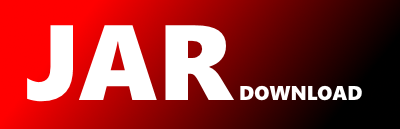
com.articulate.sigma.nlp.SVM Maven / Gradle / Ivy
Go to download
Show more of this group Show more artifacts with this name
Show all versions of sigma-component Show documentation
Show all versions of sigma-component Show documentation
Sigma knowledge engineering system is an system for developing, viewing and debugging theories in first
order logic. It works with Knowledge Interchange Format (KIF) and is optimized for the Suggested Upper Merged
Ontology (SUMO) www.ontologyportal.org.
The newest version!
package com.articulate.sigma.nlp;
import java.util.*;
import java.lang.*;
import java.io.*;
import java.rmi.*;
import java.math.*;
/** ***************************************************************
* SVM-JAVA: A simple Java implementation of SMO (Sequential Minimal Optimization) for training SVM
Developed by
Xiaoqian Jiang ([email protected])
Hwanjo Yu ([email protected])
Department of Computer Science and Engineering, Pohang University of Science and Technology (POSTECH),
http://iis.hwanjoyu.org/svm-java, 2008
[note that current URL is https://sites.google.com/site/postechdm/research/implementation/svm-java ]
Bibtex entry:
@MISC{POSTECH08svm-java,
author = "X. Jiang and H. Yu",
title = "{SVM-JAVA}: A Java Implementation of the {SMO} (Sequential Minimal Optimization) for training {SVM}",
institution = "Department of Computer Science and Engineering, Pohang University of Science and Technology (POSTECH)",
address = "http://iis.hwanjoyu.org/svm-java",
year = "2008"
}
* Edited by apease on 4/21/15.
*/
public class SVM {
class sparse_binary_vector {
Vector id = new Vector();
}
class sparse_vector {
Vector id = new Vector();
Vector val = new Vector();
}
public int N = 0; /* N points(rows) */
public int d = -1; /* d variables */
public float C = (float) 0.05;
public float tolerance = (float) 0.001;
public float eps = (float) 0.001;
public float two_sigma_squared = 2;
public int MATRIX = 4000;
Vector alph = new Vector(); /* Lagrange multipliers */
float b = 0; /* threshold */
Vector w = new Vector(); /* weight vector: only for linear kernel */
Vector error_cache = new Vector();
Vector dense_vector = new Vector();
public boolean is_sparse_data = false;
public boolean is_binary = false;
public boolean is_libsvm_file = true;
int learned_func_flag = -1;
int dot_product_flag = -1;
int kernel_flag = -1;
Vector sparse_binary_points = new Vector();
Vector sparse_points = new Vector();
float dense_points[][] = new float[MATRIX][MATRIX];
Vector target = new Vector();
boolean is_test_only = false;
boolean is_linear_kernel = false;
/* data points with index in [first_test_i .. N)
* will be tested to compute error rate
*/
int first_test_i = 0;
/*
* support vectors are within [0..end_support_i)
*/
int end_support_i = -1;
float delta_b = 0;
Vector precomputed_self_dot_product = new Vector();
float precomputed_dot_product[][] = new float[MATRIX][MATRIX];
/** ***************************************************************
* Initialize the matrix with 0's
*/
public SVM() {
for (int i = 0; i < MATRIX; i++)
for (int j = 0; j < MATRIX; j++)
dense_points[i][j] = 0;
}
/** ***************************************************************
*/
private float object2float(Object o) {
Float result = (Float) o;
return result.floatValue();
}
/** ***************************************************************
*/
private int object2int(Object o) {
