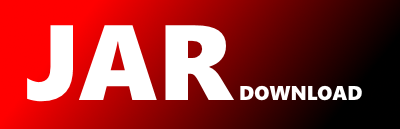
jmaxent.FeatureGen Maven / Gradle / Ivy
Go to download
Show more of this group Show more artifacts with this name
Show all versions of heideltime Show documentation
Show all versions of heideltime Show documentation
HeidelTime is a multilingual cross-domain temporal tagger that extracts temporal expressions from documents and normalizes them according to the TIMEX3 annotation standard.
/*
Copyright (C) 2010 by
*
* Cam-Tu Nguyen
* [email protected] or [email protected]
*
* Xuan-Hieu Phan
* [email protected]
*
* College of Technology, Vietnamese University, Hanoi
* Graduate School of Information Sciences, Tohoku University
*
* JVnTextPro-v.2.0 is a free software; you can redistribute it and/or modify
* it under the terms of the GNU General Public License as published
* by the Free Software Foundation; either version 2 of the License,
* or (at your option) any later version.
*
* JVnTextPro-v.2.0 is distributed in the hope that it will be useful, but
* WITHOUT ANY WARRANTY; without even the implied warranty of
* MERCHANTABILITY or FITNESS FOR A PARTICULAR PURPOSE. See the
* GNU General Public License for more details.
*
* You should have received a copy of the GNU General Public License
* along with JVnTextPro-v.2.0); if not, write to the Free Software Foundation,
* Inc., 59 Temple Place, Suite 330, Boston, MA 02111-1307 USA.
*/
package jmaxent;
import java.io.*;
import java.util.*;
// TODO: Auto-generated Javadoc
/**
* The Class FeatureGen.
*/
public class FeatureGen {
/** The features. */
List features = null; // list of features
/** The fmap. */
Map fmap = null; // feature map
/** The option. */
Option option = null; // option object
/** The data. */
Data data = null; // data object
/** The dict. */
Dictionary dict = null; // dictionary object
// for scan feature only
/** The current features. */
List currentFeatures = null;
/** The current feature idx. */
int currentFeatureIdx = 0;
/**
* Instantiates a new feature gen.
*
* @param option the option
* @param data the data
* @param dict the dict
*/
public FeatureGen(Option option, Data data, Dictionary dict) {
this.option = option;
this.data = data;
this.dict = dict;
}
// adding a feature
/**
* Adds the feature.
*
* @param f the f
*/
public void addFeature(Feature f) {
f.strId2IdxAdd(fmap);
features.add(f);
}
// generating features
/**
* Generate features.
*/
public void generateFeatures() {
if (features != null) {
features.clear();
} else {
features = new ArrayList();
}
if (fmap != null) {
fmap.clear();
} else {
fmap = new HashMap();
}
if (currentFeatures != null) {
currentFeatures.clear();
} else {
currentFeatures = new ArrayList();
}
if (data.trnData == null || dict.dict == null) {
System.out.println("No data or dictionary for generating features");
return;
}
// scan over data list
for (int i = 0; i < data.trnData.size(); i++) {
Observation obsr = (Observation)data.trnData.get(i);
for (int j = 0; j < obsr.cps.length; j++) {
Element elem = null;
CountFIdx cntFIdx = null;
elem = (Element)dict.dict.get(new Integer(obsr.cps[j]));
if (elem != null) {
if (elem.count <= option.cpRareThreshold) {
// skip this context predicate, it is too rare
continue;
}
cntFIdx = (CountFIdx)elem.lbCntFidxes.get(new Integer(obsr.humanLabel));
if (cntFIdx != null) {
if (cntFIdx.count <= option.fRareThreshold) {
// skip this feature, it is too rare
continue;
}
} else {
// not found in the dictionary, then skip
continue;
}
} else {
// not found in the dictionary, then skip
continue;
}
// update the feature
Feature f = new Feature(obsr.humanLabel, obsr.cps[j]);
f.strId2Idx(fmap);
if (f.idx < 0) {
// new feature, add to the feature list
addFeature(f);
// update the feature index in the dictionary
cntFIdx.fidx = f.idx;
elem.chosen = 1;
}
}
}
option.numFeatures = features.size();
}
/**
* Num features.
