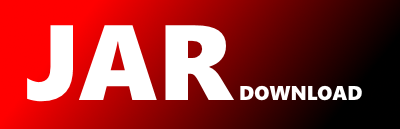
cc.mallet.classify.MaxEntOptimizableByLabelLikelihood Maven / Gradle / Ivy
Go to download
Show more of this group Show more artifacts with this name
Show all versions of mallet Show documentation
Show all versions of mallet Show documentation
MALLET is a Java-based package for statistical natural language processing,
document classification, clustering, topic modeling, information extraction,
and other machine learning applications to text.
The newest version!
package cc.mallet.classify;
import java.io.Serializable;
import java.util.Arrays;
import java.util.Iterator;
import java.util.logging.Logger;
import cc.mallet.optimize.LimitedMemoryBFGS;
import cc.mallet.optimize.Optimizable;
import cc.mallet.types.Alphabet;
import cc.mallet.types.FeatureSelection;
import cc.mallet.types.FeatureVector;
import cc.mallet.types.Instance;
import cc.mallet.types.InstanceList;
import cc.mallet.types.LabelAlphabet;
import cc.mallet.types.Labeling;
import cc.mallet.types.MatrixOps;
import cc.mallet.util.MalletLogger;
import cc.mallet.util.MalletProgressMessageLogger;
import cc.mallet.util.Maths;
public class MaxEntOptimizableByLabelLikelihood implements Optimizable.ByGradientValue {
private static Logger logger =
MalletLogger.getLogger(MaxEntOptimizableByLabelLikelihood.class.getName());
private static Logger progressLogger =
MalletProgressMessageLogger.getLogger(MaxEntOptimizableByLabelLikelihood.class.getName()+"-pl");
// xxx Why does TestMaximizable fail when this variance is very small?
static final double DEFAULT_GAUSSIAN_PRIOR_VARIANCE = 1;
static final double DEFAULT_HYPERBOLIC_PRIOR_SLOPE = 0.2;
static final double DEFAULT_HYPERBOLIC_PRIOR_SHARPNESS = 10.0;
static final Class DEFAULT_MAXIMIZER_CLASS = LimitedMemoryBFGS.class;
boolean usingHyperbolicPrior = false;
boolean usingGaussianPrior = true;
double gaussianPriorVariance = DEFAULT_GAUSSIAN_PRIOR_VARIANCE;
double hyperbolicPriorSlope = DEFAULT_HYPERBOLIC_PRIOR_SLOPE;
double hyperbolicPriorSharpness = DEFAULT_HYPERBOLIC_PRIOR_SHARPNESS;
Class maximizerClass = DEFAULT_MAXIMIZER_CLASS;
double[] parameters, constraints, cachedGradient;
MaxEnt theClassifier;
InstanceList trainingList;
// The expectations are (temporarily) stored in the cachedGradient
double cachedValue;
boolean cachedValueStale;
boolean cachedGradientStale;
int numLabels;
int numFeatures;
int defaultFeatureIndex; // just for clarity
FeatureSelection featureSelection;
FeatureSelection[] perLabelFeatureSelection;
int numGetValueCalls = 0;
int numGetValueGradientCalls = 0;
public MaxEntOptimizableByLabelLikelihood() {
}
public MaxEntOptimizableByLabelLikelihood (InstanceList trainingSet, MaxEnt initialClassifier)
{
this.trainingList = trainingSet;
Alphabet fd = trainingSet.getDataAlphabet();
LabelAlphabet ld = (LabelAlphabet) trainingSet.getTargetAlphabet();
// Don't fd.stopGrowth, because someone might want to do feature induction
ld.stopGrowth();
// Add one feature for the "default feature".
this.numLabels = ld.size();
this.numFeatures = fd.size() + 1;
this.defaultFeatureIndex = numFeatures-1;
this.parameters = new double [numLabels * numFeatures];
this.constraints = new double [numLabels * numFeatures];
this.cachedGradient = new double [numLabels * numFeatures];
Arrays.fill (parameters, 0.0);
Arrays.fill (constraints, 0.0);
Arrays.fill (cachedGradient, 0.0);
this.featureSelection = trainingSet.getFeatureSelection();
this.perLabelFeatureSelection = trainingSet.getPerLabelFeatureSelection();
// Add the default feature index to the selection
if (featureSelection != null)
featureSelection.add (defaultFeatureIndex);
if (perLabelFeatureSelection != null)
for (int i = 0; i < perLabelFeatureSelection.length; i++)
perLabelFeatureSelection[i].add (defaultFeatureIndex);
// xxx Later change this to allow both to be set, but select which one to use by a boolean flag?
