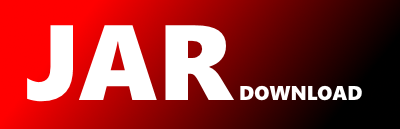
math.stats.distribution.mle.MLE Maven / Gradle / Ivy
Go to download
Show more of this group Show more artifacts with this name
Show all versions of finwhale Show documentation
Show all versions of finwhale Show documentation
Statistical distributions library (in statu nascendi)
The newest version!
/*
* Software: SSJ
* Copyright (C) 2001 Pierre L'Ecuyer and Universite de Montreal
* Organization: DIRO, Universite de Montreal
* Environment: Java
*
* Licensed under the Apache License, Version 2.0 (the "License");
* you may not use this file except in compliance with the License.
* You may obtain a copy of the License at
*
* http://www.apache.org/licenses/LICENSE-2.0
*
* Unless required by applicable law or agreed to in writing, software
* distributed under the License is distributed on an "AS IS" BASIS,
* WITHOUT WARRANTIES OR CONDITIONS OF ANY KIND, either express or implied.
* See the License for the specific language governing permissions and
* limitations under the License.
*/
package math.stats.distribution.mle;
import math.Arithmetic;
import math.FastGamma;
import math.FastMath;
import math.GammaFun;
import math.MathConsts;
import math.RootFinder;
import math.function.DoubleUnaryOperator;
import math.function.MultivariateFunctionResult;
import math.function.NumericallyDiffMultivariateFunction;
import math.minpack.Lmder_fcn;
import math.minpack.Minpack_f77;
import math.optim.CGOptimizer;
/**
* Provides methods for maximum likelihood estimation of distribution
* parameters.
*/
public final class MLE {
private static final double LN_EPS = MathConsts.LN_MIN_NORMAL - MathConsts.LN_2;
private static final double HUGE = 1.0e200;
private static final double BIG = 1.0e100;
private static final double MU_INCR = 0.1;
private static final String NO_OBS_MSG = "No observations (x[].length = 0)";
private static final class GammaMLE implements DoubleUnaryOperator {
private final int n;
private final double empiricalMean;
private final double sumLn;
GammaMLE(int n, double empiricalMean, double sumLn) {
this.n = n;
this.empiricalMean = empiricalMean;
this.sumLn = sumLn;
}
@Override
public double applyAsDouble(double x) {
if (x <= 0.0) {
return HUGE;
}
return (n * Math.log(empiricalMean / x) + n * GammaFun.digamma(x) - sumLn);
}
}
private static final class WeibullMLE implements DoubleUnaryOperator {
private final double xi[];
private final double lnXi[];
private double sumLnXi = 0.0;
WeibullMLE(double x[]) {
xi = x.clone();
lnXi = new double[x.length];
for (int i = 0; i < x.length; i++) {
double lnx;
if (x[i] > 0.0) {
lnx = Math.log(x[i]);
lnXi[i] = lnx;
} else {
lnx = LN_EPS;
lnXi[i] = lnx;
}
sumLnXi += lnx;
}
}
@Override
public double applyAsDouble(double x) {
if (x <= 0.0) {
return HUGE;
}
double sumXiLnXi = 0.0;
double sumXi = 0.0;
for (int i = 0; i < xi.length; i++) {
double xalpha = FastMath.pow(xi[i], x);
sumXiLnXi += xalpha * lnXi[i];
sumXi += xalpha;
}
return x * (xi.length * sumXiLnXi - sumLnXi * sumXi) - (xi.length * sumXi);
}
}
private static final class StudentTMLE implements DoubleUnaryOperator {
private final double[] xi;
StudentTMLE(double[] x) {
xi = x.clone();
}
@Override
public double applyAsDouble(double df) {
if (df <= 0.0) {
return HUGE;
}
double sum = 0.0;
for (int i = 0; i < xi.length; i++) {
sum += Math.log(pdf(df, xi[i]));
}
return sum;
}
private static double pdf(double df, double x) {
double tmp = FastGamma.logGamma((df + 1.0) / 2.0) - FastGamma.logGamma(df / 2.0);
double pdfConst = FastMath.exp(tmp) / Math.sqrt(Math.PI * df);
return pdfConst * FastMath.pow((1.0 + x * x / df), -(df + 1.0) * 0.5);
}
}
private static final class BetaMLE implements Lmder_fcn {
private final double a;
private final double b;
BetaMLE(double a, double b) {
this.a = a;
this.b = b;
}
@Override
public void fcn(int m, int n, double[] x, double[] fvec, double[][] fjac, int[] iflag) {
if (x[1] <= 0.0 || x[2] <= 0.0) {
fvec[1] = BIG;
fvec[2] = BIG;
fjac[1][1] = BIG;
fjac[1][2] = 0.0;
fjac[2][1] = 0.0;
fjac[2][2] = BIG;
return;
}
if (iflag[1] == 1) {
double trig = GammaFun.digamma(x[1] + x[2]);
fvec[1] = GammaFun.digamma(x[1]) - trig - a;
fvec[2] = GammaFun.digamma(x[2]) - trig - b;
} else if (iflag[1] == 2) {
double trig = GammaFun.trigamma(x[1] + x[2]);
fjac[1][1] = GammaFun.trigamma(x[1]) - trig;
fjac[1][2] = -trig;
fjac[2][1] = -trig;
fjac[2][2] = GammaFun.trigamma(x[2]) - trig;
}
}
}
private static final class ChiSquareMLE extends NumericallyDiffMultivariateFunction {
private static final double TERM = MathConsts.LN_2 / 2.0;
private final double sumLnHalfth;
private final int n;
ChiSquareMLE(double sumLn, int n) {
this.sumLnHalfth = sumLn / 2.0;
this.n = n;
}
@Override
public double valueAt(double[] point) {
double x = point[0];
if (x <= 0.0) {
return -HUGE;
}
return x * sumLnHalfth - n * FastGamma.logGamma(x / 2.0) - (n * x) * TERM;
}
}
/**
* Estimates the parameters {@code shape} ({@code k}) and {@code scale}
* (θ) of the Gamma distribution from the observations {@code x} using
* the maximum likelihood method.
