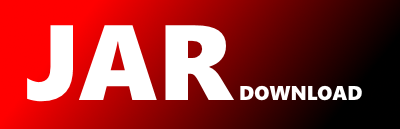
jflexcrf.Viterbi Maven / Gradle / Ivy
Go to download
Show more of this group Show more artifacts with this name
Show all versions of heideltime Show documentation
Show all versions of heideltime Show documentation
HeidelTime is a multilingual cross-domain temporal tagger that extracts temporal expressions from documents and normalizes them according to the TIMEX3 annotation standard.
/*
Copyright (C) 2010 by
*
* Cam-Tu Nguyen [email protected] [email protected]
* Xuan-Hieu Phan [email protected]
* College of Technology, Vietnamese University, Hanoi
*
* Graduate School of Information Sciences
* Tohoku University
*
* JVnTextPro-v.2.0 is a free software; you can redistribute it and/or modify
* it under the terms of the GNU General Public License as published
* by the Free Software Foundation; either version 2 of the License,
* or (at your option) any later version.
*
* JVnTextPro-v.2.0 is distributed in the hope that it will be useful, but
* WITHOUT ANY WARRANTY; without even the implied warranty of
* MERCHANTABILITY or FITNESS FOR A PARTICULAR PURPOSE. See the
* GNU General Public License for more details.
*
* You should have received a copy of the GNU General Public License
* along with JVnTextPro-v.2.0); if not, write to the Free Software Foundation,
* Inc., 59 Temple Place, Suite 330, Boston, MA 02111-1307 USA.
*/
package jflexcrf;
import java.io.*;
import java.util.*;
// TODO: Auto-generated Javadoc
/**
* The Class Viterbi.
*/
public class Viterbi {
/** The model. */
public Model model = null;
/** The num labels. */
int numLabels = 0;
/** The Mi. */
DoubleMatrix Mi = null;
/** The Vi. */
DoubleVector Vi = null;
/**
* The Class PairDblInt.
*/
public class PairDblInt {
/** The first. */
public double first = 0.0;
/** The second. */
public int second = -1;
} // enf of class PairDblInt
/** The memory size. */
public int memorySize = 0;
/** The memory. */
public PairDblInt[][] memory = null;
/**
* Instantiates a new viterbi.
*/
public Viterbi() {
}
/**
* Inits the.
*
* @param model the model
*/
public void init(Model model) {
this.model = model;
numLabels = model.taggerMaps.numLabels();
Mi = new DoubleMatrix(numLabels, numLabels);
Vi = new DoubleVector(numLabels);
allocateMemory(100);
// compute Mi once at initialization
computeMi();
}
/**
* Allocate memory.
*
* @param memorySize the memory size
*/
public void allocateMemory(int memorySize) {
this.memorySize = memorySize;
memory = new PairDblInt[memorySize][numLabels];
for (int i = 0; i < memorySize; i++) {
for (int j = 0; j < numLabels; j++) {
memory[i][j] = new PairDblInt();
}
}
}
/**
* Compute mi.
*/
public void computeMi() {
Mi.assign(0.0);
model.taggerFGen.startScanEFeatures();
while (model.taggerFGen.hasNextEFeature()) {
Feature f = model.taggerFGen.nextEFeature();
if (f.ftype == Feature.EDGE_FEATURE1) {
Mi.mtrx[f.yp][f.y] += model.lambda[f.idx] * f.val;
}
}
for (int i = 0; i < Mi.rows; i++) {
for (int j = 0; j < Mi.cols; j++) {
Mi.mtrx[i][j] = Math.exp(Mi.mtrx[i][j]);
}
}
}
/**
* Compute vi.
*
* @param seq the seq
* @param pos the pos
* @param Vi the vi
* @param isExp the is exp
*/
public void computeVi(List seq, int pos, DoubleVector Vi, boolean isExp) {
Vi.assign(0.0);
// start scan features for sequence "seq" at position "pos"
model.taggerFGen.startScanSFeaturesAt(seq, pos);
// examine all features at position "pos"
while (model.taggerFGen.hasNextSFeature()) {
Feature f = model.taggerFGen.nextSFeature();
if (f.ftype == Feature.STAT_FEATURE1) {
Vi.vect[f.y] += model.lambda[f.idx] * f.val;
}
}
// take exponential operator
if (isExp) {
for (int i = 0; i < Vi.len; i++) {
Vi.vect[i] = Math.exp(Vi.vect[i]);
}
}
}
// list is a List of PairDblInt
/**
* Sum.
*
* @param cols the cols
* @return the double
*/
public double sum(PairDblInt[] cols) {
double res = 0.0;
for (int i = 0; i < numLabels; i++) {
res += cols[i].first;
}
if (res < 1 && res > -1) {
res = 1;
}
return res;
}
// list is a List of PairDblInt
/**
* Divide.
*
* @param cols the cols
* @param val the val
*/
public void divide(PairDblInt[] cols, double val) {
for (int i = 0; i < numLabels; i++) {
cols[i].first /= val;
}
}
// list is a List of PairDblInt
/**
* Find max.
*
* @param cols the cols
* @return the int
*/
public int findMax(PairDblInt[] cols) {
int maxIdx = 0;
double maxVal = -1.0;
for (int i = 0; i < numLabels; i++) {
if (cols[i].first > maxVal) {
maxVal = cols[i].first;
maxIdx = i;
}
}
return maxIdx;
}
/**
* Viterbi inference.
*
* @param seq the seq
*/
public void viterbiInference(List seq) {
int i, j, k;
int seqLen = seq.size();
if (seqLen <= 0) {
return;
}
if (memorySize < seqLen) {
allocateMemory(seqLen);
}
// compute Vi for the first position in the sequence
computeVi(seq, 0, Vi, true);
for (j = 0; j < numLabels; j++) {
memory[0][j].first = Vi.vect[j];
memory[0][j].second = j;
}
// scaling for the first position
divide(memory[0], sum(memory[0]));
// the main loop
for (i = 1; i < seqLen; i++) {
// compute Vi at the position i
computeVi(seq, i, Vi, true);
// for all possible labels at the position i
for (j = 0; j < numLabels; j++) {
memory[i][j].first = 0.0;
memory[i][j].second = 0;
// find the maximal value and its index and store them in memory
// for later tracing back to find the best path
for (k = 0; k < numLabels; k++) {
double tempVal = memory[i - 1][k].first *
Mi.mtrx[k][j] * Vi.vect[j];
if (tempVal > memory[i][j].first) {
memory[i][j].first = tempVal;
memory[i][j].second = k;
}
}
}
// scaling for memory at position i
divide(memory[i], sum(memory[i]));
}
// viterbi backtrack to find the best label path
int maxIdx = findMax(memory[seqLen - 1]);
((Observation)seq.get(seqLen - 1)).modelLabel = maxIdx;
for (i = seqLen - 2; i >= 0; i--) {
((Observation)seq.get(i)).modelLabel =
memory[i + 1][maxIdx].second;
maxIdx = ((Observation)seq.get(i)).modelLabel;
}
}
} // end of class Viterbi
© 2015 - 2024 Weber Informatics LLC | Privacy Policy