*
* @return the int
*/
public int numFeatures() {
if (features == null) {
return 0;
} else {
return features.size();
}
}
/**
* Read features.
*
* @param fin the fin
* @throws IOException Signals that an I/O exception has occurred.
*/
public void readFeatures(BufferedReader fin) throws IOException {
if (features != null) {
features.clear();
} else {
features = new ArrayList();
}
if (fmap != null) {
fmap.clear();
} else {
fmap = new HashMap();
}
if (currentFeatures != null) {
currentFeatures.clear();
} else {
currentFeatures = new ArrayList();
}
String line;
// get the number of features
if ((line = fin.readLine()) == null) {
System.out.println("Unknown number of features");
return;
}
int numFeatures = Integer.parseInt(line);
if (numFeatures <= 0) {
System.out.println("Invalid number of features");
return;
}
System.out.println("Reading features ...");
// main loop for reading features
for (int i = 0; i < numFeatures; i++) {
line = fin.readLine();
if (line == null) {
// invalid feature line, ignore it
continue;
}
StringTokenizer strTok = new StringTokenizer(line, " ");
if (strTok.countTokens() != 4) {
System.out.println(i + " invalid feature line ");
// invalid feature line, ignore it
continue;
}
// create a new feature by parsing the line
Feature f = new Feature(line, data.cpStr2Int, data.lbStr2Int);
Integer fidx = (Integer)fmap.get(f.strId);
if (fidx == null) {
// insert the feature into the feature map
fmap.put(f.strId, new Integer(f.idx));
features.add(f);
}
else {
fmap.put(f.strId, new Integer(f.idx));
features.add(f);
}
}
System.out.println("Reading " + Integer.toString(features.size()) + " features completed!");
// read the line ###...
line = fin.readLine();
option.numFeatures = features.size();
}
/**
* Write features.
*
* @param fout the fout
* @throws IOException Signals that an I/O exception has occurred.
*/
public void writeFeatures(PrintWriter fout) throws IOException {
// write the number of features
fout.println(Integer.toString(features.size()));
for (int i = 0; i < features.size(); i++) {
Feature f = (Feature)features.get(i);
fout.println(f.toString(data.cpInt2Str, data.lbInt2Str));
}
// wirte the line ###...
fout.println(Option.modelSeparator);
}
/**
* Scan reset.
*/
public void scanReset() {
currentFeatureIdx = 0;
}
/**
* Start scan features.
*
* @param obsr the obsr
*/
public void startScanFeatures(Observation obsr) {
currentFeatures.clear();
currentFeatureIdx = 0;
// scan over all context predicates
for (int i = 0; i < obsr.cps.length; i++) {
Element elem = (Element)dict.dict.get(new Integer(obsr.cps[i]));
if (elem == null) {//this context predicate doesn't appear in the dictionary of training data
continue;
}
if (!(elem.isScanned)) {
// scan all labels for features
Iterator it = elem.lbCntFidxes.keySet().iterator();
while (it.hasNext()) {
Integer labelInt = (Integer)it.next();
CountFIdx cntFIdx = (CountFIdx)elem.lbCntFidxes.get(labelInt);
if (cntFIdx.fidx >= 0) {
Feature f = new Feature();
f.FeatureInit(labelInt.intValue(), obsr.cps[i]);
f.idx = cntFIdx.fidx;
elem.cpFeatures.add(f);
}
}
elem.isScanned = true;
}
for (int j = 0; j < elem.cpFeatures.size(); j++) {
currentFeatures.add(elem.cpFeatures.get(j));
}
}
}
/**
* Checks for next feature.
*
* @return true, if successful
*/
public boolean hasNextFeature() {
return (currentFeatureIdx < currentFeatures.size());
}
/**
* Next feature.
*
* @return the feature
*/
public Feature nextFeature() {
Feature f = (Feature)currentFeatures.get(currentFeatureIdx);
currentFeatureIdx++;
return f;
}
} // end of class FeatureGen
© 2015 - 2025 Weber Informatics LLC | Privacy Policy