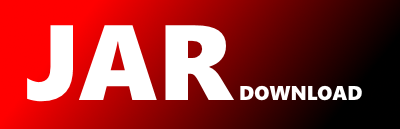
weka.classifiers.neural.common.WekaAlgorithmAncestor Maven / Gradle / Ivy
Go to download
Fork of the following defunct sourceforge.net project: https://sourceforge.net/projects/wekaclassalgos/
/*
* This program is free software: you can redistribute it and/or modify
* it under the terms of the GNU General Public License as published by
* the Free Software Foundation, either version 3 of the License, or
* (at your option) any later version.
*
* This program is distributed in the hope that it will be useful,
* but WITHOUT ANY WARRANTY; without even the implied warranty of
* MERCHANTABILITY or FITNESS FOR A PARTICULAR PURPOSE. See the
* GNU General Public License for more details.
*
* You should have received a copy of the GNU General Public License
* along with this program. If not, see .
*/
package weka.classifiers.neural.common;
import weka.classifiers.AbstractClassifier;
import weka.classifiers.neural.common.learning.LearningKernelFactory;
import weka.classifiers.neural.common.training.NeuralTrainer;
import weka.classifiers.neural.common.training.TrainerFactory;
import weka.classifiers.neural.common.transfer.TransferFunctionFactory;
import weka.core.Capabilities;
import weka.core.Capabilities.Capability;
import weka.core.Instance;
import weka.core.Instances;
import weka.core.Option;
import weka.core.WeightedInstancesHandler;
import java.util.Collection;
import java.util.Enumeration;
import java.util.LinkedList;
import java.util.Vector;
/**
* Title: Weka Neural Implementation
* Description: ...
* Copyright: Copyright (c) 2003
* Company: N/A
*
* @author Jason Brownlee
* @version 1.0
*/
public abstract class WekaAlgorithmAncestor extends AbstractClassifier
implements WeightedInstancesHandler {
private final static int PARAM_TRAINING_ITERATIONS = 0;
private final static int PARAM_LEARNING_RATE = 1;
private final static int PARAM_BIAS_CONSTANT = 2;
private final static int PARAM_RANDOM_SEED = 3;
// param flags
private final static String[] PARAMETERS =
{
"I", // iterations
"L", // learning rate
"B", // bias constant
"R" // random seed
};
// param flags
private final static String[] PARAMETER_NOTES =
{
"", // iterations
"", // learning rate
"", // bias constant
"" // random seed
};
// descriptions for all parameters
private final static String[] PARAM_DESCRIPTIONS =
{
"Number of training iterations (anywhere from a few hundred to a few thousand)",
"Learning Rate - between 0.05 and 0.75 (recommend 0.1 for most cases)",
"Bias constant input, (recommend 1.0, use 0.0 for no bias constant input)",
Constants.DESCRIPTION_RANDOM_SEED
};
// the model
protected NeuralModel model;
protected RandomWrapper rand;
// random number seed
protected long randomNumberSeed = 0;
// learning rate
protected double learningRate = 0.0;
// learning rate function
protected int learningRateFunction = 0;
// bias input constant
protected double biasInput = 0.0;
// transfer function
protected int transferFunction = 0;
// training mode
protected int trainingMode = 0;
// number of training iterations
protected int trainingIterations = 0;
// stats on the dataset used to build the model
protected int numInstances = 0;
protected int numClasses = 0;
protected int numAttributes = 0;
protected boolean classIsNominal = false;
public abstract String globalInfo();
protected abstract void validateArguments() throws Exception;
protected abstract NeuralModel prepareAlgorithm(Instances instances) throws Exception;
protected abstract Collection getListOptions();
protected abstract void setArguments(String[] options) throws Exception;
protected abstract Collection getAlgorithmOptions();
public double[] getAllWeights() {
return model.getAllWeights();
}
public void buildClassifier(Instances instances)
throws Exception {
// prepare the random number seed
rand = new RandomWrapper(randomNumberSeed);
// prepare the dataset for use
Instances trainingInstances = prepareTrainingDataset(instances);
// whether or not the class is nominal
if (trainingInstances.classAttribute().isNominal()) {
classIsNominal = true;
}
else {
classIsNominal = false;
}
// validate user provided arguments
validateAlgorithmArguments();
// initialise the model
model = prepareAlgorithm(trainingInstances);
// build the model
NeuralTrainer trainer = TrainerFactory.factory(trainingMode, rand);
trainer.trainModel(model, trainingInstances, trainingIterations);
}
protected void validateAlgorithmArguments() throws Exception {
// num training iterations
if (trainingIterations <= 0) {
throw new Exception("The number of training iterations must be > 0");
}
// validate child arguments
validateArguments();
}
public double[] distributionForInstance(Instance instance)
throws Exception {
if (model == null) {
throw new Exception("Model has not been constructed");
}
// verify number of classes
if (instance.numClasses() != numClasses) {
throw new Exception("Number of classes in instance (" + instance.numClasses() + ") does not match expected (" + numClasses + ").");
}
// verify the number of attributes
if (instance.numAttributes() != numAttributes) {
throw new Exception("Number of attributes in instance (" + instance.numAttributes() + ") does not match expected (" + numAttributes + ").");
}
// get the network output
double[] output = model.getDistributionForInstance(instance);
// return the class distribution
return output;
}
/**
* Returns the Capabilities of this classifier.