Integer result = (Integer) o;
return result.intValue();
}
/** ***************************************************************
*/
private void setVector(Vector v, int location, float value) {
Float result = new Float(value);
v.set(location,result);
}
/** ***************************************************************
*/
private void setVector(Vector v, int location, int value) {
Integer result = new Integer(value);
v.set(location,result);
}
/** ***************************************************************
*/
private float getFloatValue(Vector v, int location) {
Float result = (Float) v.elementAt(location);
return result.floatValue();
}
/** ***************************************************************
*/
private int getIntValue(Vector v, int location) {
Integer result = (Integer) v.elementAt(location);
return result.intValue();
}
/** ***************************************************************
*/
private int examineExample(int i1) {
float y1 = 0, alph1 = 0, E1 = 0, r1 = 0;
y1 = object2int(target.elementAt(i1));
alph1 = object2float(alph.elementAt(i1));
if (alph1 > 0 && alph1 < C)
E1 = object2float(error_cache.elementAt(i1));
else
E1 = learned_func(i1,learned_func_flag) - y1;
r1 = y1 * E1;
if ((r1 < -tolerance && alph1 < C) || (r1 > tolerance && alph1 > 0)) {
{
int k = 0, i2 = 0;
float tmax = 0;
for (i2 = (-1), tmax = 0, k = 0; k < end_support_i; k++)
if (object2float(alph.elementAt(k)) > 0 && object2float(alph.elementAt(k)) < C) {
float E2 = 0, temp = 0;
E2 = object2float(error_cache.elementAt(k));
temp = Math.abs(E1 - E2);
if (temp > tmax) {
tmax = temp;
i2 = k;
}
}
if (i2 >= 0) {
if (takeStep (i1, i2) == 1) {
return 1;
}
}
}
float rands = 0;
{
int k = 0, k0 = 0;
int i2 = 0;
for (rands = (float) Math.random(), k0 = (int) (rands * end_support_i), k = k0; k < end_support_i + k0; k++) {
i2 = k % end_support_i;
if (object2float(alph.elementAt(i2)) > 0 && object2float(alph.elementAt(i2)) < C) {
if (takeStep(i1, i2) == 1) {
return 1;
}
}
}
}
{
int k0 = 0, k = 0, i2 = 0;
rands = 0;
for (rands = (float) Math.random(),k0 = (int)(rands * end_support_i), k = k0; k < end_support_i + k0; k++) {
i2 = k % end_support_i;
if (takeStep(i1, i2) == 1) {
return 1;
}
}
}
}
return 0;
}
/** ***************************************************************
*/
private int takeStep(int i1, int i2) {
int y1 = 0, y2 = 0, s = 0;
float alph1 = 0, alph2 = 0; /* old_values of alpha_1, alpha_2 */
float a1 = 0, a2 = 0; /* new values of alpha_1, alpha_2 */
float E1 = 0, E2 = 0, L = 0, H = 0, k11 = 0, k22 = 0, k12 = 0, eta = 0, Lobj = 0, Hobj = 0;
if (i1 == i2) return 0;
alph1 = object2float(alph.elementAt(i1));
y1 = object2int(target.elementAt(i1));
if (alph1 > 0 && alph1 < C)
E1 = object2float(error_cache.elementAt(i1));
else
E1 = learned_func(i1,learned_func_flag) - y1;
alph2 = object2float(alph.elementAt(i2));
y2 = object2int(target.elementAt(i2));
if (alph2 > 0 && alph2 < C)
E2 = object2float(error_cache.elementAt(i2));
else
E2 = learned_func(i2,learned_func_flag) - y2;
s = y1 * y2;
if (y1 == y2) {
float gamma = alph1 + alph2;
if (gamma > C) {
L = gamma-C;
H = C;
}
else {
L = 0;
H = gamma;
}
}
else {
float gamma = alph1 - alph2;
if (gamma > 0) {
L = 0;
H = C - gamma;
}
else {
L = -gamma;
H = C;
}
}
if (L == H) {
return 0;
}
k11 = kernel_func(i1, i1,kernel_flag);
k12 = kernel_func(i1, i2,kernel_flag);
k22 = kernel_func(i2, i2,kernel_flag);
eta = 2 * k12 - k11 - k22;
if (eta < 0) {
