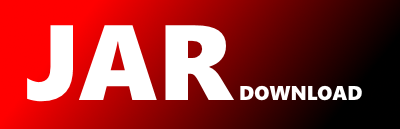
weka.classifiers.neural.lvq.initialise.RandomEven Maven / Gradle / Ivy
Go to download
Show more of this group Show more artifacts with this name
Show all versions of wekaclassalgos Show documentation
Show all versions of wekaclassalgos Show documentation
Fork of the following defunct sourceforge.net project: https://sourceforge.net/projects/wekaclassalgos/
/*
* This program is free software: you can redistribute it and/or modify
* it under the terms of the GNU General Public License as published by
* the Free Software Foundation, either version 3 of the License, or
* (at your option) any later version.
*
* This program is distributed in the hope that it will be useful,
* but WITHOUT ANY WARRANTY; without even the implied warranty of
* MERCHANTABILITY or FITNESS FOR A PARTICULAR PURPOSE. See the
* GNU General Public License for more details.
*
* You should have received a copy of the GNU General Public License
* along with this program. If not, see .
*/
package weka.classifiers.neural.lvq.initialise;
import weka.classifiers.neural.common.RandomWrapper;
import weka.core.Instance;
import weka.core.Instances;
import java.util.LinkedList;
/**
* Date: 25/05/2004
* File: RandomEven.java
*
* @author Jason Brownlee
*/
public class RandomEven extends CommonInitialiser {
protected final LinkedList[] trainingDataClassDistribution;
protected int roundRobbinIndex;
private boolean fullCircle;
public RandomEven(RandomWrapper aRand, Instances aInstances) {
super(aRand, aInstances);
trainingDataClassDistribution = new LinkedList[numClasses];
prepareTrainingDataClassDistributions();
}
protected void prepareTrainingDataClassDistributions() {
for (int i = 0; i < trainingInstances.numInstances(); i++) {
int classIndex = (int) trainingInstances.instance(i).classValue();
if (trainingDataClassDistribution[classIndex] == null) {
trainingDataClassDistribution[classIndex] = new LinkedList();
}
trainingDataClassDistribution[classIndex].add(trainingInstances.instance(i));
}
}
public double[] getAttributes() {
// select an instance
Instance instance = selectInstance();
// construct a codebook vector from the selected instance
double[] attributes = instance.toDoubleArray();
return attributes;
}
protected Instance selectInstance() {
Instance selectedInstance = null;
int startRoundRobbinValue = roundRobbinIndex;
while (selectedInstance == null && !fullCircle) {
// check for an empty class on the current round robbin
if (trainingDataClassDistribution[roundRobbinIndex] == null) {
incrementRoundRobbin();
// check for full circle
if (roundRobbinIndex == startRoundRobbinValue) {
fullCircle = true;
}
}
// check if all vectors from the current class have been included in the model
else if (trainingDataClassDistribution[roundRobbinIndex].isEmpty()) {
incrementRoundRobbin();
// check for full circle
if (roundRobbinIndex == startRoundRobbinValue) {
fullCircle = true;
}
}
// the current selection can be used
else {
int selection = makeRandomSelection(trainingDataClassDistribution[roundRobbinIndex].size());
selectedInstance = (Instance) trainingDataClassDistribution[roundRobbinIndex].remove(selection);
incrementRoundRobbin();
}
}
if (fullCircle) {
// select any random instance
int selection = makeRandomSelection(totalInstances);
selectedInstance = trainingInstances.instance(selection);
}
return selectedInstance;
}
protected void incrementRoundRobbin() {
if (++roundRobbinIndex >= numClasses) {
roundRobbinIndex = 0;
}
}
}
© 2015 - 2024 Weber Informatics LLC | Privacy Policy