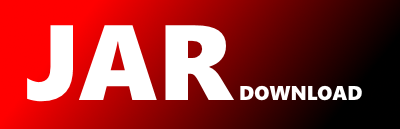
cc.mallet.grmm.learning.PseudolikelihoodACRFTrainer Maven / Gradle / Ivy
Go to download
MALLET is a Java-based package for statistical natural language processing,
document classification, clustering, topic modeling, information extraction,
and other machine learning applications to text.
The newest version!
Please wait ...