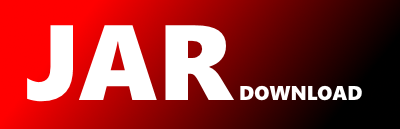
com.actelion.research.calc.MatrixTests Maven / Gradle / Ivy
Go to download
Show more of this group Show more artifacts with this name
Show all versions of openchemlib Show documentation
Show all versions of openchemlib Show documentation
Open Source Chemistry Library
/*
* Copyright (c) 1997 - 2016
* Actelion Pharmaceuticals Ltd.
* Gewerbestrasse 16
* CH-4123 Allschwil, Switzerland
*
* All rights reserved.
*
* Redistribution and use in source and binary forms, with or without
* modification, are permitted provided that the following conditions are met:
*
* 1. Redistributions of source code must retain the above copyright notice, this
* list of conditions and the following disclaimer.
* 2. Redistributions in binary form must reproduce the above copyright notice,
* this list of conditions and the following disclaimer in the documentation
* and/or other materials provided with the distribution.
* 3. Neither the name of the the copyright holder nor the
* names of its contributors may be used to endorse or promote products
* derived from this software without specific prior written permission.
*
* THIS SOFTWARE IS PROVIDED BY THE COPYRIGHT HOLDERS AND CONTRIBUTORS "AS IS" AND
* ANY EXPRESS OR IMPLIED WARRANTIES, INCLUDING, BUT NOT LIMITED TO, THE IMPLIED
* WARRANTIES OF MERCHANTABILITY AND FITNESS FOR A PARTICULAR PURPOSE ARE
* DISCLAIMED. IN NO EVENT SHALL THE COPYRIGHT OWNER OR CONTRIBUTORS BE LIABLE FOR
* ANY DIRECT, INDIRECT, INCIDENTAL, SPECIAL, EXEMPLARY, OR CONSEQUENTIAL DAMAGES
* (INCLUDING, BUT NOT LIMITED TO, PROCUREMENT OF SUBSTITUTE GOODS OR SERVICES;
* LOSS OF USE, DATA, OR PROFITS; OR BUSINESS INTERRUPTION) HOWEVER CAUSED AND
* ON ANY THEORY OF LIABILITY, WHETHER IN CONTRACT, STRICT LIABILITY, OR TORT
* (INCLUDING NEGLIGENCE OR OTHERWISE) ARISING IN ANY WAY OUT OF THE USE OF THIS
* SOFTWARE, EVEN IF ADVISED OF THE POSSIBILITY OF SUCH DAMAGE.
*/
package com.actelion.research.calc;
import com.actelion.research.calc.regression.linear.pls.SimPLS;
import com.actelion.research.util.IO;
import com.actelion.research.util.datamodel.ModelXY;
import java.util.Date;
import java.util.Random;
/**
* MatrixData
* @author Modest von Korff
* @version 1.0
* Sep 13, 2013 MvK Start implementation
*/
public class MatrixTests {
/**
* Creates a multivariate test dataset
* The regression factor factor is the col number, starting with 1.
