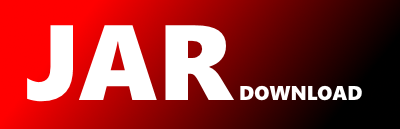
jmaxent.Train Maven / Gradle / Ivy
Go to download
Show more of this group Show more artifacts with this name
Show all versions of heideltime Show documentation
Show all versions of heideltime Show documentation
HeidelTime is a multilingual cross-domain temporal tagger that extracts temporal expressions from documents and normalizes them according to the TIMEX3 annotation standard.
/*
Copyright (C) 2010 by
*
* Cam-Tu Nguyen
* [email protected] or [email protected]
*
* Xuan-Hieu Phan
* [email protected]
*
* College of Technology, Vietnamese University, Hanoi
* Graduate School of Information Sciences, Tohoku University
*
* JVnTextPro-v.2.0 is a free software; you can redistribute it and/or modify
* it under the terms of the GNU General Public License as published
* by the Free Software Foundation; either version 2 of the License,
* or (at your option) any later version.
*
* JVnTextPro-v.2.0 is distributed in the hope that it will be useful, but
* WITHOUT ANY WARRANTY; without even the implied warranty of
* MERCHANTABILITY or FITNESS FOR A PARTICULAR PURPOSE. See the
* GNU General Public License for more details.
*
* You should have received a copy of the GNU General Public License
* along with JVnTextPro-v.2.0); if not, write to the Free Software Foundation,
* Inc., 59 Temple Place, Suite 330, Boston, MA 02111-1307 USA.
*/
package jmaxent;
import java.io.*;
import org.riso.numerical.*;
// TODO: Auto-generated Javadoc
/**
* The Class Train.
*/
public class Train {
// the model object
/** The model. */
public Model model = null;
/** The num labels. */
public int numLabels = 0;
/** The num features. */
public int numFeatures = 0;
/** The lambda. */
double[] lambda = null;
/** The temp lambda. */
double[] tempLambda = null;
// for L-BFGS
/** The grad log li. */
double[] gradLogLi = null;
/** The diag. */
double[] diag = null;
/** The temp. */
double[] temp = null;
/** The ws. */
double[] ws = null;
/** The iprint. */
int[] iprint = null;
/** The iflag. */
int[] iflag = null;
/**
* Instantiates a new train.
*/
public Train() {
// do nothing
}
/**
* Inits the.
*/
public void init() {
numLabels = model.data.numLabels();
numFeatures = model.feaGen.numFeatures();
if (numLabels <= 0 || numFeatures <= 0) {
System.out.println("Invalid number of labels or features");
return;
}
lambda = model.lambda;
tempLambda = new double[numFeatures];
gradLogLi = new double[numFeatures];
diag = new double[numFeatures];
temp = new double[numLabels];
int wsSize = numFeatures * (2 * model.option.mForHessian + 1) +
2 * model.option.mForHessian;
ws = new double[wsSize];
iprint = new int[2];
iflag = new int[1];
}
/**
* Norm.
*
* @param vect the vect
* @return the double
*/
public static double norm(double[] vect) {
double res = 0.0;
for (int i = 0; i < vect.length; i++) {
res += vect[i] * vect[i];
}
return Math.sqrt(res);
}
/**
* Do train.
*
* @param fout the fout
*/
public void doTrain(PrintWriter fout) {
long start_train, end_train, elapsed_train;
long start_iter, end_iter, elapsed_iter;
// initialization
init();
double f = 0.0;
//double old_f;
double xtol = 1.0e-16;
int numIter = 0;
// for L-BFGS
iprint[0] = model.option.debugLevel - 2;
iprint[1] = model.option.debugLevel - 1;
iflag[0] = 0;
// counter
int i;
// get initial values for lambda
for (i = 0; i < numFeatures; i++) {
lambda[i] = model.option.initLambdaVal;
}
System.out.println("Start to train ...");
if (model.option.isLogging) {
model.option.writeOptions(fout);
fout.println("Start to train ...");
}
// starting time of the training process
start_train = System.currentTimeMillis();
double maxAccuracy = 0.0;
int maxAccuracyIter = -1;
// the training loop
do {
// starting time of iteration
start_iter = System.currentTimeMillis();
// call this to compute two things:
// 1. log-likelihood value
// 2. the gradient vector of log-likelihood function
f = computeLogLiGradient(lambda, gradLogLi, numIter + 1, fout);
// negate f and its gradient because L-BFGS minimizes the objective function
// while we would like to maximize it
f *= -1;
for (i = 0; i < numFeatures; i++) {
gradLogLi[i] *= -1;
}
// calling L-BFGS
try {
new LBFGS().lbfgs(numFeatures, model.option.mForHessian, lambda, f, gradLogLi,
false, diag, iprint, model.option.epsForConvergence, xtol, iflag);
} catch (LBFGS.ExceptionWithIflag e) {
System.out.println("L-BFGS failed!");
if (model.option.isLogging) {