a2 = alph2 + y2 * (E2 - E1) / eta;
if (a2 < L)
a2 = L;
else if (a2 > H)
a2 = H;
}
else {
{
float c1 = eta/2;
float c2 = y2 * (E1-E2)- eta * alph2;
Lobj = c1 * L * L + c2 * L;
Hobj = c1 * H * H + c2 * H;
}
if (Lobj > Hobj+eps)
a2 = L;
else if (Lobj < Hobj-eps)
a2 = H;
else
a2 = alph2;
}
if (Math.abs(a2-alph2) < eps*(a2+alph2+eps))
return 0;
a1 = alph1 - s * (a2 - alph2);
if (a1 < 0) {
a2 += s * a1;
a1 = 0;
}
else if (a1 > C) {
float t = a1-C;
a2 += s * t;
a1 = C;
}
{
float b1 = 0, b2 = 0, bnew = 0;
if (a1 > 0 && a1 < C)
bnew = b + E1 + y1 * (a1 - alph1) * k11 + y2 * (a2 - alph2) * k12;
else {
if (a2 > 0 && a2 < C)
bnew = b + E2 + y1 * (a1 - alph1) * k12 + y2 * (a2 - alph2) * k22;
else {
b1 = b + E1 + y1 * (a1 - alph1) * k11 + y2 * (a2 - alph2) * k12;
b2 = b + E2 + y1 * (a1 - alph1) * k12 + y2 * (a2 - alph2) * k22;
bnew = (b1 + b2) / 2;
}
}
delta_b = bnew - b;
b = bnew;
}
if (is_linear_kernel) {
float t1 = y1 * (a1 - alph1);
float t2 = y2 * (a2 - alph2);
if (is_sparse_data && is_binary) {
int p1 = 0, num1 = 0, p2 = 0, num2 = 0;
num1 = ((sparse_binary_vector) sparse_binary_points.elementAt(i1)).id.size();
for (p1 = 0; p1 < num1; p1++) {
int temp0 = object2int(((sparse_binary_vector) sparse_binary_points.elementAt(i1)).id.elementAt(p1));
float temp = object2float(w.elementAt(temp0));
w.set(temp0,new Float(temp + t1));
}
num2 = ((sparse_binary_vector) sparse_binary_points.elementAt(i2)).id.size();
for (p2 = 0; p2 < num2; p2++) {
int temp0 = object2int(((sparse_binary_vector) sparse_binary_points.elementAt(i2)).id.elementAt(p2));
float temp = object2float(w.elementAt(temp0));
w.set(temp0,new Float(temp + t2));
}
}
else if (is_sparse_data && !is_binary) {
int p1 = 0,num1 = 0,p2 = 0,num2 = 0;
num1 = ((sparse_vector)sparse_points.elementAt(i1)).id.size();
for (p1 = 0; p1 < num1; p1++) {
int temp1 = object2int(((sparse_vector) sparse_points.elementAt(i1)).id.elementAt(p1));
float temp = object2float(w.elementAt(temp1));
float temp2 = object2float(((sparse_vector) sparse_points.elementAt(i1)).val.elementAt(p1));
w.set(temp1,new Float(temp + t1 * temp2));
}
num2 = ((sparse_vector)sparse_points.elementAt(i2)).id.size();
for (p2 = 0; p2 < num2; p2++) {
int temp1 = object2int(((sparse_vector) sparse_points.elementAt(i2)).id.elementAt(p2));
float temp = object2float(w.elementAt(temp1));
float temp2 = object2float(((sparse_vector) sparse_points.elementAt(i2)).val.elementAt(p2));
temp = temp + t2 * temp2;
Float value = new Float(temp);
w.set(temp1,value);
}
}
else
for (int i = 0; i < d; i++) {
float temp = dense_points[i1][i] * t1 + dense_points[i2][i] * t2;;
float temp1 = object2float(w.elementAt(i));
Float value = new Float(temp + temp1);
w.set(i,value);
}
}
{
float t1 = y1 * (a1 - alph1);
float t2 = y2 * (a2 - alph2);
for (int i = 0; i < end_support_i; i++)
if (0 < object2float(alph.elementAt(i)) && object2float(alph.elementAt(i)) < C) {
float tmp = object2float(error_cache.elementAt(i));
tmp += t1 * kernel_func(i1,i,kernel_flag) + t2 * kernel_func(i2,i,kernel_flag)
- delta_b;
error_cache.set(i,new Float(tmp));
}
error_cache.set(i1,new Float(0));
error_cache.set(i2,new Float(0));
}
alph.set(i1,new Float(a1));
alph.set(i2,new Float(a2));
return 1;
}
/** ***************************************************************
*/
private float learned_func_linear_sparse_binary(int k) {
float s = 0;
int temp =0;
for (int i = 0; i < ((sparse_binary_vector) sparse_binary_points.elementAt(k)).id.size(); i++) {
