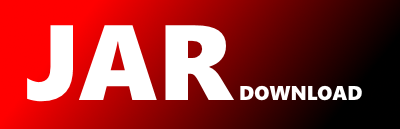
math.stats.distribution.fit.UniformTestStatistics Maven / Gradle / Ivy
Go to download
Show more of this group Show more artifacts with this name
Show all versions of finwhale Show documentation
Show all versions of finwhale Show documentation
Statistical distributions library (in statu nascendi)
The newest version!
/*
* Class: GofFormat
* Description:
* Environment: Java
* Software: SSJ
* Copyright (C) 2001 Pierre L'Ecuyer and Universite de Montreal
* Organization: DIRO, Universite de Montreal
*
* Licensed under the Apache License, Version 2.0 (the "License");
* you may not use this file except in compliance with the License.
* You may obtain a copy of the License at
*
* http://www.apache.org/licenses/LICENSE-2.0
*
* Unless required by applicable law or agreed to in writing, software
* distributed under the License is distributed on an "AS IS" BASIS,
* WITHOUT WARRANTIES OR CONDITIONS OF ANY KIND, either express or implied.
* See the License for the specific language governing permissions and
* limitations under the License.
*/
package math.stats.distribution.fit;
import math.Arithmetic;
import math.MathConsts;
import math.stats.ValidatedValue;
/**
* Summary classes for values associated with goodness of fit tests.
* {@link Result} summarizes the values of several test statistics (mainly the
* Anderson-Darling {@link Result#AD} and Kolomogorov-Smirnov {@link Result#KS}
* test statistics). {@link PValue} summarizes the p-values
* ({@link PValue#AD_PVAL} for Anderson-Darling and {@link PValue#KS_PVAL} for
* Kolmogorov-Smirnov) for a given test statistic.
*/
public final class UniformTestStatistics {
/**
* {@link #AD} contains the value of the Anderson-Darling test statistic.
* {@link #KS} contains the value of the Kolmogorov-Smirnov test statistic.
* If a statistic couldn't be computed or wasn't computed it contains the
* value {@link Double#NaN} (which is also the initialization value for all
* members of this class).
*/
public static final class Result implements ValidatedValue {
/**
* Kolmogorov-Smirnov+ test statistic
*/
public double KSP = Double.NaN;
/**
* Kolmogorov-Smirnov- test statistic
*/
public double KSM = Double.NaN;
/**
* Kolmogorov-Smirnov test statistic
*/
public double KS = Double.NaN;
/**
* Anderson-Darling test statistic
*/
public double AD = Double.NaN;
/**
* Cramér-von Mises test statistic
*/
public double CM = Double.NaN;
/**
* Watson G test statistic
*/
public double WG = Double.NaN;
/**
* Watson U test statistic
*/
public double WU = Double.NaN;
/**
* Mean
*/
public double MEAN = Double.NaN;
/**
* Number of observations
*/
public int N = -1;
/**
* {@inheritDoc}
*/
@Override
public boolean isValid() {
return N > 0 && !(Arithmetic.isBadNum(KS) || Arithmetic.isBadNum(AD));
}
/**
* {@inheritDoc}
*/
@Override
public String toString() {
StringBuilder b = new StringBuilder(256);
b.append("\r\n");
// b.append("KSP D+ : ").append(KSP).append("\r\n");
// b.append("KSM D- : ").append(KSM).append("\r\n");
b.append("KS D : ").append(KS).append("\r\n");
b.append("AD A2 : ").append(AD).append("\r\n");
// b.append("CM W2 : ").append(CM).append("\r\n");
// b.append("WG G : ").append(WG).append("\r\n");
// b.append("WU U2 : ").append(WU).append("\r\n");
b.append("MEAN : ").append(MEAN).append("\r\n");
b.append("N : ").append(N).append("\r\n\r\n");
return b.toString();
}
}
/**
* {@link #AD_PVAL} contains the p-value for the Anderson-Darling test.
