nodeAndParents = new ArrayList(n.getParents().size() + 1);
nodeAndParents.add(n.getId());
for (final BayesNode p : n.getParents()) {
nodeAndParents.add(p.getId());
}
return nodeAndParents;
}
private void initializeClusterFactors(BayesNet net, final List> clusters, int[] homeClusters) {
nodePotentials = new AbstractFactor[clusters.size()];
Map> multiplicationPartners = findMultiplicationPartners(net, homeClusters);
for (final ListIterator> cliqueIt = clusters.listIterator(); cliqueIt.hasNext();) {
final List cluster = cliqueIt.next();
int current = cliqueIt.nextIndex() - 1;
List multiplicationPartnerList = multiplicationPartners.get(current);
final AbstractFactor cliqueFactor = factory.create(cluster,
multiplicationPartnerList == null ? Collections.emptyList()
: multiplicationPartnerList);
nodePotentials[current] = cliqueFactor;
}
}
private Map> findMultiplicationPartners(BayesNet net, int[] homeClusters) {
Map> potentialMap = new HashMap>();
for (final BayesNode node : net.getNodes()) {
final Integer nodeHome = homeClusters[node.getId()];
if (!potentialMap.containsKey(nodeHome)) {
potentialMap.put(nodeHome, new ArrayList());
}
potentialMap.get(nodeHome).add(node.getFactor());
}
return potentialMap;
}
private void initializeSepsetFactors(final List>> sepSets) {
for (final Pair> sep : sepSets) {
this.sepSets.put(sep.getFirst(), factory.create(sep.getSecond(), Collections.emptyList()));
}
}
private void setQueryFactors() {
for (int i = 0; i < queryFactors.length; i++) {
for (final Integer f : concernedClusters[i]) {
final boolean isFirstOrSmallerTable = queryFactors[i] == null
|| queryFactors[i].getValues().length() > nodePotentials[f].getValues().length();
if (isFirstOrSmallerTable) {
queryFactors[i] = nodePotentials[f];
}
}
}
queryFactorReverseMapping = new int[nodePotentials.length][];
for (int i = 0; i < nodePotentials.length; i++) {
List queryVars = new ArrayList();
for (int var : nodePotentials[i].getDimensionIDs()) {
if (queryFactors[var] == nodePotentials[i]) {
queryVars.add(var);
}
}
queryFactorReverseMapping[i] = ArrayUtils.toIntArray(queryVars);
}
}
private void prepareMultiplications() {
// compress by combining equal prepared statements, thus saving memory
final CanonicalIntArrayManager flyWeight = new CanonicalIntArrayManager();
prepareSepsetMultiplications(flyWeight);
prepareQueries(flyWeight);
}
private void prepareSepsetMultiplications(final CanonicalIntArrayManager flyWeight) {
for (int node = 0; node < nodePotentials.length; node++) {
for (final Edge e : junctionTree.getIncidentEdges(node)) {
final int[] preparedMultiplication = nodePotentials[e.getSecond()]
.prepareMultiplication(sepSets.get(e));
preparedMultiplications.put(e, flyWeight.getInstance(preparedMultiplication));
}
}
}
private void prepareQueries(final CanonicalIntArrayManager flyWeight) {
for (int i = 0; i < queryFactors.length; i++) {
final AbstractFactor beliefFactor = factory.create(Arrays.asList(i),
Collections.emptyList());
final int[] preparedQuery = queryFactors[i].prepareMultiplication(beliefFactor);
preparedQueries[i] = flyWeight.getInstance(preparedQuery);
}
}
private void prepareScratch() {
int maxSize = 0;
for (AbstractFactor sepSet : sepSets.values()) {
maxSize = Math.max(maxSize, sepSet.getValues().length());
}
scratchpad = new double[maxSize];
}
private void invokeInitialBeliefUpdate() {
collectEvidence(0, new HashSet());
distributeEvidence(0, new HashSet());
}
private void initializePotentialValues() {
final double ONE_LOG = 0.0;
final double ONE = 1.0;
for (final AbstractFactor f : nodePotentials) {
f.fill(f.isLogScale() ? ONE_LOG : ONE);
}
for (final Entry sepSet : sepSets.entrySet()) {
if (!areBothEndsLogScale(sepSet.getKey())) {
// if one part is log-scale, we transform to non-log-scale
sepSet.getValue().fill(ONE);
} else {
sepSet.getValue().fill(ONE_LOG);
}
}
}
private void multiplyCPTsIntoPotentials(BayesNet net, int[] homeClusters) {
for (final BayesNode node : net.getNodes()) {
final AbstractFactor nodeHome = nodePotentials[homeClusters[node.getId()]];
if (nodeHome.isLogScale()) {
nodeHome.multiplyCompatibleToLog(node.getFactor());
} else {
nodeHome.multiplyCompatible(node.getFactor());
}
}
}
private boolean areBothEndsLogScale(final Edge edge) {
return nodePotentials[edge.getFirst()].isLogScale() && nodePotentials[edge.getSecond()].isLogScale();
}
private void storePotentialValues() {
CanonicalArrayWrapperManager flyweight = new CanonicalArrayWrapperManager();
for (final AbstractFactor pot : nodePotentials) {
initializations.add(newPair(pot, flyweight.getInstance(pot.getValues().clone())));
}
for (final AbstractFactor sep : sepSets.values()) {
initializations.add(newPair(sep, flyweight.getInstance(sep.getValues().clone())));
}
}
}
© 2015 - 2025 Weber Informatics LLC | Privacy Policy