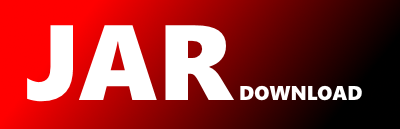
com.brettonw.math.DensityBasedScan Maven / Gradle / Ivy
Go to download
Show more of this group Show more artifacts with this name
Show all versions of clustering Show documentation
Show all versions of clustering Show documentation
Clustering is a Java library for identifying similar data in n-dimensional spaces.
package com.brettonw.math;
import org.apache.logging.log4j.LogManager;
import org.apache.logging.log4j.Logger;
import java.util.ArrayList;
import java.util.Arrays;
import java.util.List;
// https://en.wikipedia.org/wiki/DBSCAN
public class DensityBasedScan extends ClusterAlgorithm {
private static final Logger log = LogManager.getLogger (DensityBasedScan.class);
// the assign array contains either UNTOUCHED, NOISE, or a cluster index
private static final int FIRST_CLUSTER = 0;
private static final int UNTOUCHED = -1;
private static final int NOISE = -2;
private int clusterCount;
private int[] assign;
public DensityBasedScan (DataSet dataSet, double range, int minPts) {
super (dataSet);
// initialize the clustering engine
assign = new int[dataSet.getN ()];
Arrays.fill (assign, UNTOUCHED);
clusterCount = FIRST_CLUSTER;
// scan over all the points...
for (int i = 0, n = dataSet.getN (); i < n; ++i) {
if (assign[i] == UNTOUCHED) {
// get the neighbors
Tuple tuple = dataSet.get (i);
int[] neighbors = dataSet.rangeSearch (tuple, range);
if (neighbors.length < minPts) {
assign[i] = NOISE;
} else {
assign[i] = clusterCount;
expandCluster (neighbors, range, minPts);
++clusterCount;
}
}
}
}
/*
private boolean neighborhoodPredicate (Tuple a, Tuple b) {
double epsilon = 1.0e-1;
return Tuple.deltaNorm (a, b) < epsilon;
}
private double densityPredicate (Tuple[] neighborhood) {
return neighborhood.length;
}
*/
private void expandCluster (int[] neighbors, double range, int minPts) {
for (int i : neighbors) {
if (assign[i] == UNTOUCHED) {
assign[i] = clusterCount;
// get the neighbors
Tuple tuple = dataSet.get (i);
int[] neighborNeighbors = dataSet.rangeSearch (tuple, range);
if (neighborNeighbors.length > minPts) {
expandCluster (neighborNeighbors, range, minPts);
}
}
}
}
public int getClusterCount () {
return clusterCount;
}
@Override
public Tuple[] getCluster (int i) {
// naive scan of the full list
List list = new ArrayList<> ();
// an exhaustive search over all the tuples to find tuples in the cluster
for (int j = 0, n = dataSet.getN (); j < n; ++j) {
if (assign[j] == i) {
list.add (dataSet.get (j));
}
}
return list.toArray (new Tuple[list.size ()]);
}
}
/* Wikipedia (https://en.wikipedia.org/wiki/DBSCAN)
DBSCAN(D, eps, MinPts) {
C = 0
for each point P in dataset D {
if P is visited
continue next point
mark P as visited
NeighborPts = regionQuery(P, eps)
if sizeof(NeighborPts) < MinPts
mark P as NOISE
else {
C = next cluster
expandCluster(P, NeighborPts, C, eps, MinPts)
}
}
}
expandCluster(P, NeighborPts, C, eps, MinPts) {
add P to cluster C
for each point P' in NeighborPts {
if P' is not visited {
mark P' as visited
NeighborPts' = regionQuery(P', eps)
if sizeof(NeighborPts') >= MinPts
NeighborPts = NeighborPts joined with NeighborPts'
}
if P' is not yet member of any cluster
add P' to cluster C
}
}
*/
© 2015 - 2025 Weber Informatics LLC | Privacy Policy