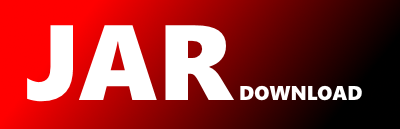
weka.classifiers.neural.lvq.AlgorithmAncestor Maven / Gradle / Ivy
Go to download
Show more of this group Show more artifacts with this name
Show all versions of wekaclassalgos Show documentation
Show all versions of wekaclassalgos Show documentation
Fork of the following defunct sourceforge.net project: https://sourceforge.net/projects/wekaclassalgos/
/*
* This program is free software: you can redistribute it and/or modify
* it under the terms of the GNU General Public License as published by
* the Free Software Foundation, either version 3 of the License, or
* (at your option) any later version.
*
* This program is distributed in the hope that it will be useful,
* but WITHOUT ANY WARRANTY; without even the implied warranty of
* MERCHANTABILITY or FITNESS FOR A PARTICULAR PURPOSE. See the
* GNU General Public License for more details.
*
* You should have received a copy of the GNU General Public License
* along with this program. If not, see .
*/
package weka.classifiers.neural.lvq;
import weka.classifiers.AbstractClassifier;
import weka.classifiers.neural.common.RandomWrapper;
import weka.classifiers.neural.lvq.algorithm.CommonAncestor;
import weka.classifiers.neural.lvq.event.EpochEventListener;
import weka.classifiers.neural.lvq.initialise.InitialisationFactory;
import weka.classifiers.neural.lvq.model.CodebookVector;
import weka.classifiers.neural.lvq.model.CommonModel;
import weka.core.Capabilities;
import weka.core.Capabilities.Capability;
import weka.core.Instance;
import weka.core.Instances;
import weka.core.SelectedTag;
import weka.core.UnsupportedClassTypeException;
import weka.core.WeightedInstancesHandler;
import java.text.NumberFormat;
import java.util.Iterator;
import java.util.LinkedList;
/**
* Date: 25/05/2004
* File: AlgorithmAncestor.java
*
* @author Jason Brownlee
*/
public abstract class AlgorithmAncestor extends AbstractClassifier
implements WeightedInstancesHandler {
protected final LinkedList epochEventListeners;
protected int initialisationMode;
protected boolean useVoting;
protected long seed;
protected int numClasses;
protected int numAttributes;
protected long initialisationTime;
protected long trainingTime;
protected int[][] trainingBmuUsage;
protected int totalTrainingBmuHits;
protected RandomWrapper random;
protected CommonModel model;
protected boolean modelHasBeenPreInitialised;
protected boolean prepareBmuStatistis;
protected double trainingQuantisationError;
protected double trainingAvgQuantisationError;
public abstract String globalInfo();
protected abstract void validateAlgorithmArguments() throws Exception;
protected abstract void initialiseModel(Instances instances);
protected abstract void trainModel(Instances instances) throws Exception;
public abstract int getTotalCodebookVectors();
public AlgorithmAncestor() {
epochEventListeners = new LinkedList();
prepareBmuStatistis = true;
modelHasBeenPreInitialised = false;
}
public void addEpochEventListener(EpochEventListener aListener) {
epochEventListeners.add(aListener);
}
protected void addEventListenersToAlgorithm(CommonAncestor aAlgorithm) {
for (Iterator iter = epochEventListeners.iterator(); iter.hasNext(); ) {
EpochEventListener element = (EpochEventListener) iter.next();
aAlgorithm.addEpochEventListener(element);
}
}
/**
* Build a model of the provided training dataset using the specific LVQ
* algorithm implementation. The model is constructed (if not already provided),
* it is initialised, then the model is trained (constructed) using
* the specific implementation of the LVQ algorithm by calling
* prepareLVQClassifier()
*
* @param instances - training dataset.