fout.println("L-BFGS failed!");
}
break;
}
numIter++;
// get the end time of the current iteration
end_iter = System.currentTimeMillis();
elapsed_iter = end_iter - start_iter;
System.out.println("\tIteration elapsed: " +
Double.toString((double)elapsed_iter / 1000) + " seconds");
if (model.option.isLogging) {
fout.println("\tIteration elapsed: " +
Double.toString((double)elapsed_iter / 1000) + " seconds");
}
// evaluate during training
if (model.option.evaluateDuringTraining) {
// inference on testing data
model.doInference(model.data.tstData);
// evaluation
double accuracy = model.evaluation.evaluate(fout);
if (accuracy > maxAccuracy) {
maxAccuracy = accuracy;
maxAccuracyIter = numIter;
// save the best model towards testing evaluation
if (model.option.saveBestModel) {
for (i = 0; i < numFeatures; i++) {
tempLambda[i] = lambda[i];
}
}
}
System.out.println("\tCurrent max accuracy: " +
Double.toString(maxAccuracy) + " (at iteration " +
Integer.toString(maxAccuracyIter) + ")");
if (model.option.isLogging) {
fout.println("\tCurrent max accuracy: " +
Double.toString(maxAccuracy) + " (at iteration " +
Integer.toString(maxAccuracyIter) + ")");
}
// get the end time of the current iteration
end_iter = System.currentTimeMillis();
elapsed_iter = end_iter - start_iter;
System.out.println("\tIteration elapsed (including testing & evaluation): " +
Double.toString((double)elapsed_iter / 1000) + " seconds");
if (model.option.isLogging) {
fout.println("\tIteration elapsed (including testing & evaluation): " +
Double.toString((double)elapsed_iter / 1000) + " seconds");
fout.flush();
}
}
} while (iflag[0] != 0 && numIter < model.option.numIterations);
// get the end time of the training process
end_train = System.currentTimeMillis();
elapsed_train = end_train - start_train;
System.out.println("\tThe training process elapsed: " +
Double.toString((double)elapsed_train / 1000) + " seconds");
if (model.option.isLogging) {
fout.println("\tThe training process elapsed: " +
Double.toString((double)elapsed_train / 1000) + " seconds");
}
if (model.option.evaluateDuringTraining && model.option.saveBestModel) {
for (i = 0; i < numFeatures; i++) {
lambda[i] = tempLambda[i];
}
}
}
/**
* Compute log li gradient.
*
* @param lambda the lambda
* @param gradLogLi the grad log li
* @param numIter the num iter
* @param fout the fout
* @return the double
*/
public double computeLogLiGradient(double[] lambda, double[] gradLogLi,
int numIter, PrintWriter fout) {
double logLi = 0.0;
int ii, i;//, j, k;
for (i = 0; i < numFeatures; i++) {
gradLogLi[i] = -1 * lambda[i] / model.option.sigmaSquare;
logLi -= (lambda[i] * lambda[i]) / (2 * model.option.sigmaSquare);
}
// go through all training data examples/observations
for (ii = 0; ii < model.data.trnData.size(); ii++) {
Observation obsr = (Observation)model.data.trnData.get(ii);
for (i = 0; i < numLabels; i++) {
temp[i] = 0.0;
}
// log-likelihood value of the current data observation
double obsrLogLi = 0.0;
// start to scan all features at the current obsr
model.feaGen.startScanFeatures(obsr);
while (model.feaGen.hasNextFeature()) {
Feature f = model.feaGen.nextFeature();
if (f.label == obsr.humanLabel) {
gradLogLi[f.idx] += f.val;
obsrLogLi += lambda[f.idx] * f.val;
}
temp[f.label] += lambda[f.idx] * f.val;
}
double Zx = 0.0;
for (i = 0; i < numLabels; i++) {
Zx += Math.exp(temp[i]);
}
model.feaGen.scanReset();
while (model.feaGen.hasNextFeature()) {
Feature f = model.feaGen.nextFeature();
gradLogLi[f.idx] -= f.val * Math.exp(temp[f.label]) / Zx;
}
obsrLogLi -= Math.log(Zx);
logLi += obsrLogLi;
} // end of the main loop
System.out.println();
System.out.println("Iteration: " + Integer.toString(numIter));
System.out.println("\tLog-likelihood = " + Double.toString(logLi));
double gradLogLiNorm = Train.norm(gradLogLi);
System.out.println("\tNorm (log-likelihood gradient) = " + Double.toString(gradLogLiNorm));
double lambdaNorm = Train.norm(lambda);
System.out.println("\tNorm (lambda) = " + Double.toString(lambdaNorm));
if (model.option.isLogging) {
fout.println();
fout.println("Iteration: " + Integer.toString(numIter));
fout.println("\tLog-likelihood = " + Double.toString(logLi));
fout.println("\tNorm (log-likelihood gradient) = " + Double.toString(gradLogLiNorm));
fout.println("\tNorm (lambda) = " + Double.toString(lambdaNorm));
}
return logLi;
}
} // end of class Train
© 2015 - 2025 Weber Informatics LLC | Privacy Policy