* @param rows
* @param cols
* @return
*/
public static ModelXY getMultivariate(int rows, int cols){
ModelXY modelXY = new ModelXY();
Matrix X = new Matrix(rows, cols);
Matrix Y = new Matrix(rows, 1);
double max = 10;
Random rnd = new Random();
for (int i = 0; i < cols; i++) {
for (int j = 0; j < rows; j++) {
double v = rnd.nextDouble() * max;
X.set(j,i,v);
}
}
for (int i = 0; i < rows; i++) {
double y = 0;
for (int j = 0; j < cols; j++) {
double v = X.get(i, j);
y += v*(j+1);
}
Y.set(i, 0, y);
}
modelXY.X = X;
modelXY.Y = Y;
return modelXY;
}
public static Matrix test00() {
double [][] A = {{1.001},
{1.002},
{1.003},
{1.004}};
Matrix ma = new Matrix(A);
return ma;
}
public static Matrix test01() {
double [][] A = {{1.004},
{1.003},
{1.002},
{1.001}};
Matrix ma = new Matrix(A);
return ma;
}
public static Matrix test02() {
double [][] A = {{1,3,4},
{2,3,4},
{3,3,2},
{4,3,1}};
Matrix ma = new Matrix(A);
return ma;
}
public static Matrix test03() {
double [][] A = {{1,0,0,0},
{1,0,0,0},
{0,1,0,0},
{0,1,0,0},
{0,0,1,0},
{0,0,1,0},
{0,0,0,1},
{0,0,0,1}};
Matrix ma = new Matrix(A);
return ma;
}
public static Matrix test04() {
double [][] A = {{1,1,1,1},
{1,1,1,1},
{2,20,2,2},
{2,20,2,2},
{3,30,3,3},
{3,30,3,3},
{4,40,40,4},
{4,40,40,4}};
Matrix ma = new Matrix(A);
return ma;
}
public static Matrix test05() {
double [][] A = {{1,1,1,1},
{1,2,1,1},
{1,3,1,1},
{1,4,1,1},
{0,5,1,1},
{0,6,1,1},
{0,7,1,1},
{0,8,1,1}};
Matrix ma = new Matrix(A);
return ma;
}
public static Matrix test06() {
double [][] A = {{1,0},
{1,0},
{1,0},
{1,0},
{0,1},
{0,1},
{0,1},
{0,1}};
Matrix ma = new Matrix(A);
return ma;
}
public static Matrix test07() {
double [][] A = {{1,1,0,0},
{1,1,0,0},
{1,1,0,0},
{1,1,0,0},
{0,0,1,1},
{0,0,1,1},
{0,0,1,1},
{0,0,1,1}};
Matrix ma = new Matrix(A);
return ma;
}
public static Matrix test08() {
double [][] A = {{1,1,1,0},
{1,0,0,0},
{1,1,0,0},
{1,1,0,0},
{0,1,1,1},
{0,0,1,0},
{0,0,1,1},
{0,0,1,1}};
Matrix ma = new Matrix(A);
return ma;
}
public static Matrix testMatrix02() {
double [][] A = {{16, 2, 3, 13},
{ 5, 11, 10, 8},
{ 9, 7, 6, 12},
{ 4, 14, 15, 1}};
Matrix ma = new Matrix(A);
return ma;
}
/**
* Validation data for PLS from Abdi, PLS, Encyclopedia of Social Sciences
* Reasearch Methods (2003).
* @return Matrix
*/
public static Matrix testMatrix_YWine() {
double [][] A = {{ 14, 7, 8},
{ 10, 7, 6},
{ 8, 5, 5},
{ 2, 4, 7},
{ 6, 2, 4}};
Matrix ma = new Matrix(A);
return ma;
}
public static Matrix testMatrix_XWine() {
double [][] A = {{ 7, 7, 13, 7},
{ 4, 3, 14, 7},
{ 10, 5, 12, 5},
{ 16, 7, 11, 3},
{ 13, 3, 10, 3}};
Matrix ma = new Matrix(A);
return ma;
}
public static Matrix testMatrixHenrion01() {
double [][] A = {{ 4, 0},
{ 0, 8},
{ 4, 4},
{ 2, 0},
{ 0, 8}};
Matrix ma = new Matrix(A);
return ma;
}
/**
* http://www.itl.nist.gov/div898/strd/lls/data/Longley.shtml
* Y Matrix
* @return Y matrix
*/