* @throws Exception
*/
public void buildClassifier(Instances instances)
throws Exception {
// prepare the dataset for use
Instances trainingInstances = prepareDataset(instances);
// validate user provided arguments
validateAlgorithmArguments();
// construct the model
initialisationTime = System.currentTimeMillis();
random = new RandomWrapper(seed);
if (!modelHasBeenPreInitialised) {
initialiseModel(trainingInstances);
// whether or not to use voting
model.setUseVoting(useVoting);
}
initialisationTime = (System.currentTimeMillis() - initialisationTime);
// train the model
trainingTime = System.currentTimeMillis();
trainModel(trainingInstances);
// calculate bmu hit counts only if the model has not been pre-initialised
if (prepareBmuStatistis) {
model.clearBmuCounts();
for (int i = 0; i < trainingInstances.numInstances(); i++) {
model.classifyInstance(trainingInstances.instance(i));
}
trainingBmuUsage = model.getBmuCounts();
totalTrainingBmuHits = trainingInstances.numInstances();
}
if (m_Debug) {
trainingQuantisationError = calculateQuantisationError(instances);
trainingAvgQuantisationError = (trainingQuantisationError / (double) instances.numInstances());
}
trainingTime = (System.currentTimeMillis() - trainingTime);
}
public void setPreInitialisedModel(CommonModel aModel) {
modelHasBeenPreInitialised = true;
model = aModel;
}
public CommonModel getModel() {
return model;
}
/**
* Calcualte the class distribution for the provided instance
*
* @param instance - an instance to calculate the class distribution for
* @return double [] - class distribution for instance
* @throws Exception
*/
public double[] distributionForInstance(Instance instance)
throws Exception {
if (model == null) {
throw new Exception("Model has not been prepared");
}
// verify number of classes
else if (instance.numClasses() != numClasses) {
throw new Exception("Number of classes in instance (" + instance.numClasses() + ") does not match expected (" + numClasses + ").");
}
// verify the number of attributes
else if (instance.numAttributes() != numAttributes) {
throw new Exception("Number of attributes in instance (" + instance.numAttributes() + ") does not match expected (" + numAttributes + ").");
}
// get the bmu for the instance
CodebookVector bmu = model.getBmu(instance);
double[] classDistribution = new double[numClasses];
// there is no class distribution, only the predicted class
if (useVoting) {
// return the class distribution
int[] distribution = bmu.getClassHitDistribution();
int total = 0;
// calculate the total hits
for (int i = 0; i < distribution.length; i++) {
total += distribution[i];
}
// calculate percentages for each class
for (int i = 0; i < classDistribution.length; i++) {
classDistribution[i] = ((double) distribution[i] / (double) total);
}
}
else {
int index = (int) bmu.getClassification();
classDistribution[index] = 1.0;
}
return classDistribution;
}
/**
* Returns the Capabilities of this classifier.
*
* @return the capabilities of this object
* @see Capabilities
*/
@Override
public Capabilities getCapabilities() {
Capabilities result = super.getCapabilities();
result.disableAll();
// attributes
result.enable(Capability.NUMERIC_ATTRIBUTES);
result.enable(Capability.DATE_ATTRIBUTES);
result.enable(Capability.NOMINAL_ATTRIBUTES);
// class
result.enable(Capability.NOMINAL_CLASS);
result.enable(Capability.MISSING_CLASS_VALUES);
result.setMinimumNumberInstances(1);
return result;
}
/**
* Verify the dataset can be used with the LVQ algorithm and store details about
* the nature of the data.
* Rules:
*
* - Class must be assigned
* - Class must be nominal
* - Must be atleast 1 training instance
* - Must have attributes besides the class attribute
*
*
* @param instances - training dataset
* @return - all instances that can be used for training
* @throws Exception
*/
protected Instances prepareDataset(final Instances instances)
throws Exception {
Instances trainingInstances = new Instances(instances);
trainingInstances.deleteWithMissingClass();
getCapabilities().testWithFail(trainingInstances);
numClasses = trainingInstances.numClasses();
numAttributes = trainingInstances.numAttributes();
// return training instances
return trainingInstances;
}
/**
* Responsible for calculating the class distribution of nodes in the provided model
*
* @param context - context of the distribution (descriptiuon)
* @param distribution - a calculated distribution of each class
* @param aModel - model to evaluate
* @return
*/
public String prepareClassDistributionReport(String aHeader) {
NumberFormat formatter = NumberFormat.getPercentInstance();
StringBuffer buffer = new StringBuffer(200);
// calculate distribution
int[] distribution = model.calculateCodebookClassDistribution();
buffer.append(aHeader + "\n");
for (int i = 0; i < distribution.length; i++) {
int count = distribution[i];
double percentage = ((double) count / (double) model.getTotalCodebookVectors());
buffer.append(model.getClassLabelIndex(i) + " : " + count + " (" + formatter.format(percentage) + ")\n");
}
return buffer.toString();
}
public String prepareIndividualClassDistributionReport() {
NumberFormat format = NumberFormat.getPercentInstance();
StringBuffer buffer = new StringBuffer(1024);
