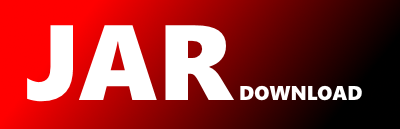
weka.classifiers.neural.common.SimpleNeuron Maven / Gradle / Ivy
Go to download
Show more of this group Show more artifacts with this name
Show all versions of wekaclassalgos Show documentation
Show all versions of wekaclassalgos Show documentation
Fork of the following defunct sourceforge.net project: https://sourceforge.net/projects/wekaclassalgos/
/*
* This program is free software: you can redistribute it and/or modify
* it under the terms of the GNU General Public License as published by
* the Free Software Foundation, either version 3 of the License, or
* (at your option) any later version.
*
* This program is distributed in the hope that it will be useful,
* but WITHOUT ANY WARRANTY; without even the implied warranty of
* MERCHANTABILITY or FITNESS FOR A PARTICULAR PURPOSE. See the
* GNU General Public License for more details.
*
* You should have received a copy of the GNU General Public License
* along with this program. If not, see .
*/
package weka.classifiers.neural.common;
import weka.core.Instance;
import java.io.Serializable;
/**
* Title: Weka Neural Implementation
* Description: ...
* Copyright: Copyright (c) 2003
* Company: N/A
*
* @author Jason Brownlee
* @version 1.0
*/
public class SimpleNeuron implements Serializable {
public final static double DEFAULT_BIAS_VALUE = 1.0;
protected final double biasInputValue;
// weights to apply to inputs (num inputs + 1 for the bias)
protected final double[] inputWeights;
// derivatives of error in regard to weights
protected final double[] dEwE;
// last change in each weight
protected final double[] lastWeightDeltas;
// index in the weight vector of the bias weight (always at the end of the array)
protected final int biasIndex;
public SimpleNeuron(int numInputs, double aBiasInput) {
biasInputValue = aBiasInput;
// +1 for the bias value (end)
inputWeights = new double[numInputs + 1];
dEwE = new double[numInputs + 1];
lastWeightDeltas = new double[numInputs + 1];
biasIndex = numInputs; // the end of the array
}
public double activate(Instance instance) {
// calculates the activation given an instance
double result = 0.0;
double[] input = instance.toDoubleArray();
int offset = 0;
for (int i = 0; i < input.length; i++) {
// class values are not included
if (i != instance.classIndex()) {
// never add missing values into the activation
if (instance.isMissing(i)) {
offset++;
}
else {
result += (input[i] * inputWeights[offset++]);
}
}
}
// add the bias output
result += (biasInputValue * inputWeights[biasIndex]);
return result;
}
public double activate(double[] inputs) {
// calculate the activation given an input vector
double result = 0.0;
for (int i = 0; i < inputs.length; i++) {
result += (inputs[i] * inputWeights[i]);
}
// add the bias output
result += (biasInputValue * inputWeights[biasIndex]);
return result;
}
public double[] getdEwE() {
return dEwE;
}
public double[] getLastWeightDeltas() {
return lastWeightDeltas;
}
public double[] getWeights() {
return inputWeights;
}
public int getBiasIndex() {
return biasIndex;
}
/**
* @return
*/
public double getBiasInputValue() {
return biasInputValue;
}
}
© 2015 - 2024 Weber Informatics LLC | Privacy Policy