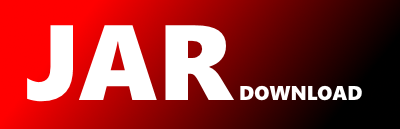
weka.classifiers.neural.lvq.MultipassSom Maven / Gradle / Ivy
Go to download
Show more of this group Show more artifacts with this name
Show all versions of wekaclassalgos Show documentation
Show all versions of wekaclassalgos Show documentation
Fork of the following defunct sourceforge.net project: https://sourceforge.net/projects/wekaclassalgos/
/*
* This program is free software: you can redistribute it and/or modify
* it under the terms of the GNU General Public License as published by
* the Free Software Foundation, either version 3 of the License, or
* (at your option) any later version.
*
* This program is distributed in the hope that it will be useful,
* but WITHOUT ANY WARRANTY; without even the implied warranty of
* MERCHANTABILITY or FITNESS FOR A PARTICULAR PURPOSE. See the
* GNU General Public License for more details.
*
* You should have received a copy of the GNU General Public License
* along with this program. If not, see .
*/
package weka.classifiers.neural.lvq;
import weka.classifiers.AbstractClassifier;
import weka.classifiers.Classifier;
import weka.classifiers.neural.lvq.model.CommonModel;
import weka.core.Instance;
import weka.core.Instances;
import weka.core.Option;
import weka.core.Utils;
import weka.core.WeightedInstancesHandler;
import java.util.Enumeration;
import java.util.LinkedList;
import java.util.Vector;
/**
* Date: 26/05/2004
* File: MultipassLvq2.java
*
* @author Jason Brownlee
*/
public class MultipassSom extends AbstractClassifier
implements WeightedInstancesHandler {
private final static int PARAM_CLASSIFIER_1 = 0;
private final static int PARAM_CLASSIFIER_2 = 1;
private final static String[] PARAMETERS =
{
"A", // pass 1
"B", // pass 2
};
private final static String[] PARAMETER_NOTES =
{
"", // pass 1
"", // pass 2
};
private final static String[] PARAM_DESCRIPTIONS =
{
"SOM algorithm and parameters used for the first pass. Short number of training iterations, large neighbourhood and large learning rate. "
+ "Typically this pass is 50 times the number of codebook vectors, and is unsupervised",
"SOM algorithm and parameters used for the second pass. "
+ "Fine tuning pass with larger number of training iterations (10 times the first pass is typical), smaller neighbourhood and smaller learning rate. Typically this pass is supervised."
};
private final static int TOTAL_PASSES = 2;
protected Som[] algorithms;
protected long[] trainingTimes;
protected boolean modelIsInitialised;
public MultipassSom() {
algorithms = new Som[TOTAL_PASSES];
trainingTimes = new long[TOTAL_PASSES];
algorithms[0] = new Som();
int total = (algorithms[0].getMapWidth() * algorithms[0].getMapHeight());
algorithms[0].setTrainingIterations(total * 20);
algorithms[0].setLearningRate(0.3);
algorithms[0].setSupervised(false);
algorithms[1] = new Som();
algorithms[1].setTrainingIterations(algorithms[0].getTrainingIterations() * 10);
algorithms[1].setLearningRate(0.05);
algorithms[1].setSupervised(false);
}
public void buildClassifier(Instances instances)
throws Exception {
// validate user provided arguments
validateAlgorithmArguments();
// construct and train each model
for (int i = 0; i < algorithms.length; i++) {
trainingTimes[i] = System.currentTimeMillis();
// check for first pass
if (i == 0) {
// build the model
algorithms[i].setPrepareBmuStatistis(false); // not used
}
// not the first pass
else {
// pre-initialise the algorithm
algorithms[i].setPreInitialisedModel(algorithms[0].getModel());
// check for last pass
if (i == algorithms.length - 1) {
// want to use statistics from the last pass
algorithms[i].setPrepareBmuStatistis(true);
}
}
// common
algorithms[i].setDebug(m_Debug);
algorithms[i].buildClassifier(instances);
trainingTimes[i] = (System.currentTimeMillis() - trainingTimes[i]);
}
}
public double[] distributionForInstance(Instance instance)
throws Exception {
// use the first algorithm to classify the instance
// all algorithms use the same underlying model to classify at this point
return algorithms[0].distributionForInstance(instance);
}
public String globalInfo() {
StringBuffer buffer = new StringBuffer(100);
buffer.append("Self Organising Map - Multipass SOM, where the same underlying model is tuned by two SOM algorithms. ");
buffer.append("The is the recommended usage for LVQ-SOM as described by Kohonoen. ");
buffer.append("The same model is used, only it is constructed by passing through two ");
buffer.append("SOM algorithms. It is recommended that the first pass is rough (larger learning rate), ");
buffer.append("and that the second pass is used to fine tune the model (smaller learning rate and longer training time and smaller neighbourhood size).\n\n");
