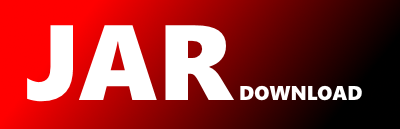
weka.classifiers.neural.singlelayerperceptron.algorithm.SLPAlgorithmAncestor Maven / Gradle / Ivy
Go to download
Show more of this group Show more artifacts with this name
Show all versions of wekaclassalgos Show documentation
Show all versions of wekaclassalgos Show documentation
Fork of the following defunct sourceforge.net project: https://sourceforge.net/projects/wekaclassalgos/
/*
* This program is free software: you can redistribute it and/or modify
* it under the terms of the GNU General Public License as published by
* the Free Software Foundation, either version 3 of the License, or
* (at your option) any later version.
*
* This program is distributed in the hope that it will be useful,
* but WITHOUT ANY WARRANTY; without even the implied warranty of
* MERCHANTABILITY or FITNESS FOR A PARTICULAR PURPOSE. See the
* GNU General Public License for more details.
*
* You should have received a copy of the GNU General Public License
* along with this program. If not, see .
*/
package weka.classifiers.neural.singlelayerperceptron.algorithm;
import weka.classifiers.neural.common.CommonNeuralAlgorithmAncestor;
import weka.classifiers.neural.common.RandomWrapper;
import weka.classifiers.neural.common.SimpleNeuron;
import weka.classifiers.neural.common.initialisation.Initialisation;
import weka.classifiers.neural.common.learning.LearningRateKernel;
import weka.classifiers.neural.common.transfer.TransferFunction;
import weka.core.Instance;
import weka.core.Instances;
/**
* Title: Weka Neural Implementation
* Description: ...
* Copyright: Copyright (c) 2003
* Company: N/A
*
* @author Jason Brownlee
* @version 1.0
*/
public abstract class SLPAlgorithmAncestor extends CommonNeuralAlgorithmAncestor {
// neurons which make up this model
protected final SimpleNeuron[] neurons;
// learning rate function
protected final LearningRateKernel learningRateFunction;
public SLPAlgorithmAncestor(TransferFunction aTransfer,
double aBiasInput,
RandomWrapper aRand,
LearningRateKernel aKernel,
Instances trainingInstances) {
super(aTransfer, aRand);
learningRateFunction = aKernel;
// determine the number of neurons required
if (trainingInstances.classAttribute().isNumeric()) {
neurons = new SimpleNeuron[1];
}
// must be numeric
else {
neurons = new SimpleNeuron[trainingInstances.numClasses()];
}
// prepare the network structure
prepareNetworkStructure(trainingInstances, aBiasInput);
}
public int getNumOutputNeurons() {
if (neurons == null) {
return 0;
}
return neurons.length;
}
public double[] getAllWeights() {
if (neurons == null) {
return null;
}
int totalWeights = neurons.length * neurons[0].getWeights().length;
double[] weights = new double[totalWeights];
int offset = 0;
for (int i = 0; i < neurons.length; i++) {
double[] tmpWeights = neurons[i].getWeights();
for (int k = 0; k < tmpWeights.length; k++, offset++) {
weights[offset] = tmpWeights[k];
}
}
return weights;
}
protected abstract void calculateWeightErrors(Instance instance, SimpleNeuron neuron, double expected, double aLearningRate);
public double getLearningRate(int aEpochNumber) {
return learningRateFunction.currentLearningRate(aEpochNumber);
}
public void startingEpoch() {
}
public void finishedEpoch(Instances instances, double aLearningRate) {
}
public String getModelInformation() {
StringBuffer buffer = new StringBuffer();
buffer.append("Initial Learing Rate : " + learningRateFunction.getInitialLearningRate() + "\n");
buffer.append("Bias Input Value : " + neurons[0].getBiasInputValue() + "\n");
buffer.append("Output Layer Neurons : " + neurons.length + "\n");
return buffer.toString();
}
public void updateModel(Instance inputs, double aLearningRate) {
// prepare an expected output vector
double[] expected = prepareExpectedOutputVector(inputs);
// calculate weight changes for each neuron
for (int i = 0; i < neurons.length; i++) {
// calculate weight changes
calculateWeightErrors(inputs, neurons[i], expected[i], aLearningRate);
}
}
protected void prepareNetworkStructure(Instances instances, double aBiasInput) {
// determine the number of attribtes
int numAttributes = instances.numAttributes() - 1;
// construct the required number of neurons
for (int i = 0; i < neurons.length; i++) {
neurons[i] = new SimpleNeuron(numAttributes, aBiasInput);
// initialise weights to between -0.5 and +0.5
Initialisation.initialiseVectorToRandomWithSign(neurons[i].getWeights(), 0.5, 0.0, rand);
}
}
public double[] getNetworkOutputs(Instance instance) {
double[] distribution = new double[neurons.length];
for (int i = 0; i < distribution.length; i++) {
double activation = activate(neurons[i], instance);
distribution[i] = transfer(activation);
}
return distribution;
}
}
© 2015 - 2024 Weber Informatics LLC | Privacy Policy