assert (featureSelection == null || perLabelFeatureSelection == null);
if (initialClassifier != null) {
this.theClassifier = initialClassifier;
this.parameters = theClassifier.parameters;
this.featureSelection = theClassifier.featureSelection;
this.perLabelFeatureSelection = theClassifier.perClassFeatureSelection;
this.defaultFeatureIndex = theClassifier.defaultFeatureIndex;
assert (initialClassifier.getInstancePipe() == trainingSet.getPipe());
}
else if (this.theClassifier == null) {
this.theClassifier = new MaxEnt (trainingSet.getPipe(), parameters, featureSelection, perLabelFeatureSelection);
}
cachedValueStale = true;
cachedGradientStale = true;
// Initialize the constraints
logger.fine("Number of instances in training list = " + trainingList.size());
for (Instance inst : trainingList) {
double instanceWeight = trainingList.getInstanceWeight(inst);
Labeling labeling = inst.getLabeling ();
if (labeling == null)
continue;
//logger.fine ("Instance "+ii+" labeling="+labeling);
FeatureVector fv = (FeatureVector) inst.getData ();
Alphabet fdict = fv.getAlphabet();
assert (fv.getAlphabet() == fd);
int li = labeling.getBestIndex();
MatrixOps.rowPlusEquals (constraints, numFeatures, li, fv, instanceWeight);
// For the default feature, whose weight is 1.0
assert(!Double.isNaN(instanceWeight)) : "instanceWeight is NaN";
assert(!Double.isNaN(li)) : "bestIndex is NaN";
boolean hasNaN = false;
for (int i = 0; i < fv.numLocations(); i++) {
if(Double.isNaN(fv.valueAtLocation(i))) {
logger.info("NaN for feature " + fdict.lookupObject(fv.indexAtLocation(i)).toString());
hasNaN = true;
}
}
if (hasNaN)
logger.info("NaN in instance: " + inst.getName());
constraints[li*numFeatures + defaultFeatureIndex] += 1.0 * instanceWeight;
}
//TestMaximizable.testValueAndGradientCurrentParameters (this);
}
public MaxEnt getClassifier () { return theClassifier; }
public double getParameter (int index) {
return parameters[index];
}
public void setParameter (int index, double v) {
cachedValueStale = true;
cachedGradientStale = true;
parameters[index] = v;
}
public int getNumParameters() {
return parameters.length;
}
public void getParameters (double[] buff) {
if (buff == null || buff.length != parameters.length)
buff = new double [parameters.length];
System.arraycopy (parameters, 0, buff, 0, parameters.length);
}
public void setParameters (double [] buff) {
assert (buff != null);
cachedValueStale = true;
cachedGradientStale = true;
if (buff.length != parameters.length)
parameters = new double[buff.length];
System.arraycopy (buff, 0, parameters, 0, buff.length);
}
// log probability of the training labels
public double getValue ()
{
if (cachedValueStale) {
numGetValueCalls++;
cachedValue = 0;
// We'll store the expectation values in "cachedGradient" for now
cachedGradientStale = true;
MatrixOps.setAll (cachedGradient, 0.0);
// Incorporate likelihood of data
double[] scores = new double[trainingList.getTargetAlphabet().size()];
double value = 0.0;
Iterator iter = trainingList.iterator();
int ii=0;
while (iter.hasNext()) {
ii++;
Instance instance = iter.next();
double instanceWeight = trainingList.getInstanceWeight(instance);
Labeling labeling = instance.getLabeling ();
if (labeling == null)
continue;
//System.out.println("L Now "+inputAlphabet.size()+" regular features.");
this.theClassifier.getClassificationScores (instance, scores);
FeatureVector fv = (FeatureVector) instance.getData ();
int li = labeling.getBestIndex();
value = - (instanceWeight * Math.log (scores[li]));
if(Double.isNaN(value)) {
logger.fine ("MaxEntTrainer: Instance " + instance.getName() +
"has NaN value. log(scores)= " + Math.log(scores[li]) +
" scores = " + scores[li] +
" has instance weight = " + instanceWeight);
}
if (Double.isInfinite(value)) {
logger.warning ("Instance "+instance.getSource() + " has infinite value; skipping value and gradient");
cachedValue -= value;
cachedValueStale = false;
return -value;
// continue;
}
cachedValue += value;
for (int si = 0; si < scores.length; si++) {
if (scores[si] == 0) continue;
assert (!Double.isInfinite(scores[si]));
MatrixOps.rowPlusEquals (cachedGradient, numFeatures,
si, fv, -instanceWeight * scores[si]);
cachedGradient[numFeatures*si + defaultFeatureIndex] += (-instanceWeight * scores[si]);
}
}
//logger.info ("-Expectations:"); cachedGradient.print();
// Incorporate prior on parameters
double prior = 0;
