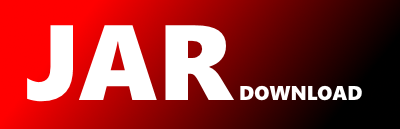
xf.xfvrp.opt.XFVRPOptSplitter Maven / Gradle / Ivy
Go to download
Show more of this group Show more artifacts with this name
Show all versions of xfvrp Show documentation
Show all versions of xfvrp Show documentation
Solver for realistic vehicle routing problems
package xf.xfvrp.opt;
import java.util.ArrayList;
import java.util.Arrays;
import java.util.List;
import java.util.Random;
import java.util.stream.Collectors;
import xf.xfvrp.base.Node;
import xf.xfvrp.base.Quality;
import xf.xfvrp.base.SiteType;
import xf.xfvrp.base.XFVRPModel;
import xf.xfvrp.base.monitor.StatusManager;
/**
* Copyright (c) 2012-present Holger Schneider
* All rights reserved.
*
* This source code is licensed under the MIT License (MIT) found in the
* LICENSE file in the root directory of this source tree.
*
*
* General concept in classic Container Routing is the independence of routes.
* A change in one route leaves all decisions in another route valid. So the
* separation of route plan in several blocks is possible and their union either.
*
* This class provides methods to optimize a big route plan by separating it into
* several blocks, where each block may contains ALLOWED_NBR_OF_CUSTOMERS_IN_BLOCK
* number of customers. Each block is optimized by a given optimization procedure.
* Last but not least the routes of all blocks are joined.
*
* The loss of separation is covered by iteration of this separation and union, where
* the separation process is randomized. So the set of routes is different in each
* iteration. If optimization can not found an improvement in at least one block for
* ALLOWED_NON_IMPROVES times, the search process will be terminated.
*
* @author hschneid
*
*/
public class XFVRPOptSplitter {
private static int ALLOWED_NBR_OF_CUSTOMERS_IN_BLOCK = 250;
private static int ALLOWED_NBR_OF_BLOCKS = 1;
private static int ALLOWED_NBR_OF_NON_IMPROVES = 2;
/**
* Executes the optimization by splitting a big route plan into smaller
* blocks with less routes and optimizes each block independently. Afterwards
* the optimized blocks are joined back. This process is iterated until no further
* improvement could be found.
*
* @param solution Current route plan
* @param model Model with nodes, distances and parameters
* @param opt Optimization procedure
* @param statusManager
* @return Optimized route plan
*/
public Solution execute(final Solution solution, XFVRPModel model, StatusManager statusManager, XFVRPOptBase opt) {
init(model);
Solution best = solution.copy();
float bestCost = getCost(solution, opt);
int nbrOfTries = 0;
while(nbrOfTries < ALLOWED_NBR_OF_NON_IMPROVES) {
List blocks = splitIntoBlocks(best, opt.getRandom());
Solution newSolution = optimizeBlocks(model, statusManager, opt, blocks);
float cost = getCost(newSolution, opt);
nbrOfTries++;
if(cost < bestCost) {
best = newSolution;
bestCost = cost;
nbrOfTries = 0;
}
}
return best;
}
private void init(XFVRPModel model) {
ALLOWED_NBR_OF_BLOCKS = (int)Math.max(1, model.getNbrOfNodes() / (float)ALLOWED_NBR_OF_CUSTOMERS_IN_BLOCK);
}
private Solution optimizeBlocks(XFVRPModel model, StatusManager statusManager, XFVRPOptBase opt, List blocks) {
List resultGiantList = new ArrayList<>();
for (Solution partialSolution : blocks) {
opt.execute(partialSolution, model, statusManager);
resultGiantList.addAll(Arrays.asList(partialSolution.getGiantRoute()));
}
Solution newSolution = new Solution();
newSolution.setGiantRoute(resultGiantList.toArray(new Node[0]));
return newSolution;
}
/**
* Splits a big route plan into smaller blocks. The number of
* blocks is precalculated by the average number of customers
* in a block. A route can not be split into two routes.
*
* @param giantRoute Current route plan
* @return List of blocks, where each block is a route plan (small giant tour)
*/
private static List splitIntoBlocks(Solution solution, Random random) {
List[] blocks = initBlocks();
fillRoutesIntoBlocks(solution, blocks, random);
addDepotsToBlocks(blocks);
return convertToSolutions(solution, blocks);
}
private static List convertToSolutions(Solution solution, List[] blocks) {
List partialSolutions = Arrays.stream(blocks)
.filter(b -> b.size() > 0)
.map(b -> {
Solution sol = new Solution();
sol.setGiantRoute(b.toArray(new Node[0]));
return solution;
})
.collect(Collectors.toList());
return partialSolutions;
}
private static void addDepotsToBlocks(List[] blocks) {
for (int i = 0; i < blocks.length; i++)
if(blocks[i].size() > 0)
blocks[i].add(blocks[i].get(0));
}
private static void fillRoutesIntoBlocks(Solution solution, List[] blocks, Random random) {
int lastDepot = 0;
Node[] giantTour = solution.getGiantRoute();
for (int i = 1; i < giantTour.length; i++) {
if(giantTour[i].getSiteType() == SiteType.DEPOT) {
int blockIdx = random.nextInt(ALLOWED_NBR_OF_BLOCKS);
for (int j = lastDepot; j < i; j++)
blocks[blockIdx].add(giantTour[j]);
lastDepot = i;
}
}
}
@SuppressWarnings("unchecked")
private static List[] initBlocks() {
List[] blocks = new ArrayList[ALLOWED_NBR_OF_BLOCKS];
for (int i = 0; i < blocks.length; i++)
blocks[i] = new ArrayList<>();
return blocks;
}
/**
* Returns the cost of route plan by building up a report.
*
* @param giantRoute Current route plan
* @param optBase Current model with nodes, distances and parameters
* @return Fitness of current solution
*/
private float getCost(Solution solution, XFVRPOptBase optBase) {
Quality quality = optBase.check(solution);
return quality.getCost();
}
}
© 2015 - 2025 Weber Informatics LLC | Privacy Policy