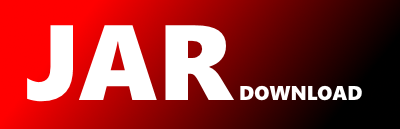
smile.clustering.BBDTree Maven / Gradle / Ivy
Go to download
Show more of this group Show more artifacts with this name
Show all versions of openchemlib Show documentation
Show all versions of openchemlib Show documentation
Open Source Chemistry Library
/*******************************************************************************
* Copyright (c) 2010 Haifeng Li
*
* Licensed under the Apache License, Version 2.0 (the "License");
* you may not use this file except in compliance with the License.
* You may obtain a copy of the License at
*
* http://www.apache.org/licenses/LICENSE-2.0
*
* Unless required by applicable law or agreed to in writing, software
* distributed under the License is distributed on an "AS IS" BASIS,
* WITHOUT WARRANTIES OR CONDITIONS OF ANY KIND, either express or implied.
* See the License for the specific language governing permissions and
* limitations under the License.
*******************************************************************************/
package smile.clustering;
import smile.math.Math;
import java.util.Arrays;
/**
* Balanced Box-Decomposition Tree. BBD tree is a specialized k-d tree that
* vastly speeds up an iteration of k-means. This is used internally by KMeans
* and batch SOM., and will most likely not need to be used directly.
*
* The structure works as follows:
*
* - All data data are placed into a tree where we choose child nodes by
* partitioning all data data along a plane parallel to the axis.
*
- We maintain for each node, the bounding box of all data data stored
* at that node.
*
- To do a k-means iteration, we need to assign data to clusters and
* calculate the sum and the number of data assigned to each cluster.
* For each node in the tree, we can rule out some cluster centroids as
* being too far away from every single point in that bounding box.
* Once only one cluster is left, all data in the node can be assigned
* to that cluster in batch.
*
*
* References
*
* - Tapas Kanungo, David M. Mount, Nathan S. Netanyahu, Christine D. Piatko, Ruth Silverman, and Angela Y. Wu. An Efficient k-Means Clustering Algorithm: Analysis and Implementation. IEEE TRANS. PAMI, 2002.
*
*
* @see KMeans
* @see smile.vq.SOM
*
* @author Haifeng Li
*/
public class BBDTree {
class Node {
/**
* The number of data stored in this node.
*/
int count;
/**
* The smallest point index stored in this node.
*/
int index;
/**
* The center/mean of bounding box.
*/
double[] center;
/**
* The half side-lengths of bounding box.
*/
double[] radius;
/**
* The sum of the data stored in this node.
*/
double[] sum;
/**
* The min cost for putting all data in this node in 1 cluster
*/
double cost;
/**
* The child node of lower half box.
*/
Node lower;
/**
* The child node of upper half box.
*/
Node upper;
/**
* Constructor.
* @param d the dimension of vector space.
*/
Node(int d) {
center = new double[d];
radius = new double[d];
sum = new double[d];
}
}
/**
* Root node.
*/
private Node root;
/**
* The index of data objects.
*/
private int[] index;
/**
* Constructs a tree out of the given n data data living in R^d.
*/
public BBDTree(double[][] data) {
int n = data.length;
index = new int[n];
for (int i = 0; i < n; i++) {
index[i] = i;
}
// Build the tree
root = buildNode(data, 0, n);
}
/**
* Build a k-d tree from the given set of data.
*/
private Node buildNode(double[][] data, int begin, int end) {
int d = data[0].length;
// Allocate the node
Node node = new Node(d);
// Fill in basic info
node.count = end - begin;
node.index = begin;
// Calculate the bounding box
double[] lowerBound = new double[d];
double[] upperBound = new double[d];
for (int i = 0; i < d; i++) {
lowerBound[i] = data[index[begin]][i];
upperBound[i] = data[index[begin]][i];
}
for (int i = begin + 1; i < end; i++) {
for (int j = 0; j < d; j++) {
double c = data[index[i]][j];
if (lowerBound[j] > c) {
lowerBound[j] = c;
}
if (upperBound[j] < c) {
upperBound[j] = c;
}
}
}
// Calculate bounding box stats
double maxRadius = -1;
int splitIndex = -1;
for (int i = 0; i < d; i++) {
node.center[i] = (lowerBound[i] + upperBound[i]) / 2;
node.radius[i] = (upperBound[i] - lowerBound[i]) / 2;
if (node.radius[i] > maxRadius) {
maxRadius = node.radius[i];
splitIndex = i;
}
}
// If the max spread is 0, make this a leaf node
if (maxRadius < 1E-10) {
node.lower = node.upper = null;
System.arraycopy(data[index[begin]], 0, node.sum, 0, d);
if (end > begin + 1) {
int len = end - begin;
for (int i = 0; i < d; i++) {
node.sum[i] *= len;
}
}
node.cost = 0;
return node;
}
// Partition the data around the midpoint in this dimension. The
// partitioning is done in-place by iterating from left-to-right and
// right-to-left in the same way that partioning is done in quicksort.
