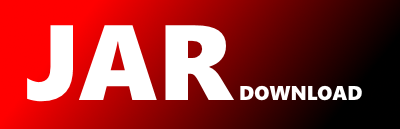
com.github.tommyettinger.random.GoldenQuasiRandom Maven / Gradle / Ivy
Go to download
Show more of this group Show more artifacts with this name
Show all versions of juniper Show documentation
Show all versions of juniper Show documentation
Serializable pseudo-random number generators and distributions.
The newest version!
/*
* Copyright (c) 2022-2023 See AUTHORS file.
*
* Licensed under the Apache License, Version 2.0 (the "License");
* you may not use this file except in compliance with the License.
* You may obtain a copy of the License at
*
* http://www.apache.org/licenses/LICENSE-2.0
*
* Unless required by applicable law or agreed to in writing, software
* distributed under the License is distributed on an "AS IS" BASIS,
* WITHOUT WARRANTIES OR CONDITIONS OF ANY KIND, either express or implied.
* See the License for the specific language governing permissions and
* limitations under the License.
*
*/
package com.github.tommyettinger.random;
import com.github.tommyettinger.digital.Distributor;
import com.github.tommyettinger.digital.Hasher;
import java.util.Random;
/**
* Not actually a pseudo-random number generator, but a quasi-random number generator, this is an extremely simple
* way to produce random-seeming numbers with a high distance between one number and the next. This has a period of
* 2 to the 64. It does not pass any tests for randomness. This is simply a counter with a specific large increment:
* 2 to the 64 divided by the golden ratio.
*
* Useful traits of this generator are that it has exactly one {@code long} of state, that all values are
* permitted for that state, and that you can {@link #skip(long)} the state forwards or backwards in constant time.
* It is also extremely fast, though it shouldn't be compared to pseudo-random number generators. It implements
* {@link #nextGaussian()} and its overload specially; these methods advance the state differently and don't return
* quasi-random output (it's much closer to pseudo-random, and is similar to {@link DistinctRandom}'s approach). The
* Gaussian methods needed this treatment because anything that requested multiple Gaussian-distributed variables each
* time it produced one output (such as a Chi or Beta distribution) would have extremely noticeable, severe artifacts.
* Because there's always a strong separation between subsequent results of {@link #nextDouble()}, that made the
* Gaussian doubles have large gaps in their output range, because some combinations were impossible.
*
* This class is an {@link EnhancedRandom} from juniper and is also a JDK {@link Random} as a result.
*
* This doesn't randomize the seed when given one with {@link #setSeed(long)}, and it doesn't do anything else to
* randomize the output, so sequential seeds will produce extremely similar sequences. You can randomize sequential
* seeds using something like {@link Hasher#randomize3(long)}, if you want random starting points.
*
* This implements all methods from {@link EnhancedRandom}, including the optional {@link #skip(long)} and
* {@link #previousLong()} methods.
*/
public class GoldenQuasiRandom extends EnhancedRandom {
/**
* The only long state variable; can be any {@code long}.
*/
public long state;
/**
* Creates a new GoldenQuasiRandom with a random state.
*/
public GoldenQuasiRandom() {
this(EnhancedRandom.seedFromMath());
}
/**
* Creates a new GoldenQuasiRandom with the given state; all {@code long} values are permitted.
*
* @param state any {@code long} value
*/
public GoldenQuasiRandom(long state) {
super(state);
this.state = state;
}
@Override
public String getTag() {
return "GoQR";
}
/**
* This has one long state.
*
* @return 1 (one)
*/
@Override
public int getStateCount () {
return 1;
}
/**
* Gets the only state, which can be any long value.
*
* @param selection ignored; this always returns the same, only state
* @return the only state's exact value
*/
@Override
public long getSelectedState (int selection) {
return state;
}
/**
* Sets the only state, which can be given any long value. The selection
* can be anything and is ignored.
*
* @param selection ignored; this always sets the same, only state
* @param value the exact value to use for the state; all longs are valid
*/
@Override
public void setSelectedState (int selection, long value) {
state = value;
}
/**
* Sets the only state, which can be given any long value; this seed value
* will not be altered. Equivalent to {@link #setSelectedState(int, long)}
* with any selection and {@code seed} passed as the {@code value}.