buffer.append("-- Individual BMU Class Distribution --\n");
buffer.append("bmu,\t");
for (int i = 0; i < numClasses; i++) {
buffer.append(i);
buffer.append(",\t");
}
buffer.append("class,\tchanged\n");
CodebookVector[] vectors = model.getCodebookVectors();
for (int i = 0; i < vectors.length; i++) {
int[] distribution = vectors[i].getClassHitDistribution();
int total = 0;
// build a total
for (int j = 0; j < distribution.length; j++) {
total += distribution[j];
}
buffer.append(" ");
buffer.append(i);
buffer.append(",\t");
for (int j = 0; j < distribution.length; j++) {
// calculate percentage
double percentage = 0.0;
if (total > 0) {
percentage = (double) distribution[j] / (double) total;
}
if ((double) j == vectors[i].getClassification()) {
buffer.append("(" + format.format(percentage) + ")");
buffer.append(",\t");
}
else {
buffer.append(" " + format.format(percentage));
buffer.append(", \t");
}
}
buffer.append(Math.round(vectors[i].getClassification()));
buffer.append(",\t");
if (vectors[i].hasClassChanged()) {
buffer.append(vectors[i].hasClassChanged());
}
buffer.append("\n");
}
return buffer.toString();
}
public String prepareTrainingBMUReport() {
NumberFormat format = NumberFormat.getPercentInstance();
StringBuffer buffer = new StringBuffer(1024);
int totalUnused = 0;
// produce a bmu hits report
buffer.append("-- Training BMU Hits Report --\n");
buffer.append("bmu,\t%right,\t%wrong,\t%total,\tunused\n");
for (int i = 0; i < trainingBmuUsage.length; i++) {
int bmuTotal = (trainingBmuUsage[i][0] + trainingBmuUsage[i][1]);
double percentCorrect = 0.0;
double percentError = 0.0;
double percentTotal = 0.0;
if (bmuTotal == 0) {
totalUnused++;
}
else {
percentCorrect = (double) trainingBmuUsage[i][0] / (double) bmuTotal;
percentError = (double) trainingBmuUsage[i][1] / (double) bmuTotal;
percentTotal = (double) bmuTotal / (double) totalTrainingBmuHits;
}
buffer.append(i);
buffer.append(",\t");
buffer.append(format.format(percentCorrect));
buffer.append(",\t");
buffer.append(format.format(percentError));
buffer.append(",\t");
buffer.append(format.format(percentTotal));
buffer.append(",\t");
if (bmuTotal == 0) {
buffer.append("true");
}
buffer.append("\n");
}
buffer.append("Total unused vectors: " + totalUnused + "\n");
return buffer.toString();
}
public String prepareCodebookVectorReport() {
StringBuffer buffer = new StringBuffer(1024);
CodebookVector[] vectors = model.getCodebookVectors();
buffer.append("-- Codebook Vectors (" + vectors.length + " in total) --\n");
for (int i = 0; i < vectors.length; i++) {
// vector attributes and class label
buffer.append(vectors[i].toString());
buffer.append("\n");
}
return buffer.toString();
}
public String prepareBuildTimeReport() {
StringBuffer buffer = new StringBuffer(1024);
buffer.append("-- Training Time Breakdown --\n");
buffer.append("Model Initialisation Time : " + initialisationTime + "ms\n");
buffer.append("Model Training Time : " + trainingTime + "ms\n");
buffer.append("Total Model Preparation Time: " + (initialisationTime + trainingTime) + "ms\n");
return buffer.toString();
}
public String quantisationErrorReport() {
StringBuffer buffer = new StringBuffer(1024);
buffer.append("-- Training Quantisation Error Report --\n");
buffer.append("Quantisation Error : " + trainingQuantisationError + "\n");
buffer.append("Average Quantisation Error : " + trainingAvgQuantisationError + "\n");
return buffer.toString();
}
public double calculateQuantisationError(Instances instances) {
return model.calculateQuantisationError(instances);
}
public String toString() {
StringBuffer buffer = new StringBuffer();
if (super.m_Debug) {
// bmu hits report
if (prepareBmuStatistis) {
buffer.append(prepareTrainingBMUReport());
buffer.append("\n");
}
// class distributions for each codebook vector
buffer.append(prepareIndividualClassDistributionReport());
buffer.append("\n");
// quantisation error
buffer.append(quantisationErrorReport());
buffer.append("\n");
// codebook vectors
buffer.append(prepareCodebookVectorReport());
buffer.append("\n");
}
// build times
buffer.append(prepareBuildTimeReport());
buffer.append("\n");
// distribution report
buffer.append(prepareClassDistributionReport("-- Cass Distribution --"));
buffer.append("\n");
return buffer.toString();
}
/**
* Set the initialisation mode
*
* @param s
*/
public void setInitialisationMode(SelectedTag s) {
if (s.getTags() == InitialisationFactory.TAGS_MODEL_INITALISATION) {
initialisationMode = s.getSelectedTag().getID();
}
}
/**
* Return the initialisation mode
*
* @return
*/
public SelectedTag getInitialisationMode() {
return new SelectedTag(initialisationMode, InitialisationFactory.TAGS_MODEL_INITALISATION);
}
/**
* @return
*/
public int getTotalTrainingBmuHits() {
return totalTrainingBmuHits;
}
/**
* @return
*/
public int[][] getTrainingBmuUsage() {
return trainingBmuUsage;
}
/**
* @param l
*/
public void setSeed(long l) {
seed = l;
}
/**
* @return
*/
public long getSeed() {
return seed;
}
/**
* @return
*/
public boolean getUseVoting() {
return useVoting;
}
/**
* @param b
*/
public void setUseVoting(boolean b) {
useVoting = b;
}
/**
* @param b
*/
public void setPrepareBmuStatistis(boolean b) {
prepareBmuStatistis = b;
}
}
© 2015 - 2024 Weber Informatics LLC | Privacy Policy