*
* @param x
* the list of observations to use to evaluate parameters
* @return returns the parameters {@code k} and θ
*/
public static ParGamma getGammaMLE(double[] x) {
int n = getLength(x);
double sum = 0.0;
double sumLn = 0.0;
for (int i = 0; i < n; i++) {
sum += x[i];
if (x[i] <= 0.0) {
sumLn += LN_EPS;
} else {
sumLn += Math.log(x[i]);
}
}
double empiricalMean = sum / (double) n;
sum = 0.0;
for (int i = 0; i < n; i++) {
sum += (x[i] - empiricalMean) * (x[i] - empiricalMean);
}
double alphaMME = (empiricalMean * empiricalMean * (double) n) / sum;
// left endpoint of initial interval
double left = alphaMME - 10.0;
if (left <= 0) {
left = 1.0e-5;
}
// right endpoint of initial interval
double right = alphaMME + 10.0;
ParGamma params = new ParGamma();
params.shape = RootFinder.brentDekker(left, right, new GammaMLE(n, empiricalMean, sumLn), 1e-7);
params.scale = empiricalMean / params.shape;
return params;
}
/**
* Estimates the parameters μ and σ of the LogNormal distribution
* from the observations {@code x} using the maximum likelihood method.
*
* @param x
* the list of observations to use to evaluate parameters
* @return returns the parameters μ and σ
*/
public static ParLogNormal getLogNormalMLE(double[] x) {
int n = getLength(x);
double sum = 0.0;
for (int i = 0; i < n; i++) {
if (x[i] > 0.0) {
sum += Math.log(x[i]);
} else {
sum += LN_EPS; // log(MIN_NORMAL / 2)
}
}
double mu_hat = sum / n;
double tmp;
sum = 0.0;
for (int i = 0; i < n; i++) {
if (x[i] > 0.0) {
tmp = Math.log(x[i]) - mu_hat;
} else {
tmp = LN_EPS - mu_hat;
}
sum += (tmp * tmp);
}
ParLogNormal params = new ParLogNormal();
params.mu = mu_hat;
params.sigma = Math.sqrt(sum / n);
return params;
}
/**
* Estimates the parameters {@code scale} (λ) and {@code shape}
* ({@code k}) of the Weibull distribution from the observations {@code x}
* using the maximum likelihood method.
*
* @param x
* the list of observations to use to evaluate parameters
* @return returns the parameters λ and {@code k}
*/
public static ParWeibull getWeibullMLE(double[] x) {
int n = getLength(x);
double sumLn = 0.0;
double sumLn2 = 0.0;
for (int i = 0; i < x.length; i++) {
double lnxi;
if (x[i] <= 0.0) {
lnxi = LN_EPS;
} else {
lnxi = Math.log(x[i]);
}
sumLn += lnxi;
sumLn2 += (lnxi * lnxi);
}
double alpha0 = Math.sqrt((double) n / ((6.0 / MathConsts.PI_SQUARED) * (sumLn2 - sumLn * sumLn / (double) n)));
// left endpoint of initial interval
double left = alpha0 - 20.0;
if (left <= 0.0) {
left = 1.0e-5;
}
// right endpoint of initial interval
double right = alpha0 + 20.0;
double k = RootFinder.brentDekker(left, right, new WeibullMLE(x), 1e-5);
double sumXalpha = 0.0;
for (int i = 0; i < x.length; i++) {
sumXalpha += FastMath.pow(x[i], k);
}
double scale = 1.0 / (FastMath.pow((double) n / sumXalpha, 1.0 / k));
ParWeibull params = new ParWeibull();
params.shape = k;
params.scale = scale;
return params;
}
/**
* Estimates the parameter μ (degrees of freedom) of the StudentT
* distribution from the observations {@code x} using the maximum likelihood
* method. Note that this implementation allows for double-valued
* estimators.
