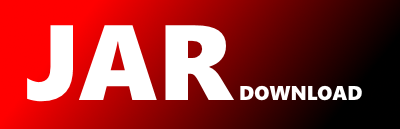
com.brettonw.math.AgglomeratedHierarchy Maven / Gradle / Ivy
Go to download
Show more of this group Show more artifacts with this name
Show all versions of clustering Show documentation
Show all versions of clustering Show documentation
Clustering is a Java library for identifying similar data in n-dimensional spaces.
package com.brettonw.math;
import org.apache.logging.log4j.LogManager;
import org.apache.logging.log4j.Logger;
import java.util.ArrayList;
import java.util.HashMap;
import java.util.List;
import java.util.Map;
public class AgglomeratedHierarchy extends ClusterAlgorithm {
private static final Logger log = LogManager.getLogger (AgglomeratedHierarchy.class);
private abstract class Cluster {
private int id;
public Cluster (int id) {
this.id = id;
}
public int getId () {
return id;
}
public int getPairId () {
return (id << 16) | id;
}
public int[] getSubPairIds () {
return new int[] { id };
}
public Cluster[] getChildren () {
return new Cluster[] {};
}
public abstract int[] getSamples ();
}
private class Single extends Cluster {
private int sample;
public Single (int sample) {
super(sample);
this.sample = sample;
}
@Override
public int[] getSamples () {
return new int[] { sample };
}
}
private class Pair extends Cluster {
private Cluster a;
private Cluster b;
public Pair (int id, Cluster a, Cluster b) {
super(id);
this.a = a;
this.b = b;
}
@Override
public int getPairId () {
return makePairId (a, b);
}
@Override
public int[] getSubPairIds () {
return new int[] { a.getPairId (), b.getPairId () };
}
@Override
public Cluster[] getChildren () {
return new Cluster[] { a, b };
}
@Override
public int[] getSamples () {
int[] aSamples = a.getSamples ();
int[] bSamples = b.getSamples ();
int[] result = new int[aSamples.length + bSamples.length];
System.arraycopy (aSamples, 0, result, 0, aSamples.length);
System.arraycopy (bSamples, 0, result, aSamples.length, bSamples.length);
return result;
}
public double minDistance () {
double result = Double.MAX_VALUE;
int[] aSubPairIds = a.getSubPairIds ();
int[] bSubPairIds = b.getSubPairIds ();
for (int i = 0, aSubPairIdsLength = aSubPairIds.length; i < aSubPairIdsLength; ++i) {
int aSubPairId = aSubPairIds[i];
for (int j = 0, bSubPairIdsLength = bSubPairIds.length; j < bSubPairIdsLength; ++j) {
int bSubPairId = bSubPairIds[j];
int pairId = makePairId (aSubPairId, bSubPairId);
Double distance = distances.get (pairId);
if (distance != null) {
result = Math.min (result, distance);
}
}
}
return result;
}
public double maxDistance () {
double result = 0.0;
int[] aSubPairIds = a.getSubPairIds ();
int[] bSubPairIds = b.getSubPairIds ();
for (int i = 0, aSubPairIdsLength = aSubPairIds.length; i < aSubPairIdsLength; ++i) {
int aSubPairId = aSubPairIds[i];
for (int j = 0, bSubPairIdsLength = bSubPairIds.length; j < bSubPairIdsLength; ++j) {
int bSubPairId = bSubPairIds[j];
int pairId = makePairId (aSubPairId, bSubPairId);
Double distance = distances.get (pairId);
if (distance != null) {
result = Math.max (result, distance);
}
}
}
return result;
}
public double meanDistance () {
double result = 0.0;
int[] aSamples = a.getSamples ();
int[] bSamples = b.getSamples ();
for (int i = 0, aSamplesLength = aSamples.length; i < aSamplesLength; ++i) {
int aSample = aSamples[i];
for (int j = 0, bSamplesLength = bSamples.length; j < bSamplesLength; ++j) {
int bSample = bSamples[j];
// at this level, the cluster ids are the same as the index in the distances
int pairId = makePairId (aSample, bSample);
Double distance = distances.get (pairId);
result += distance;
}
}
return result / (aSamples.length + bSamples.length);
}
public double centroidDistance () {
int[] aSamples = a.getSamples ();
