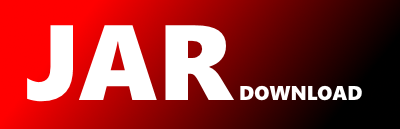
weka.classifiers.neural.lvq.HierarchalLvq Maven / Gradle / Ivy
Go to download
Fork of the following defunct sourceforge.net project: https://sourceforge.net/projects/wekaclassalgos/
The newest version!
Please wait ...