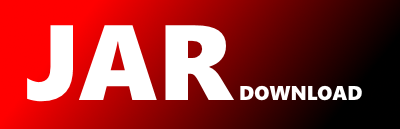
weka.classifiers.immune.airs.algorithm.AIRS2ParallelTrainer Maven / Gradle / Ivy
Go to download
Show more of this group Show more artifacts with this name
Show all versions of wekaclassalgos Show documentation
Show all versions of wekaclassalgos Show documentation
Fork of the following defunct sourceforge.net project: https://sourceforge.net/projects/wekaclassalgos/
/*
* This program is free software: you can redistribute it and/or modify
* it under the terms of the GNU General Public License as published by
* the Free Software Foundation, either version 3 of the License, or
* (at your option) any later version.
*
* This program is distributed in the hope that it will be useful,
* but WITHOUT ANY WARRANTY; without even the implied warranty of
* MERCHANTABILITY or FITNESS FOR A PARTICULAR PURPOSE. See the
* GNU General Public License for more details.
*
* You should have received a copy of the GNU General Public License
* along with this program. If not, see .
*/
/*
* Created on 8/01/2005
*
*/
package weka.classifiers.immune.airs.algorithm;
import weka.classifiers.immune.airs.algorithm.merge.ConcatenateMerge;
import weka.classifiers.immune.airs.algorithm.merge.PruneMerge;
import weka.core.Instances;
import weka.filters.Filter;
import weka.filters.unsupervised.attribute.Normalize;
import java.util.LinkedList;
import java.util.Random;
import java.util.concurrent.CountDownLatch;
/**
* Type: AIRS2ParallelTrainer
* File: AIRS2ParallelTrainer.java
* Date: 8/01/2005
*
* Description:
*
*
* @author Jason Brownlee
*/
public class AIRS2ParallelTrainer implements AISTrainer {
protected final double affinityThresholdScalar;
protected final double clonalRate;
protected final double hyperMutationRate;
protected final double totalResources;
protected final double stimulationThreshold;
protected final int affinityThresholdNumInstances;
protected final Random rand;
protected final int memoryCellPoolInitialSize;
protected final int kNN;
// different means of merging
public enum MERGE_MODE {
CONCATENATE,
PRUNE
}
// additional
protected final int numThreads;
protected final MERGE_MODE mergeMode;
protected double affinityThreshold;
protected MemoryCellMerger merger;
protected CountDownLatch latch;
protected String[] trainingSummaries;
public AIRS2ParallelTrainer(
double aAffinityThresholdScalar,
double aClonalRate,
double aHyperMutationRate,
double aTotalResources,
double aStimulationValue,
int aNumInstancesAffinityThreshold,
Random aRand,
int aMemoryCellPoolInitialSize,
int aKNN,
int aNumThreads,
MERGE_MODE aMergeMode) {
affinityThresholdScalar = aAffinityThresholdScalar;
clonalRate = aClonalRate;
hyperMutationRate = aHyperMutationRate;
totalResources = aTotalResources;
stimulationThreshold = aStimulationValue;
affinityThresholdNumInstances = aNumInstancesAffinityThreshold;
rand = aRand;
memoryCellPoolInitialSize = aMemoryCellPoolInitialSize;
kNN = aKNN;
// additional
numThreads = aNumThreads;
mergeMode = aMergeMode;
}
/**
* @param aInstances
* @return
* @throws Exception
*/
public AISModelClassifier train(Instances aInstances) throws Exception {
// normalise the dataset
Normalize normalise = new Normalize();
normalise.setInputFormat(aInstances);
Instances trainingSet = Filter.useFilter(aInstances, normalise);
// calculate affinity threshold
AffinityFunction affinityFunction = new AffinityFunction(trainingSet);
affinityThreshold = Utils.calculateAffinityThreshold(trainingSet, affinityThresholdNumInstances, rand, affinityFunction);
// prepare latch
latch = new CountDownLatch(numThreads);
// divide up dataset
Instances[] instances = new Instances[numThreads];
trainingSet.randomize(rand);
int numPerThread = (int) Math.round((double) trainingSet.numInstances() / (double) numThreads);
int offset = 0;
for (int i = 0; i < instances.length; i++) {
// check for last - give all remaining
if (i == instances.length - 1) {
instances[i] = new Instances(trainingSet, offset, trainingSet.numInstances() - offset);
}
else {
instances[i] = new Instances(trainingSet, offset, numPerThread);
offset += numPerThread;
}
}
// prepare threads
AIRSProcess[] threads = new AIRSProcess[numThreads];
for (int i = 0; i < threads.length; i++) {
// prep algorithm
AIRS2Trainer algorithm = new AIRS2Trainer(
affinityThresholdScalar,
clonalRate,
hyperMutationRate,
totalResources,
stimulationThreshold,
affinityThresholdNumInstances,
rand,
memoryCellPoolInitialSize,
kNN);
// prepare algorithm - instances only needed for distance measure prep
algorithm.algorithmPreperation(trainingSet);
// create thread
threads[i] = new AIRSProcess(algorithm, instances[i], normalise);
// start the thread - don't need ref to it - got a latch
Thread t = new Thread(threads[i], "AIRS2 Thread Number: " + i); // name for debug if required
t.start();
}
// wait for all threads to complete
latch.await();
// collect memory cells
LinkedList[] cells = new LinkedList[numThreads];
trainingSummaries = new String[numThreads];
for (int i = 0; i < threads.length; i++) {
cells[i] = threads[i].getCells();
trainingSummaries[i] = threads[i].getTrainingSummary();
}
// prepare classifier (merging)
merger = getMeger();
AISModelClassifier classifier = merger.mergeMemoryCells(cells, kNN, normalise, affinityFunction, trainingSet);
return classifier;
}
protected MemoryCellMerger getMeger() {
if (mergeMode == MERGE_MODE.CONCATENATE) {
return new ConcatenateMerge();
}
else if (mergeMode == MERGE_MODE.PRUNE) {
return new PruneMerge();
}
throw new RuntimeException("Unknown merge mode: " + mergeMode);
}
protected final class AIRSProcess implements Runnable {
protected final AIRS2Trainer algorithm;
protected final Instances instances;
protected final Normalize normalise;
protected AISModelClassifier classifier;
public AIRSProcess(
AIRS2Trainer aAlgorithm,
Instances aInstances,
Normalize aNormalise) {
algorithm = aAlgorithm;
instances = aInstances;
normalise = aNormalise;
}
public void run() {
// set the affinity threshold manually
algorithm.setAffinityThreshold(affinityThreshold);
// run training
try {
classifier = algorithm.internalTrain(instances, normalise);
}
catch (Exception e) {
throw new RuntimeException("Failed to prepare classifier partition.", e);
}
finally {
// finished
latch.countDown();
}
}
public LinkedList getCells() {
return classifier.getModel().getCells();
}
public String getTrainingSummary() {
return algorithm.getTrainingSummary();
}
}
/**
* @return
*/
public String getTrainingSummary() {
StringBuffer buffer = new StringBuffer();
buffer.append(" - Parallel Training Summary - \n");
buffer.append("Total Partitions: " + numThreads);
buffer.append("\n\n");
for (int i = 0; i < trainingSummaries.length; i++) {
buffer.append(trainingSummaries[i]);
buffer.append("\n");
}
return buffer.toString();
}
}
|
© 2015 - 2024 Weber Informatics LLC | Privacy Policy