public static Matrix testLonglyY() {
double [][] A = {{ 60323 },
{ 61122 },
{ 60171 },
{ 61187 },
{ 63221 },
{ 63639 },
{ 64989 },
{ 63761 },
{ 66019 },
{ 67857 },
{ 68169 },
{ 66513 },
{ 68655 },
{ 69564 },
{ 69331 },
{ 70551 }};
Matrix ma = new Matrix(A);
return ma;
}
/**
* http://itl.nist.gov/div898/strd/lls/data/LINKS/DATA/Longley.dat
* @return
*/
public static Matrix testLonglyX() {
double[][] A = {
{83, 234289, 2356, 1590, 107608, 1947},
{88.5, 259426, 2325, 1456, 108632, 1948},
{88.2, 258054, 3682, 1616, 109773, 1949},
{89.5, 284599, 3351, 1650, 110929, 1950},
{96.2, 328975, 2099, 3099, 112075, 1951},
{98.1, 346999, 1932, 3594, 113270, 1952},
{99, 365385, 1870, 3547, 115094, 1953},
{100, 363112, 3578, 3350, 116219, 1954},
{101.2, 397469, 2904, 3048, 117388, 1955},
{104.6, 419180, 2822, 2857, 118734, 1956},
{108.4, 442769, 2936, 2798, 120445, 1957},
{110.8, 444546, 4681, 2637, 121950, 1958},
{112.6, 482704, 3813, 2552, 123366, 1959},
{114.2, 502601, 3931, 2514, 125368, 1960},
{115.7, 518173, 4806, 2572, 127852, 1961},
{116.9, 554894, 4007, 2827, 130081, 1962},
};
Matrix ma = new Matrix(A);
return ma;
}
public static Matrix testDescriptor01X() {
double[][] A = {
{0, 0, 0, 1, 1, 1},
{0, 0, 0, 1, 1, 1},
{0, 0, 0, 1, 1, 1},
{0, 0, 0, 1, 1, 1},
{0, 0, 0, 1, 1, 1},
{0, 0, 0, 1, 1, 1},
{0, 0, 0, 1, 1, 1},
{0, 0, 0, 1, 1, 1},
{0, 0, 0, 1, 1, 1},
{0, 0, 0, 1, 1, 1},
{1, 1, 1, 0, 0, 0},
{1, 1, 1, 0, 0, 0},
{1, 1, 1, 0, 0, 0},
{1, 1, 1, 0, 0, 0},
{1, 1, 1, 0, 0, 0},
{1, 1, 1, 0, 0, 0},
{1, 1, 1, 0, 0, 0},
{1, 1, 1, 0, 0, 0},
{1, 1, 1, 0, 0, 0},
{1, 1, 1, 0, 0, 0},
{1, 1, 1, 0, 0, 0},
};
Matrix ma = new Matrix(A);
return ma;
}
public static Matrix testDescriptor01Y() {
double[][] A = {
{1,0},
{1,0},
{1,0},
{1,0},
{1,0},
{1,0},
{1,0},
{1,0},
{1,0},
{1,0},
{0,1},
{0,1},
{0,1},
{0,1},
{0,1},
{0,1},
{0,1},
{0,1},
{0,1},
{0,1},
{0,1},
};
Matrix ma = new Matrix(A);
return ma;
}
public static Matrix testSimple1Y() {
double [][] A = {{55},{56},{57},{58},{59},{60},{61},{62}};
Matrix ma = new Matrix(A);
return ma;
}
public static Matrix testSimple1X(int cols) {
double [][] a = {{55},
{56},
{57},
{58},
{59},
{60},
{61},
{62}};
Matrix Xrnd = Matrix.getRND(a.length, cols);
for (int i = 0; i < a.length; i++) {
Xrnd.set(i,0, a[i][0]);
}
return Xrnd;
}
public static Matrix testSimple2X(int cols) {
double [][] a = {{55,0},
{56,0},
{57,0},
{58,0},
{0,59},
{0,60},
{0,61},
{0,62}};
Matrix Xrnd = Matrix.getRND(a.length, cols);
for (int i = 0; i < a.length; i++) {
Xrnd.set(i,0, a[i][0]);
Xrnd.set(i,1, a[i][1]);
}
return Xrnd;
}
/**
* Checks for the correctness of the Eigenvector and Eigenvalues calculation.
* The test relies on the X V = V E equation (Henrion^2 (1995),p219).
* X is the symmetric original matrix. V is the diagonal matrix of the
* eigenvalues and E is the matrix of the corresponding eigenvectors.
* @return true if the check is ok.
