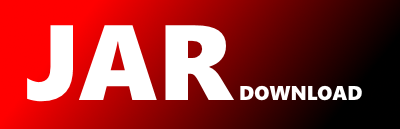
weka.classifiers.neural.lvq.model.CommonModel Maven / Gradle / Ivy
Go to download
Show more of this group Show more artifacts with this name
Show all versions of wekaclassalgos Show documentation
Show all versions of wekaclassalgos Show documentation
Fork of the following defunct sourceforge.net project: https://sourceforge.net/projects/wekaclassalgos/
/*
* This program is free software: you can redistribute it and/or modify
* it under the terms of the GNU General Public License as published by
* the Free Software Foundation, either version 3 of the License, or
* (at your option) any later version.
*
* This program is distributed in the hope that it will be useful,
* but WITHOUT ANY WARRANTY; without even the implied warranty of
* MERCHANTABILITY or FITNESS FOR A PARTICULAR PURPOSE. See the
* GNU General Public License for more details.
*
* You should have received a copy of the GNU General Public License
* along with this program. If not, see .
*/
package weka.classifiers.neural.lvq.model;
import weka.classifiers.neural.lvq.initialise.ModelInitialiser;
import weka.classifiers.neural.lvq.vectordistance.AttributeDistance;
import weka.classifiers.neural.lvq.vectordistance.DistanceFactory;
import weka.core.Attribute;
import weka.core.Instance;
import weka.core.Instances;
import java.io.Serializable;
/**
* Date: 25/05/2004
* File: CommonModel.java
*
* @author Jason Brownlee
*/
public class CommonModel implements Serializable {
protected final CodebookVector[] codebookCollection;
protected String[] classLabels;
protected AttributeDistance[] distanceMeasures;
/**
* @param totalVectors
*/
public CommonModel(int totalVectors) {
codebookCollection = new CodebookVector[totalVectors];
for (int i = 0; i < codebookCollection.length; i++) {
codebookCollection[i] = new CodebookVector(i);
}
}
public void updateModel(ModelUpdater aModelUpdator) {
for (int i = 0; i < codebookCollection.length; i++) {
aModelUpdator.updateCodebookVector(codebookCollection[i]);
}
}
public void initialiseModel(ModelInitialiser aModelInitialiser) {
distanceMeasures = aModelInitialiser.getAttributeDistanceList();
classLabels = aModelInitialiser.getClassLables();
for (int i = 0; i < codebookCollection.length; i++) {
aModelInitialiser.initialiseCodebookVector(codebookCollection[i]);
}
}
public void applyLearningRateToAllVectors(double aLearningRate) {
for (int i = 0; i < codebookCollection.length; i++) {
codebookCollection[i].setIndividualLearningRate(aLearningRate);
}
}
/**
* Calculates the codebook vector class distributeion - that is
* the distribution of classes that codebook vectors are currently assigned to
*
* @return
*/
public int[] calculateCodebookClassDistribution() {
int[] counter = new int[classLabels.length];
// count number of codebook vectors allocated to each class
for (int i = 0; i < codebookCollection.length; i++) {
double classification = codebookCollection[i].getClassification();
int index = (int) Math.floor(classification);
counter[index]++;
}
return counter;
}
/**
* Caches a list of all known class labels
*
* @param classAttribute
*/
protected void cacheKnownClasses(Attribute classAttribute) {
classLabels = new String[classAttribute.numValues()];
// cache each class double value at its index
for (int i = 0; i < classLabels.length; i++) {
classLabels[i] = classAttribute.value(i);
}
}
/**
* Classifies the provided data instance
*
* @param aInstance
* @return
*/
public double classifyInstance(Instance aInstance) {
// return the distribution of the BMU
return getBmu(aInstance).getClassification();
}
/**
* Returns the class label for the provided class value/type index
*
* @param i
* @return
*/
public String getClassLabelIndex(int i) {
return classLabels[i];
}
/**
* Calculates the distance between the provided data instance and a code
* book vector. Uses attribute specific distance measures.
*
* @param instance
* @param codebookVector
* @param classIndex
* @return
*/
protected double distance(double[] instance,
double[] codebookVector,
double aBestValue) {
return DistanceFactory.calculateDistance(distanceMeasures, instance, codebookVector, aBestValue);
}
/**
* Returns the best matching unit (codebook vecotr) for a given data instance.
* A distance measure from the instance to each codebook vector is calculated. The lowest
* distance measure becomes the best matching unit (BMU)
*
* @param aInstance - a dat instance
* @return
*/
public CodebookVector getBmu(Instance aInstance) {
double[] instance = aInstance.toDoubleArray();
int bestIndex = 0;
double bestDistance = distance(instance, codebookCollection[bestIndex].getAttributes(), Double.POSITIVE_INFINITY);
// process all codebook vectors
for (int i = 1; i < codebookCollection.length; i++) {
double distance = distance(instance, codebookCollection[i].getAttributes(), bestDistance);
// check for new bmu
if (distance < bestDistance) {
bestDistance = distance;
bestIndex = i;
}
}
codebookCollection[bestIndex].setBmuHit(bestDistance, aInstance);
return codebookCollection[bestIndex];
}
public double getBmuDistance(Instance aInstance) {
double[] instance = aInstance.toDoubleArray();
double bestDistance = distance(instance, codebookCollection[0].getAttributes(), Double.MAX_VALUE);
// process all codebook vectors
for (int i = 1; i < codebookCollection.length; i++) {
double distance = distance(instance, codebookCollection[i].getAttributes(), bestDistance);
// check for new bmu
if (distance < bestDistance) {
bestDistance = distance;
}
}
return bestDistance;
}
public int getTotalCodebookVectors() {
if (codebookCollection == null) {
return 0;
}
return codebookCollection.length;
}
public void clearBmuCounts() {
for (int i = 0; i < codebookCollection.length; i++) {
codebookCollection[i].resetBmuCounts();
}
}
public int[][] getBmuCounts() {
int[][] bmuCounts = new int[codebookCollection.length][2];
for (int i = 0; i < codebookCollection.length; i++) {
bmuCounts[i][0] = codebookCollection[i].getBmuCorrectCount();
bmuCounts[i][1] = codebookCollection[i].getBmuIncorrectCount();
}
return bmuCounts;
}
public void setUseVoting(boolean useVoting) {
for (int i = 0; i < codebookCollection.length; i++) {
codebookCollection[i].setUseVoting(useVoting);
}
}
public void clearClassDistributions() {
for (int i = 0; i < codebookCollection.length; i++) {
codebookCollection[i].clearClassDistributions();
}
}
public double calculateQuantisationError(Instances aInstances) {
double quantisationError = 0.0;
for (int i = 0; i < aInstances.numInstances(); i++) {
quantisationError += Math.sqrt(getBmuDistance(aInstances.instance(i)));
}
return quantisationError;
}
/**
* @return
*/
public CodebookVector[] getCodebookVectors() {
return codebookCollection;
}
}
© 2015 - 2024 Weber Informatics LLC | Privacy Policy