double splitCutoff = node.center[splitIndex];
int i1 = begin, i2 = end - 1, size = 0;
while (i1 <= i2) {
boolean i1Good = (data[index[i1]][splitIndex] < splitCutoff);
boolean i2Good = (data[index[i2]][splitIndex] >= splitCutoff);
if (!i1Good && !i2Good) {
int temp = index[i1];
index[i1] = index[i2];
index[i2] = temp;
i1Good = i2Good = true;
}
if (i1Good) {
i1++;
size++;
}
if (i2Good) {
i2--;
}
}
// Create the child nodes
node.lower = buildNode(data, begin, begin + size);
node.upper = buildNode(data, begin + size, end);
// Calculate the new sum and opt cost
for (int i = 0; i < d; i++) {
node.sum[i] = node.lower.sum[i] + node.upper.sum[i];
}
double[] mean = new double[d];
for (int i = 0; i < d; i++) {
mean[i] = node.sum[i] / node.count;
}
node.cost = getNodeCost(node.lower, mean) + getNodeCost(node.upper, mean);
return node;
}
/**
* Returns the total contribution of all data in the given kd-tree node,
* assuming they are all assigned to a mean at the given location.
*
* sum_{x \in node} ||x - mean||^2.
*
* If c denotes the mean of mass of the data in this node and n denotes
* the number of data in it, then this quantity is given by
*
* n * ||c - mean||^2 + sum_{x \in node} ||x - c||^2
*
* The sum is precomputed for each node as cost. This formula follows
* from expanding both sides as dot products.
*/
private double getNodeCost(Node node, double[] center) {
int d = center.length;
double scatter = 0.0;
for (int i = 0; i < d; i++) {
double x = (node.sum[i] / node.count) - center[i];
scatter += x * x;
}
return node.cost + node.count * scatter;
}
/**
* Given k cluster centroids, this method assigns data to nearest centroids.
* The return value is the distortion to the centroids. The parameter sums
* will hold the sum of data for each cluster. The parameter counts hold
* the number of data of each cluster. If membership is
* not null, it should be an array of size n that will be filled with the
* index of the cluster [0 - k) that each data point is assigned to.
*/
public double clustering(double[][] centroids, double[][] sums, int[] counts, int[] membership) {
int k = centroids.length;
Arrays.fill(counts, 0);
int[] candidates = new int[k];
for (int i = 0; i < k; i++) {
candidates[i] = i;
Arrays.fill(sums[i], 0.0);
}
return filter(root, centroids, candidates, k, sums, counts, membership);
}
/**
* This determines which clusters all data that are rooted node will be
* assigned to, and updates sums, counts and membership (if not null)
* accordingly. Candidates maintains the set of cluster indices which
* could possibly be the closest clusters for data in this subtree.
*/
private double filter(Node node, double[][] centroids, int[] candidates, int k, double[][] sums, int[] counts, int[] membership) {
int d = centroids[0].length;
// Determine which mean the node mean is closest to
double minDist = Math.squaredDistance(node.center, centroids[candidates[0]]);
int closest = candidates[0];
for (int i = 1; i < k; i++) {
double dist = Math.squaredDistance(node.center, centroids[candidates[i]]);
if (dist < minDist) {
minDist = dist;
closest = candidates[i];
}
}
// If this is a non-leaf node, recurse if necessary
if (node.lower != null) {
// Build the new list of candidates
int[] newCandidates = new int[k];
int newk = 0;
for (int i = 0; i < k; i++) {
if (!prune(node.center, node.radius, centroids, closest, candidates[i])) {
newCandidates[newk++] = candidates[i];
}
}
// Recurse if there's at least two
if (newk > 1) {
double result = filter(node.lower, centroids, newCandidates, newk, sums, counts, membership) + filter(node.upper, centroids, newCandidates, newk, sums, counts, membership);
return result;
}
}
// Assigns all data within this node to a single mean
for (int i = 0; i < d; i++) {
sums[closest][i] += node.sum[i];
}
counts[closest] += node.count;
if (membership != null) {
int last = node.index + node.count;
for (int i = node.index; i < last; i++) {
membership[index[i]] = closest;
}
}
return getNodeCost(node, centroids[closest]);
}
/**
* Determines whether every point in the box is closer to centroids[bestIndex] than to
* centroids[testIndex].
*
* If x is a point, c_0 = centroids[bestIndex], c = centroids[testIndex], then:
* (x-c).(x-c) < (x-c_0).(x-c_0)
* <=> (c-c_0).(c-c_0) < 2(x-c_0).(c-c_0)
*
* The right-hand side is maximized for a vertex of the box where for each
* dimension, we choose the low or high value based on the sign of x-c_0 in
* that dimension.
*/
private boolean prune(double[] center, double[] radius, double[][] centroids, int bestIndex, int testIndex) {
if (bestIndex == testIndex) {
return false;
}
int d = centroids[0].length;
double[] best = centroids[bestIndex];
double[] test = centroids[testIndex];
double lhs = 0.0, rhs = 0.0;
for (int i = 0; i < d; i++) {
double diff = test[i] - best[i];
lhs += diff * diff;
if (diff > 0) {
rhs += (center[i] + radius[i] - best[i]) * diff;
} else {
rhs += (center[i] - radius[i] - best[i]) * diff;
}
}
return (lhs >= 2 * rhs);
}
}
© 2015 - 2025 Weber Informatics LLC | Privacy Policy