*
* @return the capabilities of this object
* @see Capabilities
*/
@Override
public Capabilities getCapabilities() {
Capabilities result = super.getCapabilities();
result.disableAll();
// attributes
result.enable(Capability.NUMERIC_ATTRIBUTES);
result.enable(Capability.DATE_ATTRIBUTES);
// class
result.enable(Capability.NOMINAL_CLASS);
result.enable(Capability.NUMERIC_CLASS);
result.enable(Capability.MISSING_CLASS_VALUES);
result.setMinimumNumberInstances(1);
return result;
}
protected Instances prepareTrainingDataset(Instances aInstances) throws Exception {
Instances trainingInstances = new Instances(aInstances);
trainingInstances.deleteWithMissingClass();
getCapabilities().testWithFail(trainingInstances);
numInstances = trainingInstances.numInstances();
numClasses = trainingInstances.numClasses();
numAttributes = trainingInstances.numAttributes();
return trainingInstances;
}
public String toString() {
StringBuffer buffer = new StringBuffer(200);
buffer.append("--------------------------------------------");
buffer.append("\n");
// algorithm name
buffer.append(globalInfo() + "\n");
// check if the model has been constructed
if (model == null) {
buffer.append("The model has not been constructed");
}
else {
buffer.append("Random Number Seed: " + randomNumberSeed + "\n");
buffer.append("Learning Rate: " + learningRate + "\n");
buffer.append("Learning Rate Function: " + LearningKernelFactory.getDescription(learningRateFunction) + "\n");
buffer.append("Constant Bias Input: " + biasInput + "\n");
buffer.append("Training Iterations: " + trainingIterations + "\n");
buffer.append("Training Mode: " + TrainerFactory.getDescriptionForMode(trainingMode) + "\n");
buffer.append("Transfer Function " + TransferFunctionFactory.getDescriptionForFunction(transferFunction) + "\n");
buffer.append("\n");
buffer.append(model.getModelInformation());
}
buffer.append("--------------------------------------------");
return buffer.toString();
}
public Enumeration listOptions() {
Vector list = new Vector(PARAMETERS.length);
for (int i = 0; i < PARAMETERS.length; i++) {
String param = "-" + PARAMETERS[i] + " " + PARAMETER_NOTES[i];
list.add(new Option("\t" + PARAM_DESCRIPTIONS[i], PARAMETERS[i], 1, param));
}
Collection c = getListOptions();
if (c != null) {
list.addAll(c);
}
return list.elements();
}
public void setOptions(String[] options)
throws Exception {
String[] values = new String[PARAMETERS.length];
for (int i = 0; i < values.length; i++) {
values[i] = weka.core.Utils.getOption(PARAMETERS[i].charAt(0), options);
}
for (int i = 0; i < values.length; i++) {
String data = values[i];
if (data == null || data.length() == 0) {
continue;
}
switch (i) {
case PARAM_TRAINING_ITERATIONS: {
trainingIterations = Integer.parseInt(data);
break;
}
case PARAM_LEARNING_RATE: {
learningRate = Double.parseDouble(data);
break;
}
case PARAM_BIAS_CONSTANT: {
biasInput = Double.parseDouble(data);
break;
}
case PARAM_RANDOM_SEED: {
randomNumberSeed = Long.parseLong(data);
break;
}
default: {
throw new Exception("Invalid option offset: " + i);
}
}
}
// pass of options to decendents
setArguments(options);
}
protected boolean hasValue(String aString) {
return (aString != null && aString.length() != 0);
}
public String[] getOptions() {
LinkedList list = new LinkedList();
list.add("-" + PARAMETERS[PARAM_TRAINING_ITERATIONS]);
list.add(Integer.toString(trainingIterations));
list.add("-" + PARAMETERS[PARAM_LEARNING_RATE]);
list.add(Double.toString(learningRate));
list.add("-" + PARAMETERS[PARAM_BIAS_CONSTANT]);
list.add(Double.toString(biasInput));
list.add("-" + PARAMETERS[PARAM_RANDOM_SEED]);
list.add(Long.toString(randomNumberSeed));
Collection c = getAlgorithmOptions();
if (c != null) {
list.addAll(c);
}
return (String[]) list.toArray(new String[list.size()]);
}
public String trainingIterationsTipText() {
return PARAM_DESCRIPTIONS[PARAM_TRAINING_ITERATIONS];
}
public String learningRateTipText() {
return PARAM_DESCRIPTIONS[PARAM_LEARNING_RATE];
}
public String biasInputTipText() {
return PARAM_DESCRIPTIONS[PARAM_BIAS_CONSTANT];
}
public String randomNumberSeedTipText() {
return PARAM_DESCRIPTIONS[PARAM_RANDOM_SEED];
}
// accessor and mutator for algorithm parameters
public int getTrainingIterations() {
return trainingIterations;
}
public void setTrainingIterations(int i) {
trainingIterations = i;
}
public double getLearningRate() {
return learningRate;
}
public void setLearningRate(double l) {
learningRate = l;
}
public double getBiasInput() {
return biasInput;
}
public void setBiasInput(double l) {
biasInput = l;
}
public long getRandomNumberSeed() {
return randomNumberSeed;
}
public void setRandomNumberSeed(long l) {
randomNumberSeed = l;
}
}
© 2015 - 2025 Weber Informatics LLC | Privacy Policy