temp = object2int(((sparse_binary_vector) sparse_binary_points.elementAt(i)).id.elementAt(i));
s += object2float(w.elementAt(temp));
}
s -= b;
return s;
}
/** ***************************************************************
*/
private float learned_func_linear_sparse_nonbinary(int k) {
float s = 0;
for (int i = 0; i < ((sparse_vector) sparse_points.elementAt(k)).id.size(); i++) {
int j = object2int (((sparse_vector) sparse_points.elementAt(k)).id.elementAt(i));
float v = object2float (((sparse_vector) sparse_points.elementAt(k)).val.elementAt(i));
s += object2float(w.elementAt(j)) * v;
}
s -= b;
return s;
}
/** ***************************************************************
*/
private float learned_func_linear_dense(int k) {
float s = 0;
for (int i = 0; i < d; i++)
s += object2float(w.elementAt(i)) * dense_points[k][i];
s -= b;
return s;
}
/** ***************************************************************
*/
private float learned_func_nonlinear(int k) {
float s = 0;
for (int i = 0; i < end_support_i; i++)
if (object2float(alph.elementAt(i)) > 0) {
s += object2float(alph.elementAt(i)) * object2int(target.elementAt(i)) * kernel_func(i,k,kernel_flag);
}
s -= b;
return s;
}
/** ***************************************************************
*/
private float dot_product_sparse_binary(int i1, int i2) {
int p1 = 0, p2 = 0, dot = 0;
int num1 = ((sparse_binary_vector) sparse_binary_points.elementAt(i1)).id.size();
int num2 = ((sparse_binary_vector) sparse_binary_points.elementAt(i2)).id.size();
while (p1 < num1 && p2 < num2) {
int a1 = object2int(((sparse_binary_vector) sparse_binary_points.elementAt(i1)).id.elementAt(p1));
int a2 = object2int(((sparse_binary_vector) sparse_binary_points.elementAt(i2)).id.elementAt(p2));
if (a1 == a2) {
dot++;
p1++;
p2++;
}
else if (a1 > a2)
p2++;
else
p1++;
}
return (float)dot;
}
/** ***************************************************************
*/
private float dot_product_sparse_nonbinary(int i1, int i2) {
int p1 = 0, p2 = 0;
float dot = 0;
int num1 = ((sparse_vector) sparse_points.elementAt(i1)).id.size();
int num2 = ((sparse_vector) sparse_points.elementAt(i2)).id.size();
while (p1 < num1 && p2 < num2) {
int a1 = object2int(((sparse_vector) sparse_points.elementAt(i1)).id.elementAt(p1));
int a2 = object2int(((sparse_vector) sparse_points.elementAt(i2)).id.elementAt(p2));
if (a1 == a2) {
float val1 = object2float(((sparse_vector) sparse_points.elementAt(i1)).val.elementAt(p1));
float val2 = object2float(((sparse_vector) sparse_points.elementAt(i2)).val.elementAt(p2));
dot += val1 * val2;
p1++;
p2++;
}
else if (a1 > a2)
p2++;
else
p1++;
}
return (float) dot;
}
/** ***************************************************************
*/
private float dot_product_dense(int i1, int i2) {
float dot = 0;
for (int i = 0; i < d; i++)
dot += dense_points[i1][i] * dense_points[i2][i];
return dot;
}
/** ***************************************************************
*/
private float rbf_kernel(int i1, int i2) {
float s = this.precomputed_dot_product[i1][i2];
s *= -2;
s += object2float(precomputed_self_dot_product.elementAt(i1))
+ object2float(precomputed_self_dot_product.elementAt(i2));
return (float)Math.exp((float)(-s / two_sigma_squared));
}
/** ***************************************************************
* Read the data from a stream
* sparse data is in the form
*
© 2015 - 2025 Weber Informatics LLC | Privacy Policy