* {@link #KS_PVAL} contains the p-value for the Kolmogorov-Smirnov test. If
* a p-value couldn't be computed or wasn't computed it contains the value
* {@link Double#NaN} (which is also the initialization value for all
* members of this class).
*/
public static final class PValue implements ValidatedValue {
/**
* Kolmogorov-Smirnov+ test p-value
*/
public double KSP_PVAL = Double.NaN;
/**
* Kolmogorov-Smirnov- test p-value
*/
public double KSM_PVAL = Double.NaN;
/**
* Kolmogorov-Smirnov test p-value
*/
public double KS_PVAL = Double.NaN;
/**
* Anderson-Darling test p-value
*/
public double AD_PVAL = Double.NaN;
/**
* Number of observations
*/
public int N = -1;
/**
* {@inheritDoc}
*/
@Override
public boolean isValid() {
return N > 0 && Arithmetic.isProbability(KS_PVAL) && Arithmetic.isProbability(AD_PVAL);
}
/**
* {@inheritDoc}
*/
@Override
public String toString() {
// String ksp = "KolmogorovSmirnovPlus p-value: " + KSP_PVAL;
// String ksm = "KolmogorovSmirnovMinus p-value: " + KSM_PVAL;
String ks = "KolmogorovSmirnov p-value: " + KS_PVAL;
String ad = "Anderson-Darling p-value: " + AD_PVAL;
String size = "Sample size : " + N;
StringBuilder b = new StringBuilder(384);
b.append("\r\n");
// b.append(ksp).append("\r\n");
// b.append(ksm).append("\r\n");
b.append(ks).append("\r\n");
b.append(ad).append("\r\n");
b.append(size).append("\r\n\r\n");
return b.toString();
}
}
private static final double EPS = MathConsts.BIG_INV / 2.0;
/**
* Computes the {@link UniformTestStatistics.Result} for a sorted array of
* observations assuming they are IID Uniform distributed over
* {@code (0,1)}.
*
* @param obs
* sorted (!) array of observations
* @return the {@link UniformTestStatistics.Result} for the given
* observations assuming they are IID Uniform distributed over
* {@code (0,1)}.
*/
static Result compareEmpiricalToUniform(double[] obs) {
if (obs == null || obs.length == 0) {
throw new IllegalArgumentException("obs == null || obs.length == 0");
}
Result statistic = new Result();
// we assume that obs is already sorted
if (obs.length == 1) {
statistic.KSP = 1.0 - obs[0];
statistic.MEAN = obs[0];
statistic.N = 1;
return statistic;
}
final int n = obs.length;
final double share = 1.0 / n;
double a2 = 0.0;
double dm = 0.0;
double dp = 0.0;
double w2 = share / 12.0;
double sumZ = 0.0;
for (int i = 0; i < n; i++) {
// KS statistics
double d1 = obs[i] - i * share;
double d2 = (i + 1) * share - obs[i];
if (d1 > dm) {
dm = d1;
}
if (d2 > dp) {
dp = d2;
}
// Watson U and G
sumZ += obs[i];
double w = obs[i] - (i + 0.5) * share;
w2 += w * w;
// Anderson-Darling
double ui = obs[i];
double u1 = 1.0 - ui;
if (ui < EPS) {
ui = EPS;
} else if (u1 < EPS) {
u1 = EPS;
}
a2 += (2 * i + 1) * Math.log(ui) + (1 + 2 * (n - i - 1)) * Math.log(u1);
}
if (dm > dp) {
statistic.KS = dm;
} else {
statistic.KS = dp;
}
statistic.KSM = dm;
statistic.KSP = dp;
sumZ = sumZ * share - 0.5;
statistic.CM = w2;
statistic.WG = Math.sqrt((double) n) * (dp + sumZ);
statistic.WU = w2 - sumZ * sumZ * n;
statistic.AD = -n - a2 * share;
statistic.MEAN = sumZ + 0.5;
statistic.N = n;
return statistic;
}
private UniformTestStatistics() {
throw new AssertionError();
}
}
© 2015 - 2024 Weber Informatics LLC | Privacy Policy