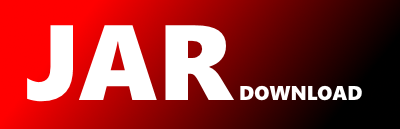
ch.ethz.globis.phtree.v11.PhQueryKnnMbbPP Maven / Gradle / Ivy
Go to download
Show more of this group Show more artifacts with this name
Show all versions of phtree Show documentation
Show all versions of phtree Show documentation
The PH-Tree is a multi-dimensional index
/*
* Copyright 2011-2016 ETH Zurich. All Rights Reserved.
*
* This software is the proprietary information of ETH Zurich.
* Use is subject to license terms.
*/
package ch.ethz.globis.phtree.v11;
import java.util.ArrayList;
import java.util.Arrays;
import java.util.NoSuchElementException;
import ch.ethz.globis.phtree.PhDistance;
import ch.ethz.globis.phtree.PhEntry;
import ch.ethz.globis.phtree.PhEntryDist;
import ch.ethz.globis.phtree.PhFilterDistance;
import ch.ethz.globis.phtree.PhTree.PhExtent;
import ch.ethz.globis.phtree.PhTree.PhKnnQuery;
/**
* kNN query implementation that uses preprocessors and distance functions.
*
* The algorithm works as follows:
*
* First we drill down in the tree to find an entry that is 'close' to
* desired center of the kNN query. A 'close' entry is one that is in the same node
* where the center would be, or in one of its sub-nodes. Note that we do not use
* the center-point itself in case it exists in the tree. The result of the first step is
* a guess at the initial search distance (this would be 0 if we used the center itself).
*
* We then use a combination of rectangle query (center +/- initDistance) and distance-query.
* The query traverses only nodes and values that lie in the query rectangle and that satisfy the
* distance requirement (circular distance when using euclidean space).
*
* While iterating through the query result, we regularly sort the returned entries
* to see which distance would suffice to return 'k' result. If the new distance is smaller,
* we adjust the query rectangle and the distance function before continuing the
* query. As a result, when the query returns no more entries, we are guaranteed to
* have all closest neighbours.
*
* The only thing that can go wrong is that we may get less than 'k' neighbours if the
* initial distance was too small. In that case we multiply the initial distance by 10
* and run the algorithm again. Not that multiplying the distance by 10 means a 10^k fold
* increase in the search volume.
*
*
* WARNING:
* The query rectangle is calculated using the PhDistance.toMBB() method.
* The implementation of this method may not work with non-euclidean spaces!
*
* @param value type
*/
public class PhQueryKnnMbbPP implements PhKnnQuery {
private final int dims;
private int nMin;
private PhTree11 pht;
private PhDistance distance;
private final ArrayList> entries = new ArrayList<>();
private int resultSize = 0;
private int currentPos = -1;
private final long[] mbbMin;
private final long[] mbbMax;
private final PhIteratorNoGC iter;
private final PhFilterDistance checker;
public PhQueryKnnMbbPP(PhTree11 pht) {
this.dims = pht.getDim();
this.mbbMin = new long[dims];
this.mbbMax = new long[dims];
this.pht = pht;
this.checker = new PhFilterDistance();
this.iter = new PhIteratorNoGC<>(pht, checker);
}
@Override
public long[] nextKey() {
return nextEntryReuse().getKey();
}
@Override
public T nextValue() {
return nextEntryReuse().getValue();
}
@Override
public PhEntryDist nextEntry() {
return new PhEntryDist<>(nextEntryReuse());
}
@Override
public PhEntryDist nextEntryReuse() {
if (currentPos >= resultSize) {
throw new NoSuchElementException();
}
return entries.get(currentPos++);
}
@Override
public boolean hasNext() {
return currentPos < resultSize;
}
@Override
public T next() {
return nextValue();
}
@Override
public PhKnnQuery reset(int nMin, PhDistance dist, long... center) {
this.distance = dist == null ? this.distance : dist;
this.nMin = nMin;
clearEntries();
if (nMin > 0) {
nearestNeighbourBinarySearch(center, nMin);
}
currentPos = 0;
return this;
}
private void findKnnCandidate(long[] center, long[] ret) {
findKnnCandidate(center, pht.getRoot(), ret);
}
private long[] findKnnCandidate(long[] key, Node node, long[] ret) {
Object v = node.doIfMatching(key, true, null, null, null, pht);
if (v == null) {
//Okay, there is no perfect match:
//just perform a query on the current node and return the first value that we find.
return returnAnyValue(ret, key, node);
}
if (v instanceof Node) {
return findKnnCandidate(key, (Node) v, ret);
}
//so we have a perfect match!
//But we should return it only if nMin=1, otherwise our search area is too small.
if (nMin == 1) {
//Never return closest key if we look for nMin>1 keys!