*
* @param x
* the list of observations to use to evaluate parameters
* @return returns the parameter μ
*/
public static ParStudentT getStudentTMLE(double[] x) {
int n = getLength(x);
double var = 0.0;
for (int i = 0; i < x.length; i++) {
var += (x[i] * x[i]);
}
var /= (double) n;
StudentTMLE f = new StudentTMLE(x);
double n0 = (2.0 * var) / (var - 1.0);
double fn0 = f.applyAsDouble(n0);
double min = fn0;
double fna = f.applyAsDouble(n0 - MU_INCR);
double fnb = f.applyAsDouble(n0 + MU_INCR);
double df_est = n0;
if (fna > fn0) {
double mu = n0 - MU_INCR;
double y;
while (((y = f.applyAsDouble(mu)) > min) && (mu > 0.0)) {
min = y;
df_est = mu;
mu -= MU_INCR;
}
} else if (fnb > fn0) {
double mu = n0 + MU_INCR;
double y;
while ((y = f.applyAsDouble(mu)) > min) {
min = y;
df_est = mu;
mu += MU_INCR;
}
}
ParStudentT param = new ParStudentT();
param.df = Arithmetic.round(df_est);
return param;
}
/**
* Estimates the parameters {@code alpha} (α) and {@code beta}
* (β) of the Beta distribution from the observations {@code x} using
* the maximum likelihood method.
*
* @param x
* the list of observations to use to evaluate parameters
* @return returns the parameters {@code alpha} (α) and {@code beta}
* (β)
*/
public static ParBeta getBetaMLE(double[] x) {
int n = getLength(x);
double sum = 0.0;
double a = 0.0;
double b = 0.0;
for (int i = 0; i < x.length; i++) {
sum += x[i];
if (x[i] > 0.0) {
a += Math.log(x[i]);
} else {
a += LN_EPS;
}
if (x[i] < 1.0) {
b += FastMath.log1p(-x[i]);
} else {
b += LN_EPS;
}
}
double mean = sum / n;
sum = 0.0;
for (int i = 0; i < x.length; i++) {
sum += (x[i] - mean) * (x[i] - mean);
}
double var = sum / (n - 1);
// param[0] unused because of FORTRAN indexing convention
double[] param = new double[3];
param[1] = mean * ((mean * (1.0 - mean) / var) - 1.0);
param[2] = (1.0 - mean) * ((mean * (1.0 - mean) / var) - 1.0);
// all of them unused
double[] fvec = new double[3];
double[][] fjac = new double[3][3];
int[] info = new int[2];
int[] ipvt = new int[3];
Minpack_f77.lmder1_f77(new BetaMLE(a, b), 2, 2, param, fvec, fjac, 1e-5, info, ipvt);
ParBeta params = new ParBeta();
params.alpha = param[1];
params.beta = param[2];
return params;
}
/**
* Estimates the parameter {@code k} (degrees of freedom) of the ChiSquare
* distribution from the observations {@code x} using the maximum likelihood
* method. Note that this implementation allows for double-valued
* estimators.
*
* @param x
* the list of observations to use to evaluate parameters
* @return returns the parameter {@code k} (degrees of freedom)
*/
public static ParChiSquare getChiSquareMLE(double[] x) {
int n = getLength(x);
double sumLn = 0.0;
for (int i = 0; i < x.length; i++) {
if (x[i] > 0.0) {
sumLn += Math.log(x[i]);
} else {
sumLn += LN_EPS;
}
}
MultivariateFunctionResult res = CGOptimizer.maximize(new ChiSquareMLE(sumLn, n),
new double[] { 0.001, 100.0 });
ParChiSquare param = new ParChiSquare();
param.degreesOfFreedom = res.point[0];
return param;
}
/**
* Estimates the parameter λ (rate) of the Exponential distribution
* from the observations {@code x} using the maximum likelihood method.
*
* @param x
* the list of observations to use to evaluate parameters
* @return returns the exponential rate parameter λ
*/
public static ParExponential getExponentialMLE(double[] x) {
int n = getLength(x);
double sum = 0.0;
for (int i = 0; i < x.length; i++) {
sum += x[i];
}
ParExponential param = new ParExponential();
param.lambda = (double) n / sum;
return param;
}
/**
* Estimates the parameters {@code mean} (μ) and {@code stdDev} (σ)
* of the Normal distribution from the observations {@code x} using the
* maximum likelihood method.
*
* @param x
* the list of observations to use to evaluate parameters
* @return returns the parameters {@code mean} (μ) and {@code stdDev}
* (σ)
*/
public static ParNormal getNormalMLE(double[] x) {
int n = getLength(x);
double sum = 0.0;
for (int i = 0; i < x.length; i++) {
sum += x[i];
}
double sigma = sum / n;
sum = 0.0;
for (int i = 0; i < x.length; i++) {
double dev = x[i] - sigma;
sum = sum + (dev * dev);
}
ParNormal params = new ParNormal();
params.mean = sigma;
params.stdDev = Math.sqrt(sum / n);
return params;
}
private static int getLength(double[] x) {
int n = x.length;
if (n == 0) {
throw new IllegalArgumentException(NO_OBS_MSG);
}
return n;
}
private MLE() {
throw new AssertionError();
}
}
© 2015 - 2024 Weber Informatics LLC | Privacy Policy