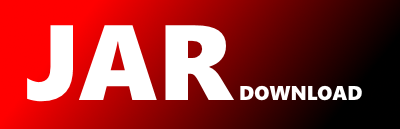
weka.classifiers.neural.multilayerperceptron.algorithm.BackPropagationAlgorithm Maven / Gradle / Ivy
Go to download
Fork of the following defunct sourceforge.net project: https://sourceforge.net/projects/wekaclassalgos/
/*
* This program is free software: you can redistribute it and/or modify
* it under the terms of the GNU General Public License as published by
* the Free Software Foundation, either version 3 of the License, or
* (at your option) any later version.
*
* This program is distributed in the hope that it will be useful,
* but WITHOUT ANY WARRANTY; without even the implied warranty of
* MERCHANTABILITY or FITNESS FOR A PARTICULAR PURPOSE. See the
* GNU General Public License for more details.
*
* You should have received a copy of the GNU General Public License
* along with this program. If not, see .
*/
package weka.classifiers.neural.multilayerperceptron.algorithm;
import weka.classifiers.neural.common.BatchTrainableNeuralModel;
import weka.classifiers.neural.common.CommonNeuralAlgorithmAncestor;
import weka.classifiers.neural.common.RandomWrapper;
import weka.classifiers.neural.common.SimpleNeuron;
import weka.classifiers.neural.common.initialisation.Initialisation;
import weka.classifiers.neural.common.learning.LearningRateKernel;
import weka.classifiers.neural.common.transfer.TransferFunction;
import weka.core.Instance;
import weka.core.Instances;
/**
* Date: 31/05/2004
* File: BackPropagationAlgorithm.java
*
* @author Jason Brownlee
*/
public class BackPropagationAlgorithm extends CommonNeuralAlgorithmAncestor
implements BatchTrainableNeuralModel {
// neurons which make up this model
protected SimpleNeuron[][] neurons;
protected final int numInputs;
// activations
protected final double[][] activations;
// transferred (node outputs)
protected final double[][] outputs;
// derivatives of error in regard to weights
protected final double[][] deltas;
// momentum
protected final double momentum;
// weight decay parameter
protected final double weightDecay;
// learning rate function
protected final LearningRateKernel learningRateFunction;
public BackPropagationAlgorithm(TransferFunction aTransferFunction,
RandomWrapper aRand,
LearningRateKernel aLearningRateKernel,
double aMomentum,
double aWeightDecay,
double aBiasValue,
int[] aHiddenLayersTopology,
Instances aTrainingInstances) {
super(aTransferFunction, aRand);
learningRateFunction = aLearningRateKernel;
momentum = aMomentum;
weightDecay = aWeightDecay;
// prepare the network structure
prepareNetworkStructure(aTrainingInstances, aHiddenLayersTopology, aBiasValue);
// initialise network weights
initialiseNetworkWeights();
// number of inputs
numInputs = aTrainingInstances.numAttributes() - 1;
// allocate memory for activations, outputs and derivatives of error
activations = new double[neurons.length][];
outputs = new double[neurons.length][];
deltas = new double[neurons.length][];
for (int i = 0; i < neurons.length; i++) {
activations[i] = new double[neurons[i].length];
outputs[i] = new double[neurons[i].length];
deltas[i] = new double[neurons[i].length];
}
}
public double[] getAllWeights() {
int totalWeights = 0;
double[] weights;
for (int i = 0; i < neurons.length; i++) {
for (int j = 0; j < neurons[i].length; j++) {
totalWeights += neurons[i][j].getWeights().length;
}
}
weights = new double[totalWeights];
int offset = 0;
for (int i = 0; i < neurons.length; i++) {
for (int j = 0; j < neurons[i].length; j++) {
double[] tmpWeights = neurons[i][j].getWeights();
for (int k = 0; k < tmpWeights.length; k++, offset++) {
weights[offset] = tmpWeights[k];
}
}
}
return weights;
}
public void updateModel(Instance instance, double aLearningRate) {
// calcuate errors
calculateWeightErrors(instance, aLearningRate);
// apply weight changes
applyWeightDeltas(aLearningRate);
}
public int getNumOutputNeurons() {
if (neurons == null) {
return 0;
}
return neurons[neurons.length - 1].length;
}
public void calculateWeightErrors(Instance instance, double aLearningRate) {
// forward propagate based on provided input
forwardPropagate(instance);
// determine the expected output
