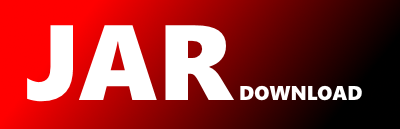
com.actelion.research.calc.regression.ModelError Maven / Gradle / Ivy
Go to download
Show more of this group Show more artifacts with this name
Show all versions of openchemlib Show documentation
Show all versions of openchemlib Show documentation
Open Source Chemistry Library
/*
* Copyright (c) 1997 - 2016
* Actelion Pharmaceuticals Ltd.
* Gewerbestrasse 16
* CH-4123 Allschwil, Switzerland
*
* All rights reserved.
*
* Redistribution and use in source and binary forms, with or without
* modification, are permitted provided that the following conditions are met:
*
* 1. Redistributions of source code must retain the above copyright notice, this
* list of conditions and the following disclaimer.
* 2. Redistributions in binary form must reproduce the above copyright notice,
* this list of conditions and the following disclaimer in the documentation
* and/or other materials provided with the distribution.
* 3. Neither the name of the the copyright holder nor the
* names of its contributors may be used to endorse or promote products
* derived from this software without specific prior written permission.
*
* THIS SOFTWARE IS PROVIDED BY THE COPYRIGHT HOLDERS AND CONTRIBUTORS "AS IS" AND
* ANY EXPRESS OR IMPLIED WARRANTIES, INCLUDING, BUT NOT LIMITED TO, THE IMPLIED
* WARRANTIES OF MERCHANTABILITY AND FITNESS FOR A PARTICULAR PURPOSE ARE
* DISCLAIMED. IN NO EVENT SHALL THE COPYRIGHT OWNER OR CONTRIBUTORS BE LIABLE FOR
* ANY DIRECT, INDIRECT, INCIDENTAL, SPECIAL, EXEMPLARY, OR CONSEQUENTIAL DAMAGES
* (INCLUDING, BUT NOT LIMITED TO, PROCUREMENT OF SUBSTITUTE GOODS OR SERVICES;
* LOSS OF USE, DATA, OR PROFITS; OR BUSINESS INTERRUPTION) HOWEVER CAUSED AND
* ON ANY THEORY OF LIABILITY, WHETHER IN CONTRACT, STRICT LIABILITY, OR TORT
* (INCLUDING NEGLIGENCE OR OTHERWISE) ARISING IN ANY WAY OUT OF THE USE OF THIS
* SOFTWARE, EVEN IF ADVISED OF THE POSSIBILITY OF SUCH DAMAGE.
*/
package com.actelion.research.calc.regression;
import com.actelion.research.calc.Matrix;
import com.actelion.research.calc.MatrixFunctions;
import com.actelion.research.calc.classification.PrecisionAndRecall;
import com.actelion.research.util.Formatter;
import com.actelion.research.util.datamodel.DoubleArray;
import java.util.*;
/**
* ModelError
*
* This class is a data model for the error. It is not the error of the model.
*
* @author Modest von Korff
* Aug 14, 2015 MvK Start implementation
*/
public class ModelError {
// Average from the sum of |errors|
public double error;
public double errorMedian;
public double errorRelative;
public double errorRelativeMedian;
public double errorRelativeWeighted;
public double errSumSquared;
public double errMax;
public double errMin;
public double corrSquared;
public double corrSquaredSpearman;
// Classification
public boolean classification;
public PrecisionAndRecall precisionAndRecall;
public boolean failed;
public int nNotFiniteRelError;
/**
*
*/
public ModelError() {
failed = false;
}
public void setFailed() {
this.failed = true;
}
public boolean isFailed() {
return failed;
}
/* (non-Javadoc)
* @see java.lang.Object#toString()
*/
@Override
public String toString() {
StringBuilder sb = new StringBuilder();
if(failed) {
sb.append("ModelError [error=failed]");
} else {
sb.append("ModelError [error=");
sb.append(Formatter.format3(error));
sb.append(", errRelativeMedian=");
sb.append(Formatter.format3(errorRelativeMedian));
if(nNotFiniteRelError!=0){
sb.append(", NotFiniteRelError=");
sb.append(nNotFiniteRelError);
}
sb.append(", errMax=");
sb.append(Formatter.format3(errMax));
sb.append(", errMin=");
sb.append(Formatter.format3(errMin));
sb.append(", corrSquared=");
sb.append(Formatter.format3(corrSquared));
if(precisionAndRecall!=null){
sb.append(", Cohen's kappa=");
sb.append(Formatter.format3(precisionAndRecall.calculateCohensKappa()));
}
sb.append("]");
}
return sb.toString();
}
/**
* Calculates the absolute and the relative error.