//now return the key, even if it may not be an exact match (we don't check)
System.arraycopy(key, 0, ret, 0, key.length);
return ret;
}
//Okay just perform a query on the current node and return the first value that we find.
return returnAnyValue(ret, key, node);
}
private long[] returnAnyValue(long[] ret, long[] key, Node node) {
//First, get correct prefix.
long mask = (-1L) << (node.getPostLen()+1);
for (int i = 0; i < dims; i++) {
ret[i] = key[i] & mask;
}
NodeIteratorFullNoGC ni = new NodeIteratorFullNoGC<>(dims, ret);
//This allows writing the result directly into 'ret'
PhEntry result = new PhEntry<>(ret, null);
ni.init(node, null);
while (ni.increment(result)) {
if (result.hasNodeInternal()) {
//traverse sub node
ni.init((Node) result.getNodeInternal(), null);
} else {
//Never return closest key if we look for nMin>1 keys!
if (nMin > 1 && Arrays.equals(key, result.getKey())) {
//Never return a perfect match if we look for nMin>1 keys!
//otherwise the distance is too small.
//This check should be cheap and will not be executed more than once anyway.
continue;
}
return ret;
}
}
throw new IllegalStateException();
}
/**
* This approach applies binary search to queries.
* It start with a query that covers the whole tree. Then whenever it finds an entry (the first)
* it discards the query and starts a smaller one with half the distance to the search-point.
* This effectively reduces the volume by 2^k.
* Once a query returns no result, it uses the previous query to traverse all results
* and find the nearest result.
* As an intermediate step, it may INCREASE the query size until a non-empty query appears.
* Then it could decrease again, like a true binary search.
*
* When looking for nMin > 1, one could search for queries with at least nMin results...
*
* @param val
* @param nMin
*/
private void nearestNeighbourBinarySearch(long[] val, int nMin) {
//special case with minDist = 0
if (nMin == 1 && pht.contains(val)) {
addEntry(new PhEntry(val, pht.get(val)), val);
return;
}
//special case with size() <= nMin
if (pht.size() <= nMin) {
PhExtent itEx = pht.queryExtent();
while (itEx.hasNext()) {
PhEntry e = itEx.nextEntryReuse();
addEntry(e, val);
}
sortEntries();
return;
}
//estimate initial distance
long[] cand = new long[dims];
findKnnCandidate(val, cand);
double currentDist = distance.dist(val, cand);
while (!findNeighbours(currentDist, nMin, val)) {
currentDist *= 10;
}
}
private final boolean findNeighbours(double maxDist, int nMin, long[] val) {
//Epsilon for calculating the distance depends on DIM, the magnitude of the values and
//the precision of the Double mantissa.
final double EPS = dims * maxDist / (double)(1L << 51);//2^(53-2));
final int CONSOLIDATION_INTERVAL = 10;
clearEntries();
checker.set(val, distance, maxDist);
distance.toMBB(maxDist, val, mbbMin, mbbMax);
iter.reset(mbbMin, mbbMax);
// Get nMin results
while (iter.hasNext() && resultSize < nMin) {
PhEntry en = iter.nextEntryReuse();
addEntry(en, val);
}
sortEntries();
if (resultSize < nMin) {
//too small, we need a bigger range
return false;
}
if (!iter.hasNext()) {
//perfect fit!
return true;
}
//get distance of farthest entry and continue query with this new distance
maxDist = entries.get(nMin-1).dist();
checker.set(val, distance, maxDist);
distance.toMBB(maxDist, val, mbbMin, mbbMax);
iter.adjustMinMax();
// we continue the query but reduce the range maximum range
int cnt = 0;
while (iter.hasNext()) {
PhEntry e = iter.nextEntryReuse();
addEntry(e, val);
cnt++;
if (cnt % CONSOLIDATION_INTERVAL == 0) {
maxDist = consolidate(nMin, EPS, maxDist);
//update query-dist
checker.set(val, distance, maxDist);
distance.toMBB(maxDist, val, mbbMin, mbbMax);
iter.adjustMinMax();
}
}
// no more elements in tree
consolidate(nMin, EPS, maxDist);
return true;
}
private double consolidate(int nMin, double eps, double max) {
sortEntries();
double maxDnew = entries.get(nMin-1).dist();
if (maxDnew < max+eps) { //TODO epsilon?
max = maxDnew;
for (int i2 = nMin; i2 < resultSize; i2++) {
//purge
if (entries.get(i2).dist() + eps > max) {
resultSize = i2;
break;
}
}
}
return max;
}
private void addEntry(PhEntry e, long[] center) {
double dist = distance.dist(center, e.getKey());
if (resultSize < entries.size()) {
entries.get(resultSize).set(e, dist);
} else {
PhEntryDist de = new PhEntryDist<>(e, dist);
entries.add(de);
}
resultSize++;
}
private void clearEntries() {
resultSize = 0;
for (int i = 0; i < entries.size(); i++) {
entries.get(i).clear();
}
}
private void sortEntries() {
entries.sort(PhEntryDist.COMP);
}
}
© 2015 - 2024 Weber Informatics LLC | Privacy Policy