*/
public static boolean checkForEigenvaluesAndEigenvectors() {
boolean bCheckOK = true;
Matrix A = testMatrix02();
Matrix AtA = A.multiply(true,false,A);
Matrix d = new Matrix(1,1);
Matrix e = new Matrix(1,1);
Matrix EV = new Matrix(AtA.getArray());
Matrix.getEigenvector(EV, EV.getColDim(), d, e);
Matrix D = d.diagonalize();
Matrix C = EV.multiply(false,false,D);
Matrix F = AtA.multiply(false,false,EV);
bCheckOK = C.equal(F, Matrix.TINY);
// System.out.println(C);
// System.out.println(F);
return bCheckOK;
}
static protected Matrix pls(Matrix X, Matrix Y,
String sPatternHeaderX,
int iNumPrincipalComponents,
boolean bLogarithm,
String sFileDataSummaryOut) {
Matrix R = null;
if(bLogarithm)
X = X.log();
// System.out.println(X.toString(4));
// Perform PLS
SimPLS pls = new SimPLS();
Matrix Xc = X.getCenteredMatrix();
Matrix Yc = Y.getCenteredMatrix();
String sSummary = "Xc(standardized):\r\n" + Xc + "\r\n\r\n";
sSummary += "Yc:\r\n" + Yc + "\r\n\r\n";
IO.write(sFileDataSummaryOut, sSummary, true);
pls.simPlsSave(Xc,Yc,iNumPrincipalComponents);
Matrix P = pls.getP();
R = pls.getR();
Matrix U = pls.getU();
Matrix V = pls.getV();
Matrix Q = pls.getQ();
Matrix T = pls.getT();
// Result matrices to summary file
sSummary = "Matrices from the PLS decomposition of the Training data.\r\n\r\n";
sSummary += "P:\r\n" + P + "\r\n\r\n";
sSummary += "R:\r\n" + R + "\r\n\r\n";
sSummary += "U:\r\n" + U + "\r\n\r\n";
sSummary += "V:\r\n" + V + "\r\n\r\n";
sSummary += "Q:\r\n" + Q + "\r\n\r\n";
sSummary += "T:\r\n" + T + "\r\n\r\n";
IO.write(sFileDataSummaryOut, sSummary, true);
return R;
}
@SuppressWarnings("unused")
public static void testMain01() {
int repeat = 10;
int rowsA = 1000;
int colsA = 1000;
int colsB = 100;
int n = 10;
Matrix X = MatrixFunctions.getRandomMatrix(rowsA,colsA);
Matrix Y = MatrixFunctions.getRandomMatrix(colsA,colsB);
Matrix Eleft = new Matrix(X);
Matrix Eright = new Matrix();
Matrix D = new Matrix();
Date dateStart = new Date();
Matrix.getEigenvector(Eleft, n, D, Eright);
Date dateEnd = new Date();
long delta = dateEnd.getTime() - dateStart.getTime();
System.out.println("Time: " + delta);
// System.out.println("D: " + D.toString());
// Date dateStart = new Date();
// Matrix C = null;
// for (int ii = 0; ii < repeat; ii++) {
// C = X.multiply(false, false, Y);
// }
// Date dateEnd = new Date();
// long delta = dateEnd.getTime() - dateStart.getTime();
// System.out.println("Time: " + delta);
// System.out.println("size: " + (C.getColDim() * C.getRowDim()));
//
// dateStart = new Date();
// for (int ii = 0; ii < repeat; ii++) {
// C = X.multiplyBig(false, false, Y);
// }
//
// dateEnd = new Date();
// delta = dateEnd.getTime() - dateStart.getTime();
// System.out.println("Time: " + delta);
// System.out.println("size: " + (C.getColDim() * C.getRowDim()));
}
public static void testMainHenrion() {
Matrix X = testMatrixHenrion01();
Matrix Xc = X.getCenteredMatrix();
System.out.println(Xc);
Matrix Xstand = X.getStandardDeviationCols();
System.out.println(Xstand);
Matrix Xs = X.getStandardized();
System.out.println(Xs);
// checkForEigenvaluesAndEigenvectors();
}
}
© 2015 - 2025 Weber Informatics LLC | Privacy Policy