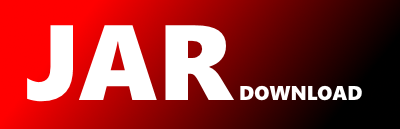
com.actelion.research.orbit.imageAnalysis.tasks.ClassificationTaskTiled Maven / Gradle / Ivy
Go to download
Roaring bitmaps are compressed bitmaps (also called bitsets) which tend to outperform
conventional compressed bitmaps such as WAH or Concise.
Please wait ...