if (usingHyperbolicPrior) {
for (int li = 0; li < numLabels; li++)
for (int fi = 0; fi < numFeatures; fi++)
prior += (hyperbolicPriorSlope / hyperbolicPriorSharpness
* Math.log (Maths.cosh (hyperbolicPriorSharpness * parameters[li *numFeatures + fi])));
}
else if (usingGaussianPrior) {
for (int li = 0; li < numLabels; li++)
for (int fi = 0; fi < numFeatures; fi++) {
double param = parameters[li*numFeatures + fi];
prior += param * param / (2 * gaussianPriorVariance);
}
}
double oValue = cachedValue;
cachedValue += prior;
cachedValue *= -1.0; // MAXIMIZE, NOT MINIMIZE
cachedValueStale = false;
progressLogger.info ("Value (labelProb="+oValue+" prior="+prior+") loglikelihood = "+cachedValue);
}
return cachedValue;
}
public void getValueGradient (double [] buffer) {
// Gradient is (constraint - expectation - parameters/gaussianPriorVariance)
if (cachedGradientStale) {
numGetValueGradientCalls++;
if (cachedValueStale)
// This will fill in the cachedGradient with the "-expectation"
getValue ();
MatrixOps.plusEquals (cachedGradient, constraints);
// Incorporate prior on parameters
if (usingHyperbolicPrior) {
throw new UnsupportedOperationException ("Hyperbolic prior not yet implemented.");
}
else if (usingGaussianPrior) {
MatrixOps.plusEquals (cachedGradient, parameters,
-1.0 / gaussianPriorVariance);
}
// A parameter may be set to -infinity by an external user.
// We set gradient to 0 because the parameter's value can
// never change anyway and it will mess up future calculations
// on the matrix, such as norm().
MatrixOps.substitute (cachedGradient, Double.NEGATIVE_INFINITY, 0.0);
// Set to zero all the gradient dimensions that are not among the selected features
if (perLabelFeatureSelection == null) {
for (int labelIndex = 0; labelIndex < numLabels; labelIndex++)
MatrixOps.rowSetAll (cachedGradient, numFeatures,
labelIndex, 0.0, featureSelection, false);
} else {
for (int labelIndex = 0; labelIndex < numLabels; labelIndex++)
MatrixOps.rowSetAll (cachedGradient, numFeatures,
labelIndex, 0.0,
perLabelFeatureSelection[labelIndex], false);
}
cachedGradientStale = false;
}
assert (buffer != null && buffer.length == parameters.length);
System.arraycopy (cachedGradient, 0, buffer, 0, cachedGradient.length);
//System.out.println ("MaxEntTrainer gradient infinity norm = "+MatrixOps.infinityNorm(cachedGradient));
}
// XXX Should these really be public? Why?
/** Counts how many times this trainer has computed the gradient of the
* log probability of training labels. */
public int getValueGradientCalls() {return numGetValueGradientCalls;}
/** Counts how many times this trainer has computed the
* log probability of training labels. */
public int getValueCalls() {return numGetValueCalls;}
// public int getIterations() {return maximizerByGradient.getIterations();}
public MaxEntOptimizableByLabelLikelihood useGaussianPrior () {
this.usingGaussianPrior = true;
this.usingHyperbolicPrior = false;
return this;
}
public MaxEntOptimizableByLabelLikelihood useHyperbolicPrior () {
this.usingGaussianPrior = false;
this.usingHyperbolicPrior = true;
return this;
}
/**
* In some cases a prior term is implemented in the optimizer,
* (eg orthant-wise L-BFGS), so we occasionally want to only
* calculate the log likelihood.
*/
public MaxEntOptimizableByLabelLikelihood useNoPrior () {
this.usingGaussianPrior = false;
this.usingHyperbolicPrior = false;
return this;
}
/**
* Sets a parameter to prevent overtraining. A smaller variance for the prior
* means that feature weights are expected to hover closer to 0, so extra
* evidence is required to set a higher weight.
* @return This trainer
*/
public MaxEntOptimizableByLabelLikelihood setGaussianPriorVariance (double gaussianPriorVariance)
{
this.usingGaussianPrior = true;
this.usingHyperbolicPrior = false;
this.gaussianPriorVariance = gaussianPriorVariance;
return this;
}
public MaxEntOptimizableByLabelLikelihood setHyperbolicPriorSlope (double hyperbolicPriorSlope)
{
this.usingGaussianPrior = false;
this.usingHyperbolicPrior = true;
this.hyperbolicPriorSlope = hyperbolicPriorSlope;
return this;
}
public MaxEntOptimizableByLabelLikelihood setHyperbolicPriorSharpness (double hyperbolicPriorSharpness)
{
this.usingGaussianPrior = false;
this.usingHyperbolicPrior = true;
this.hyperbolicPriorSharpness = hyperbolicPriorSharpness;
return this;
}
}
© 2015 - 2025 Weber Informatics LLC | Privacy Policy