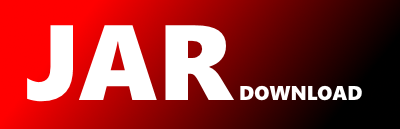
weka.classifiers.immune.airs.algorithm.samplegeneration.StimulationProportionalMutation Maven / Gradle / Ivy
Go to download
Show more of this group Show more artifacts with this name
Show all versions of wekaclassalgos Show documentation
Show all versions of wekaclassalgos Show documentation
Fork of the following defunct sourceforge.net project: https://sourceforge.net/projects/wekaclassalgos/
/*
* This program is free software: you can redistribute it and/or modify
* it under the terms of the GNU General Public License as published by
* the Free Software Foundation, either version 3 of the License, or
* (at your option) any later version.
*
* This program is distributed in the hope that it will be useful,
* but WITHOUT ANY WARRANTY; without even the implied warranty of
* MERCHANTABILITY or FITNESS FOR A PARTICULAR PURPOSE. See the
* GNU General Public License for more details.
*
* You should have received a copy of the GNU General Public License
* along with this program. If not, see .
*/
package weka.classifiers.immune.airs.algorithm.samplegeneration;
import weka.classifiers.immune.airs.algorithm.Cell;
import weka.classifiers.immune.airs.algorithm.SampleGenerator;
import weka.core.Attribute;
import weka.core.Instance;
import java.util.Random;
/**
* Type: StimulationProportionalMutation
* Date: 7/01/2005
*
* @author Jason Brownlee
*/
public class StimulationProportionalMutation extends SampleGenerator {
protected final double[][] minmax;
/**
* @param aRand
*/
public StimulationProportionalMutation(Random aRand) {
this(aRand, null);
}
public StimulationProportionalMutation(Random aRand, double[][] aMinMax) {
super(aRand);
minmax = aMinMax;
}
public Cell generateSample(Cell aCell, Instance aInstance) {
// get the normalised stimulation value
double stimulationValue = aCell.getStimulation();
double mutationRange = 1.0 - stimulationValue;
// clone the cell
Cell cell = new Cell(aCell);
// mutate each gene
// range is (1-normalised stimulation value)
// centre is the genes current value
// all mutations are bounded to [0,1]
double[] data = cell.getAttributes();
for (int i = 0; i < data.length; i++) {
int type = aInstance.attribute(i).type();
// check for class index, can never be mutated
if (i == cell.getClassIndex()) {
continue; // never adjust the class value
}
// check for nominal
else if (type == Attribute.NOMINAL) {
// simply select a new nominal value
data[i] = rand.nextInt(aInstance.attribute(i).numValues());
}
// numeric
else if (type == Attribute.NUMERIC) {
// determine bounds for new value
double min = Math.max(data[i] - (mutationRange / 2.0), 0.0);
double max = Math.min(data[i] + (mutationRange / 2.0), 1.0);
// generate new value in VALID range and store
data[i] = min + (rand.nextDouble() * (max - min));
// validation
if (data[i] > max || data[i] < min) {
throw new RuntimeException("Something is wrong with the mutation scheme.");
}
}
else {
// unsupported type
throw new IllegalArgumentException("Attemted to mutate an attribute type that is unspported: " + type);
}
}
return cell;
}
public Cell generateSameWithoutNormalisation(Cell aCell, Instance aInstance) {
// get the normalised stimulation value
double stimulationValue = aCell.getStimulation();
double mutationRange = 1.0 - stimulationValue;
double[] ranges = new double[minmax.length];
for (int i = 0; i < ranges.length; i++) {
double r = (minmax[i][1] - minmax[i][0]);
ranges[i] = (r * mutationRange); // percentage of overall range
}
// clone the cell
Cell cell = new Cell(aCell);
// mutate each gene
// range is (1-normalised stimulation value)
// centre is the genes current value
// all mutations are bounded to [0,1]
double[] data = cell.getAttributes();
for (int i = 0; i < data.length; i++) {
int type = aInstance.attribute(i).type();
// check for class index, can never be mutated
if (i == cell.getClassIndex()) {
continue; // never adjust the class value
}
// check for nominal
else if (type == Attribute.NOMINAL) {
// simply select a new nominal value
data[i] = rand.nextInt(aInstance.attribute(i).numValues());
}
// numeric
else if (type == Attribute.NUMERIC) {
// determine bounds for new value
double min = Math.max(data[i] - (ranges[i] / 2.0), minmax[i][0]);
double max = Math.min(data[i] + (ranges[i] / 2.0), minmax[i][1]);
// generate new value in VALID range and store
data[i] = min + (rand.nextDouble() * (max - min));
}
else {
// unsupported type
throw new IllegalArgumentException("Attemted to mutate an attribute type that is unspported: " + type);
}
}
return cell;
}
}
© 2015 - 2024 Weber Informatics LLC | Privacy Policy