double[] expectedOutput = prepareExpectedOutputVector(instance);
// perform a backwards pass
backwardPropagate(expectedOutput);
// store errors for each weight
storeDerivativeOfErrors(instance);
}
public void applyWeightDeltas(double aLearningRate) {
// process all layers
for (int i = 0; i < neurons.length; i++) {
// process all nodes in this layer
for (int j = 0; j < neurons[i].length; j++) {
// get the weights and errors for the current neuron
double[] weights = neurons[i][j].getWeights();
double[] dEwE = neurons[i][j].getdEwE();
double[] weightDeltas = neurons[i][j].getLastWeightDeltas();
for (int k = 0; k < weights.length; k++) {
// calculate change in weight
weightDeltas[k] = calculateWeightDelta(dEwE[k], weightDeltas[k], weights[k], aLearningRate);
// apply change in weight
weights[k] += weightDeltas[k];
// clear error signals
dEwE[k] = 0.0;
}
}
}
}
protected double calculateWeightDelta(double weightError,
double lastDelta,
double currentWeight,
double aLearningRate) {
// w(t+1) = w(t) + (lrate * error) + (momentum * lastWeightChange) - (weight decay * current weight)
return (aLearningRate * weightError) +
(momentum * lastDelta) -
(weightDecay * currentWeight);
}
public double getLearningRate(int epochNumber) {
return learningRateFunction.currentLearningRate(epochNumber);
}
public void startingEpoch() {
}
public void finishedEpoch(Instances instances, double aLearningRate) {
}
protected void backwardPropagate(double[] expectedOutput) {
// calculate the node deltas
int layer = (outputs.length - 1);
// calculate derivatives of error in regard to weight for the output layer
for (int j = 0; j < outputs[layer].length; j++) {
// calculate error (expected output - actual output)
double error = (expectedOutput[j] - outputs[layer][j]);
// store the delta (error * derivative of output)
deltas[layer][j] = error * derivative(activations[layer][j], outputs[layer][j]);
}
// calculate error for all hidden layers
for (layer = (outputs.length - 2); layer >= 0; layer--) {
// process all nodes on the current layer
for (int j = 0; j < outputs[layer].length; j++) {
// now step forward one layer and pull back the error values
// through the weighted connection to the node we are working with [j]
// this means all nodes in the next layer that take input from j's output
// will have their error signal * weight from j's output added together
double sum = 0.0;
// for all nodes on the next layer forward
for (int k = 0; k < deltas[layer + 1].length; k++) {
// get the weights for the k'th node
double[] kw = neurons[layer + 1][k].getWeights();
// add the k'ths error signal by the weighting from j's output
sum += (kw[j] * deltas[layer + 1][k]);
}
// calculate the delta (weighed sum of deltas * derivative of output)
deltas[layer][j] = sum * derivative(activations[layer][j], outputs[layer][j]);
}
}
}
protected void storeDerivativeOfErrors(Instance instance) {
double[] input = instance.toDoubleArray();
int layers = 0;
// calculate errors for the first layer
for (int i = 0; i < neurons[layers].length; i++) {
// get accumulated error vector
double[] dEwE = neurons[layers][i].getdEwE();
int offset = 0;
// calculate the derivative of error in regard to each weight in the current node
for (int j = 0; j < input.length; j++) {
// ensure not the class variable
if (instance.classIndex() != j) {
// ensure not missing
if (instance.isMissing(j)) {
offset++; // skip over the derivative of error - no value
}
else {
// calculate and store (accumulate)
// delta for node * input value
dEwE[offset++] += deltas[layers][i] * input[j];
}
}
}
// calculate error for bias weight
int biasIndex = neurons[layers][i].getBiasIndex();
dEwE[biasIndex] += deltas[layers][i] * neurons[layers][i].getBiasInputValue();
}
// calculate errors for all remaining hidden layers
for (layers = 1; layers < neurons.length; layers++) {
// process all nodes for current layer
for (int i = 0; i < neurons[layers].length; i++) {
// get accumulated error vector for current node
double[] dEwE = neurons[layers][i].getdEwE();
// calculate the derivative of error in regard to each weight in the current node
for (int j = 0; j < neurons[layers - 1].length; j++) {