*
* @param seed the exact value to use for the state; all longs are valid
*/
@Override
public void setSeed (long seed) {
state = seed;
}
/**
* Gets the current state; it's already public, but I guess this could still
* be useful. The state can be any {@code long}.
*
* @return the current state, as a long
*/
public long getState () {
return state;
}
/**
* Sets each state variable to the given {@code state}. This implementation
* simply sets the one state variable to {@code state}.
*
* @param state the long value to use for the state variable
*/
@Override
public void setState (long state) {
this.state = state;
}
@Override
public long nextLong () {
return (state += 0x9E3779B97F4A7C15L);
}
/**
* Skips the state forward or backwards by the given {@code advance}, then returns the result of {@link #nextLong()}
* at the same point in the sequence. If advance is 1, this is equivalent to nextLong(). If advance is 0, this
* returns the same {@code long} as the previous call to the generator (if it called nextLong()), and doesn't change
* the state. If advance is -1, this moves the state backwards and produces the {@code long} before the last one
* generated by nextLong(). More positive numbers move the state further ahead, and more negative numbers move the
* state further behind; all of these take constant time.
*
* @param advance how many steps to advance the state before generating a {@code long}
* @return a random {@code long} by the same algorithm as {@link #nextLong()}, using the appropriately-advanced state
*/
@Override
public long skip (long advance) {
return (state += 0x9E3779B97F4A7C15L * advance);
}
@Override
public long previousLong () {
final long s = state;
state -= 0x9E3779B97F4A7C15L;
return s;
}
@Override
public int next (int bits) {
return (int) ((state += 0x9E3779B97F4A7C15L) >>> 64 - bits);
}
@Override
public int nextInt() {
return (int) ((state += 0x9E3779B97F4A7C15L) >>> 32);
}
@Override
public int nextInt(int bound) {
return (int)(bound * ((state += 0x9E3779B97F4A7C15L) >>> 32) >> 32) & ~(bound >> 31);
}
@Override
public int nextSignedInt(int outerBound) {
outerBound = (int)(outerBound * ((state += 0x9E3779B97F4A7C15L) >>> 32) >> 32);
return outerBound + (outerBound >>> 31);
}
@Override
public double nextExclusiveDouble () {
final double n = ((state += 0x9E3779B97F4A7C15L) >>> 11) * 0x1p-53;
return n == 0.0 ? 0x1.0p-54 : n;
}
@Override
public double nextExclusiveSignedDouble() {
final long bits = (state += 0x9E3779B97F4A7C15L);
final double n = (bits >>> 11) * 0x1p-53;
return Math.copySign(n == 0.0 ? 0x1.0p-54 : n, bits << 54);
}
@Override
public float nextExclusiveFloat() {
final float n = ((state += 0x9E3779B97F4A7C15L) >>> 40) * 0x1p-24f;
return n == 0f ? 0x1p-25f : n;
}
@Override
public float nextExclusiveSignedFloat() {
final long bits = (state += 0x9E3779B97F4A7C15L);
final float n = (bits >>> 40) * 0x1p-24f;
return Math.copySign(n == 0f ? 0x1p-25f : n, bits << 25);
}
@Override
public double nextGaussian() {
// return super.nextGaussian();
// return probit(nextDouble());
// return probit(((state & 0x1FFF_FFFFF_FFFFFL) ^ nextLong() >>> 11) * 0x1p-53);
return Distributor.linearNormal(state += 0x9E3779B97F4A7C15L);
// return Ziggurat.normal(state += 0x9E3779B97F4A7C15L); // has severe problems when getting tuples of Gaussians
}
@Override
public GoldenQuasiRandom copy () {
return new GoldenQuasiRandom(state);
}
@Override
public boolean equals (Object o) {
if (this == o)
return true;
if (o == null || getClass() != o.getClass())
return false;
GoldenQuasiRandom that = (GoldenQuasiRandom)o;
return state == that.state;
}
@Override
public String toString () {
return "GoldenQuasiRandom{state=" + (state) + "L}";
}
}
© 2015 - 2025 Weber Informatics LLC | Privacy Policy