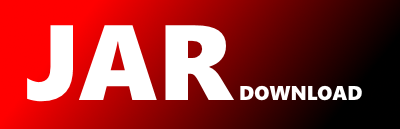
com.amazon.deequ.examples.AnomalyDetectionExample.scala Maven / Gradle / Ivy
Go to download
Show more of this group Show more artifacts with this name
Show all versions of deequ Show documentation
Show all versions of deequ Show documentation
Deequ is a library built on top of Apache Spark for defining "unit tests for data",
which measure data quality in large datasets.
/**
* Copyright 2018 Amazon.com, Inc. or its affiliates. All Rights Reserved.
*
* Licensed under the Apache License, Version 2.0 (the "License"). You may not
* use this file except in compliance with the License. A copy of the License
* is located at
*
* http://aws.amazon.com/apache2.0/
*
* or in the "license" file accompanying this file. This file is distributed on
* an "AS IS" BASIS, WITHOUT WARRANTIES OR CONDITIONS OF ANY KIND, either
* express or implied. See the License for the specific language governing
* permissions and limitations under the License.
*
*/
package com.amazon.deequ.examples
import com.amazon.deequ.VerificationSuite
import com.amazon.deequ.analyzers.Size
import com.amazon.deequ.anomalydetection.RelativeRateOfChangeStrategy
import com.amazon.deequ.examples.ExampleUtils.{itemsAsDataframe, withSpark}
import com.amazon.deequ.repository.ResultKey
import com.amazon.deequ.repository.memory.InMemoryMetricsRepository
import com.amazon.deequ.checks.CheckStatus._
private[examples] object AnomalyDetectionExample extends App {
withSpark { session =>
/* In this simple example, we assume that we compute metrics on a dataset every day and we want
to ensure that they don't change drastically. For sake of simplicity, we just look at the
size of the data */
/* Anomaly detection operates on metrics stored in a metric repository, so lets create one */
val metricsRepository = new InMemoryMetricsRepository()
/* This is the key which we use to store the metrics for the dataset from yesterday */
val yesterdaysKey = ResultKey(System.currentTimeMillis() - 24 * 60 * 1000)
/* Yesterday, the data had only two rows */
val yesterdaysDataset = itemsAsDataframe(session,
Item(1, "Thingy A", "awesome thing.", "high", 0),
Item(2, "Thingy B", "available at http://thingb.com", null, 0))
/* We test for anomalies in the size of the data, it should not increase by more than 2x. Note
that we store the resulting metrics in our repository */
VerificationSuite()
.onData(yesterdaysDataset)
.useRepository(metricsRepository)
.saveOrAppendResult(yesterdaysKey)
.addAnomalyCheck(
RelativeRateOfChangeStrategy(maxRateIncrease = Some(2.0)),
Size()
)
.run()
/* Todays data has five rows, so the data size more than doubled and our anomaly check should
catch this */
val todaysDataset = itemsAsDataframe(session,
Item(1, "Thingy A", "awesome thing.", "high", 0),
Item(2, "Thingy B", "available at http://thingb.com", null, 0),
Item(3, null, null, "low", 5),
Item(4, "Thingy D", "checkout https://thingd.ca", "low", 10),
Item(5, "Thingy E", null, "high", 12))
/* The key for today's result */
val todaysKey = ResultKey(System.currentTimeMillis())
/* Repeat the anomaly check for today's data */
val verificationResult = VerificationSuite()
.onData(todaysDataset)
.useRepository(metricsRepository)
.saveOrAppendResult(todaysKey)
.addAnomalyCheck(
RelativeRateOfChangeStrategy(maxRateIncrease = Some(2.0)),
Size()
)
.run()
/* Did we find an anomaly? */
if (verificationResult.status != Success) {
println("Anomaly detected in the Size() metric!")
/* Lets have a look at the actual metrics. */
metricsRepository
.load()
.forAnalyzers(Seq(Size()))
.getSuccessMetricsAsDataFrame(session)
.show()
}
}
}
© 2015 - 2025 Weber Informatics LLC | Privacy Policy