// delta for current node [i] * input received along that weighting
dEwE[j] += deltas[layers][i] * outputs[layers - 1][j];
}
// calculate error for bias weight
int biasIndex = neurons[layers][i].getBiasIndex();
dEwE[biasIndex] += deltas[layers][i] * neurons[layers][i].getBiasInputValue();
}
}
}
protected void forwardPropagate(Instance instance) {
// calculate network output for a given input
int layer = 0;
// calculate output for the first hidden layer
for (int j = 0; j < activations[layer].length; j++) {
// calculate activation
activations[layer][j] = activate(neurons[layer][j], instance);
// calculate output
outputs[layer][j] = transfer(activations[layer][j]);
}
// calculate outputs for all Hidden layers
for (layer = 1; layer < activations.length; layer++) {
for (int j = 0; j < activations[layer].length; j++) {
// calculate activation using the prvious layer's output as input
activations[layer][j] = activate(neurons[layer][j], outputs[layer - 1]);
// calculate output
outputs[layer][j] = transfer(activations[layer][j]);
}
}
}
public double[] getNetworkOutputs(Instance instance) {
// forward propagate based on provided input
forwardPropagate(instance);
double[] output = new double[outputs[outputs.length - 1].length];
// copy the output layers values
for (int i = 0; i < output.length; i++) {
output[i] = outputs[outputs.length - 1][i];
}
return output;
}
public String getModelInformation() {
StringBuffer buffer = new StringBuffer();
int count = 0;
buffer.append("Momentum: " + momentum + "\n");
buffer.append("Weight Decay: " + weightDecay + "\n");
buffer.append("Bias Input Value: " + neurons[0][0].getBiasInputValue() + "\n");
buffer.append("\n");
buffer.append("Num Inputs: " + numInputs + "\n");
for (int i = 0; i < neurons.length - 1; i++) {
buffer.append("Hidden Layer " + (i + 1) + ": " + neurons[i].length + "\n");
count += neurons[i].length;
}
buffer.append("Output Layer: " + neurons[neurons.length - 1].length + "\n");
count += neurons[neurons.length - 1].length;
buffer.append("Total Neurons: " + count + "\n");
return buffer.toString();
}
protected void prepareNetworkStructure(Instances instances,
int[] hiddenLayersTopology,
double aBiasValue) {
int numAttributes = 0;
if (hiddenLayersTopology != null) {
// allocate enough memory for the configured number of layers
neurons = new SimpleNeuron[hiddenLayersTopology.length + 1][];
// construct all hidden layers
for (int i = 0; i < neurons.length - 1; i++) {
// allocate memory for layer
neurons[i] = new SimpleNeuron[hiddenLayersTopology[i]];
// determine the number of input attribute for neurons on this layer
if (i == 0) {
numAttributes = instances.numAttributes() - 1; // input layer
}
else {
// number of inputs is the number of neurons on previous layer
numAttributes = hiddenLayersTopology[i - 1];
}
// construct the neurons for this layer
for (int j = 0; j < neurons[i].length; j++) {
neurons[i][j] = new SimpleNeuron(numAttributes, aBiasValue);
}
}
}
else {
// allocate enough memory for the configured number of layers
neurons = new SimpleNeuron[1][];
}
// determine the number of neurons required for output layer
if (instances.classAttribute().isNumeric()) {
neurons[neurons.length - 1] = new SimpleNeuron[1]; // regression has a single output
}
// must be nominal
else {
// the number of classes
neurons[neurons.length - 1] = new SimpleNeuron[instances.numClasses()];
}
// determine the number of inputs for output layer
if (neurons.length - 1 == 0) {
numAttributes = instances.numAttributes() - 1; // input layer
}
else {
// number of inputs is the number of neurons on previous layer
numAttributes = hiddenLayersTopology[hiddenLayersTopology.length - 1];
}
// construct the output layer
for (int i = 0; i < neurons[neurons.length - 1].length; i++) {
neurons[neurons.length - 1][i] = new SimpleNeuron(numAttributes, aBiasValue);
}
}
protected void initialiseNetworkWeights() {
for (int i = 0; i < neurons.length; i++) {
for (int j = 0; j < neurons[i].length; j++) {
// initialise weights to between -0.5 and +0.5
Initialisation.initialiseVectorToRandomWithSign(neurons[i][j].getWeights(), 0.5, 0.0, rand);
}
}
}
}
© 2015 - 2025 Weber Informatics LLC | Privacy Policy