Tuple[] aTuples = dataSet.getTuples (aSamples);
Tuple aCentroid = Tuple.average (aTuples);
int[] bSamples = b.getSamples ();
Tuple[] bTuples = dataSet.getTuples (bSamples);
Tuple bCentroid = Tuple.average (bTuples);
return Tuple.deltaNorm (aCentroid, bCentroid);
}
}
public static final int USE_MIN_DISTANCE = 0;
public static final int USE_MAX_DISTANCE = 1;
public static final int USE_MEAN_DISTANCE = 2;
public static final int USE_CENTROID_DISTANCE = 3;
private List clusters;
private Map distances;
public static int makePairId (Cluster a, Cluster b) {
return makePairId (a.getId (), b.getId ());
}
public static int makePairId (int aId, int bId) {
return (aId << 16) | bId;
}
public AgglomeratedHierarchy (DataSet dataSet, int linkage) {
super (dataSet);
int n = dataSet.getN ();
distances = new HashMap<> (n * 2);
// create a list of all clusters, this will start out as size n, but will then be trimmed
// down to just a single entry by the time we are finished
log.info ("Building " + n + " clusters");
clusters = new ArrayList<> (n);
for (int i = 0; i < n; ++i) {
clusters.add (new Single (i));
}
// pre-cache the pairwise cluster distances - - yes, this is n^2
log.info ("Pre-computing distances for " + ((n - 1) * (n - 1)) + " pairs");
for (int i = 0, end = n - 1; i < end; ++i) {
Cluster iCluster = clusters.get (i);
Tuple iTuple = dataSet.get (iCluster.getSamples ()[0]);
for (int j = i + 1; j < n; ++j) {
Cluster jCluster = clusters.get (j);
Tuple jTuple = dataSet.get (jCluster.getSamples ()[0]);
int pairId = makePairId (iCluster, jCluster);
distances.put (pairId, Tuple.deltaNorm (iTuple, jTuple));
}
}
// loop over the data set identifying the next pair to extract, keep doing that as long as
// there are potential pairs
int nextId = n;
while ((n = clusters.size ()) > 1) {
// loop over every possible pair to find the smallest distance to extract - this is an
// awful n^2 algorithm. I have a sneaky suspicion we could do better with a heap
// approach, but I'll have to come back to that another time - this is in the interest
// of simplicity
log.info ("Scan over " + ((n - 1) * (n - 1)) + " pair(s)");
double min = Double.MAX_VALUE;
int iFinal = 0;
int jFinal = 0;
for (int i = 0, end = n - 1; i < end; ++i) {
Cluster iCluster = clusters.get (i);
for (int j = i + 1; j < n; ++j) {
Cluster jCluster = clusters.get (j);
Pair pair = new Pair (-1, iCluster, jCluster);
int pairId = pair.getPairId ();
double distance = 0;
Double cachedDistance = distances.get (pairId);
if (cachedDistance != null) {
distance = cachedDistance;
} else {
switch (linkage) {
case USE_MIN_DISTANCE: distance = pair.minDistance (); break;
case USE_MAX_DISTANCE: distance = pair.maxDistance (); break;
case USE_MEAN_DISTANCE: distance = pair.meanDistance (); break;
case USE_CENTROID_DISTANCE: distance = pair.centroidDistance (); break;
}
distances.put (pairId, distance);
}
if (distance < min) {
min = distance;
iFinal = i;
jFinal = j;
}
}
}
// remove the two closest clusters, and replace them with a new, combined cluster, we
// have to order the removals so that the array order doesn't change for the second one
Cluster iCluster = clusters.get (iFinal);
Cluster jCluster = clusters.get (jFinal);
if (iFinal > jFinal) {
clusters.remove (iFinal);
clusters.remove (jFinal);
} else {
clusters.remove (jFinal);
clusters.remove (iFinal);
}
clusters.add (new Pair (nextId++, iCluster, jCluster));
}
log.info ("Finished");
}
@Override
public int getClusterCount () {
return dataSet.getN ();
}
@Override
public Tuple[] getCluster (int i) {
// the ith cluster represents a cut in the dendrogram (the tree we built) - a breadth-first
// numbering of the tree roots
return new Tuple[0];
}
}
© 2015 - 2025 Weber Informatics LLC | Privacy Policy