* @param Y
* @param YHat
* @return
*/
public static ModelError calculateError(Matrix Y, Matrix YHat){
ModelError modelError = new ModelError();
modelError.errMax = 0;
modelError.errMin = Integer.MAX_VALUE;
DoubleArray daError = new DoubleArray(Y.rows()*YHat.cols());
double sumSquared = 0;
for (int i = 0; i < YHat.cols(); i++) {
for (int j = 0; j < YHat.rows(); j++) {
double e = Math.abs(Y.get(j, i) - YHat.get(j, i));
sumSquared += e*e;
modelError.errMax = Math.max(modelError.errMax, e);
modelError.errMin = Math.min(modelError.errMin, e);
modelError.error += e;
daError.add(e);
}
}
modelError.error = modelError.error / (YHat.rows()*YHat.cols());
modelError.errSumSquared = sumSquared;
modelError.errorMedian = daError.median();
DoubleArray daErrorRelative = new DoubleArray(YHat.rows()*YHat.cols());
modelError.nNotFiniteRelError=0;
for (int i = 0; i < YHat.cols(); i++) {
for (int j = 0; j < YHat.rows(); j++) {
double y = Y.get(j, i);
double yHat = YHat.get(j, i);
double er = getRelativeError(y, yHat);
if(Double.isFinite(er)){
daErrorRelative.add(er);
}else {
modelError.nNotFiniteRelError++;
}
}
}
if(daErrorRelative.size()>0) {
modelError.errorRelative = daErrorRelative.avr();
modelError.errorRelativeMedian = daErrorRelative.median();
}
//
// Weighted error
//
DoubleArray daErrorRelativeWeighted = new DoubleArray(YHat.rows()*YHat.cols());
for (int i = 0; i < YHat.cols(); i++) {
for (int j = 0; j < YHat.rows(); j++) {
double y = Y.get(j, i);
double yHat = YHat.get(j, i);
double w = Math.log10(10+y);
if(Math.abs(y) > Matrix.TINY04) {
double er = Math.abs((yHat - y) / y) * (1.0/w);
if(Double.isFinite(er)) {
daErrorRelativeWeighted.add(er);
}
} else {
double er = Math.abs((yHat - y) / Matrix.TINY04) * (1.0/w);
if(Double.isFinite(er)) {
daErrorRelativeWeighted.add(er);
}
}
}
}
modelError.errorRelativeWeighted = daErrorRelativeWeighted.avr();
double corr = 0;
double corrSpearman = 0;
try {
corr = MatrixFunctions.getCorrPearson(YHat, Y);
corrSpearman = MatrixFunctions.getCorrSpearman(YHat, Y);
} catch (Exception e) {
e.printStackTrace();
System.err.println("YHat");
System.err.println(YHat.toString());
System.err.println("Y");
System.err.println(Y.toString());
}
if(!Double.isFinite(corr)){
corr=0;
}
if(!Double.isFinite(corrSpearman)){
corrSpearman=0;
}
modelError.corrSquared = corr*corr;
modelError.corrSquaredSpearman = corrSpearman*corrSpearman;
return modelError;
}
public static double getRelativeError(double y, double yHat){
double er = 0;
if(Math.abs(y) > Matrix.TINY04) {
er = Math.abs((yHat - y) / y);
} else {
er = Math.abs((yHat - y) / Matrix.TINY04);
}
return er;
}
public static ModelError calculateError(Matrix Y, Matrix YHat, double threshold, boolean above){
ModelError me = calculateError(Y, YHat);
me.precisionAndRecall = new PrecisionAndRecall();
for (int i = 0; i < YHat.cols(); i++) {
for (int j = 0; j < YHat.rows(); j++) {
double y = Y.get(j, i);
double yHat = YHat.get(j, i);
if(above) {
if(y>=threshold && yHat>=threshold) {
me.precisionAndRecall.truePositive++;
} else if(y < threshold && yHat < threshold) {
me.precisionAndRecall.trueNegative++;
} else if (yHat>=threshold){
me.precisionAndRecall.falsePositive++;
} else if (yHat threshold && yHat > threshold) {
me.precisionAndRecall.trueNegative++;
} else if (yHat<=threshold){
me.precisionAndRecall.falsePositive++;
} else if (yHat>threshold){
me.precisionAndRecall.falseNegative++;
}
}
}
}
me.classification = true;
return me;
}
public static List getError(List liME){
List li = new ArrayList();
for (ModelError modelError : liME) {
li.add(modelError.error);
}
return li;
}
public static ModelError getErrorAverage(List liME){
ModelError modelErrorAvr = new ModelError();
for (ModelError modelError : liME) {
modelErrorAvr.errMax += modelError.errMax;
modelErrorAvr.errMin += modelError.errMin;
modelErrorAvr.error += modelError.error;
modelErrorAvr.corrSquared += modelError.corrSquared;
}
int n = liME.size();
modelErrorAvr.errMax /= n;
modelErrorAvr.errMin /= n;
modelErrorAvr.error /= n;
modelErrorAvr.corrSquared /= n;
return modelErrorAvr;
}
public static Comparator getComparatorError(){
return new Comparator() {
@Override
public int compare(ModelError o1, ModelError o2) {
int cmp = 0;
if(o1.error > o2.error){
cmp=1;
}else if(o1.error < o2.error){
cmp=-1;
}
return cmp;
}
};
}
public static void main(String[] args) {
int n = 11;
double fracNoise = 0.1;
Random random = new Random();
double [] a = new double[n];
double [] b = new double[n];
for (int i = 0; i < n; i++) {
a[i] = random.nextDouble();
b[i] = random.nextDouble();
}
ModelError meRaw = ModelError.calculateError(new Matrix(false, a), new Matrix(false, b));
System.out.println(meRaw.toString());
Arrays.sort(a);
Arrays.sort(b);
ModelError meSort = ModelError.calculateError(new Matrix(false, a), new Matrix(false, b));
System.out.println(meSort.toString());
for (int i = 0; i < n; i++) {
if(random.nextDouble()
© 2015 - 2025 Weber Informatics LLC | Privacy Policy