buffer.append("It is important to understand that the first pass will construct the model ");
buffer.append("that is fine tuned by the second pass. This means that algorithm parameters ");
buffer.append("in the second pass used to initialise the model will not be used. ");
buffer.append("These include: initialisation mode, width, height, topology and use voting.");
return buffer.toString();
}
public String toString() {
// all stats are pulled about the model from the last pass (their all valid)
StringBuffer buffer = new StringBuffer();
AlgorithmAncestor lastPass = (AlgorithmAncestor) algorithms[algorithms.length - 1];
if (super.m_Debug) {
// bmu hits report
if (lastPass.prepareBmuStatistis) {
buffer.append(lastPass.prepareTrainingBMUReport());
buffer.append("\n");
}
// class distributions for each codebook vector
buffer.append(lastPass.prepareIndividualClassDistributionReport());
buffer.append("\n");
// quantisation error
buffer.append(lastPass.quantisationErrorReport());
buffer.append("\n");
// codebook vectors
buffer.append(lastPass.prepareCodebookVectorReport());
buffer.append("\n");
}
// build times
buffer.append(prepareTrainingTimeReport());
buffer.append("\n");
// distribution report
buffer.append(lastPass.prepareClassDistributionReport("-- Cass Distribution --"));
buffer.append("\n");
return buffer.toString();
}
public String prepareTrainingTimeReport() {
StringBuffer buffer = new StringBuffer(1024);
buffer.append("-- Training Time Breakdown --\n");
long total = 0;
for (int i = 0; i < trainingTimes.length; i++) {
buffer.append("Pass " + i + ": " + trainingTimes[i] + "ms\n");
total += trainingTimes[i];
}
buffer.append("Total Model Preparation Time: " + total + "ms\n");
return buffer.toString();
}
protected void validateAlgorithmArguments()
throws Exception {
for (int i = 0; i < algorithms.length; i++) {
if (algorithms[i] == null) {
throw new Exception("Algorithm for pass " + (i + 1) + " was not defined.");
}
}
}
public Enumeration listOptions() {
Vector list = new Vector(PARAMETERS.length);
for (int i = 0; i < PARAMETERS.length; i++) {
String param = "-" + PARAMETERS[i] + " " + PARAMETER_NOTES[i];
list.add(new Option("\t" + PARAM_DESCRIPTIONS[i], PARAMETERS[i], 1, param));
}
return list.elements();
}
public void setOptions(String[] options)
throws Exception {
for (int i = 0; i < PARAMETERS.length; i++) {
String data = Utils.getOption(PARAMETERS[i].charAt(0), options);
if (data == null || data.length() == 0) {
continue;
}
switch (i) {
case PARAM_CLASSIFIER_1: {
setPass1SOMClassifier(prepareClassifierFromParameterString(data));
break;
}
case PARAM_CLASSIFIER_2: {
setPass2SOMClassifier(prepareClassifierFromParameterString(data));
break;
}
default: {
throw new Exception("Invalid option offset: " + i);
}
}
}
}
private Classifier prepareClassifierFromParameterString(String s)
throws Exception {
String[] classifierSpec = null;
String classifierName = null;
// split the string into its componenets
classifierSpec = Utils.splitOptions(s);
// verify some componets were specified
if (classifierSpec.length == 0) {
throw new Exception("Invalid classifier specification string");
}
// copy the name, then clear it from the list (it will not be a valid param for itself)
classifierName = classifierSpec[0];
classifierSpec[0] = "";
// consrtuct the classifier with its params
return AbstractClassifier.forName(classifierName, classifierSpec);
}
public String[] getOptions() {
LinkedList list = new LinkedList();
list.add("-" + PARAMETERS[PARAM_CLASSIFIER_1]);
list.add(getClassifierSpec(algorithms[0]));
list.add("-" + PARAMETERS[PARAM_CLASSIFIER_2]);
list.add(getClassifierSpec(algorithms[1]));
return (String[]) list.toArray(new String[list.size()]);
}
protected String getClassifierSpec(Classifier c) {
String name = c.getClass().getName();
String params = Utils.joinOptions(((Som) c).getOptions());
return name + " " + params;
}
public String pass1SOMClassifierTipText() {
return PARAM_DESCRIPTIONS[PARAM_CLASSIFIER_1];
}
public String pass2SOMClassifierTipText() {
return PARAM_DESCRIPTIONS[PARAM_CLASSIFIER_2];
}
public void setPass1SOMClassifier(Classifier c) {
if (!(c instanceof Som)) {
throw new IllegalArgumentException("Only single pass Som algorithms can be specified.");
}
algorithms[0] = (Som) c;
}
public Classifier getPass1SOMClassifier() {
return algorithms[0];
}
public void setPass2SOMClassifier(Classifier c) {
if (!(c instanceof Som)) {
throw new IllegalArgumentException("Only single pass Som algorithms can be specified.");
}
algorithms[1] = (Som) c;
}
public Classifier getPass2SOMClassifier() {
return algorithms[1];
}
public CommonModel getModel() {
return algorithms[0].getModel();
}
public static void main(String[] args) {
runClassifier(new MultipassSom(), args);
}
}
© 2015 - 2024 Weber Informatics LLC | Privacy Policy