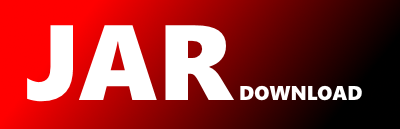
weka.classifiers.neural.common.CommonNeuralAlgorithmAncestor Maven / Gradle / Ivy
Go to download
Show more of this group Show more artifacts with this name
Show all versions of wekaclassalgos Show documentation
Show all versions of wekaclassalgos Show documentation
Fork of the following defunct sourceforge.net project: https://sourceforge.net/projects/wekaclassalgos/
/*
* This program is free software: you can redistribute it and/or modify
* it under the terms of the GNU General Public License as published by
* the Free Software Foundation, either version 3 of the License, or
* (at your option) any later version.
*
* This program is distributed in the hope that it will be useful,
* but WITHOUT ANY WARRANTY; without even the implied warranty of
* MERCHANTABILITY or FITNESS FOR A PARTICULAR PURPOSE. See the
* GNU General Public License for more details.
*
* You should have received a copy of the GNU General Public License
* along with this program. If not, see .
*/
package weka.classifiers.neural.common;
import weka.classifiers.neural.common.transfer.TransferFunction;
import weka.core.Instance;
/**
* Date: 31/05/2004
* File: CommonNeuralAlgorithmAncestor.java
*
* @author Jason Brownlee
*/
public abstract class CommonNeuralAlgorithmAncestor
implements NeuralModel {
/**
* Transfer function
*/
protected final TransferFunction transferFunction;
/**
* Random number generator
*/
protected final RandomWrapper rand;
public CommonNeuralAlgorithmAncestor(TransferFunction aTransferFunction,
RandomWrapper aRand) {
transferFunction = aTransferFunction;
rand = aRand;
}
protected double[] prepareExpectedOutputVector(Instance instance) {
// convert a provided instance into a usable vector of doubles
// which matches the dimension of the output nodes (1-to-1)
double[] expected = new double[getNumOutputNeurons()];
if (instance.classAttribute().isNumeric()) {
expected[0] = instance.classValue();
}
else {
int classValue = (int) instance.classValue();
for (int i = 0; i < expected.length; i++) {
if (i == classValue) {
expected[i] = transferFunction.getMaximum();
}
else {
expected[i] = transferFunction.getMinimum();
}
}
}
return expected;
}
/**
* Responsible for returning a class distribution for the provided instance.
* Each element is given as a fractional part of the output vectors
* magnitude.
*
* @param instance - the instance to retrieve the class distribution for
* @return double - class distribution vecotr for classification problems otherwise
* the raw network output for regression problems
*/
public double[] getDistributionForInstance(Instance instance) {
// get the network output
double[] output = getNetworkOutputs(instance);
// if the class is nominal, prepare a class distribution as the output
if (instance.classAttribute().isNominal()) {
// normalise the output
Utils.normalise(output);
// sum the vector
double sum = 0.0;
for (int i = 0; i < output.length; i++) {
sum += output[i];
}
// calculate each value as a percentage of the whole (sum to 1.0)
for (int i = 0; i < output.length; i++) {
output[i] = (output[i] / sum);
}
}
// else return output as is
// return the class distribution
return output;
}
protected double transfer(double activation) {
return transferFunction.overflowProtectionTransfer(activation);
}
protected double derivative(double activation, double transferred) {
return transferFunction.derivative(activation, transferred);
}
protected double activate(SimpleNeuron neuron, Instance inputs) {
return neuron.activate(inputs);
}
protected double activate(SimpleNeuron neuron, double[] inputs) {
return neuron.activate(inputs);
}
}
© 2015 - 2024 Weber Informatics LLC | Privacy Policy