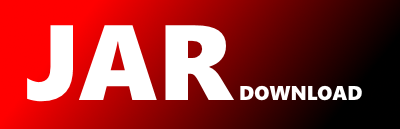
com.google.cloud.automl.v1beta1.ImageObjectDetectionModelMetadata Maven / Gradle / Ivy
Go to download
Show more of this group Show more artifacts with this name
Show all versions of proto-google-cloud-automl-v1beta1 Show documentation
Show all versions of proto-google-cloud-automl-v1beta1 Show documentation
PROTO library for proto-google-cloud-automl-v1beta1
/*
* Copyright 2020 Google LLC
*
* Licensed under the Apache License, Version 2.0 (the "License");
* you may not use this file except in compliance with the License.
* You may obtain a copy of the License at
*
* https://www.apache.org/licenses/LICENSE-2.0
*
* Unless required by applicable law or agreed to in writing, software
* distributed under the License is distributed on an "AS IS" BASIS,
* WITHOUT WARRANTIES OR CONDITIONS OF ANY KIND, either express or implied.
* See the License for the specific language governing permissions and
* limitations under the License.
*/
// Generated by the protocol buffer compiler. DO NOT EDIT!
// source: google/cloud/automl/v1beta1/image.proto
package com.google.cloud.automl.v1beta1;
/**
*
*
*
* Model metadata specific to image object detection.
*
*
* Protobuf type {@code google.cloud.automl.v1beta1.ImageObjectDetectionModelMetadata}
*/
public final class ImageObjectDetectionModelMetadata extends com.google.protobuf.GeneratedMessageV3
implements
// @@protoc_insertion_point(message_implements:google.cloud.automl.v1beta1.ImageObjectDetectionModelMetadata)
ImageObjectDetectionModelMetadataOrBuilder {
private static final long serialVersionUID = 0L;
// Use ImageObjectDetectionModelMetadata.newBuilder() to construct.
private ImageObjectDetectionModelMetadata(
com.google.protobuf.GeneratedMessageV3.Builder> builder) {
super(builder);
}
private ImageObjectDetectionModelMetadata() {
modelType_ = "";
stopReason_ = "";
}
@java.lang.Override
@SuppressWarnings({"unused"})
protected java.lang.Object newInstance(UnusedPrivateParameter unused) {
return new ImageObjectDetectionModelMetadata();
}
@java.lang.Override
public final com.google.protobuf.UnknownFieldSet getUnknownFields() {
return this.unknownFields;
}
public static final com.google.protobuf.Descriptors.Descriptor getDescriptor() {
return com.google.cloud.automl.v1beta1.ImageProto
.internal_static_google_cloud_automl_v1beta1_ImageObjectDetectionModelMetadata_descriptor;
}
@java.lang.Override
protected com.google.protobuf.GeneratedMessageV3.FieldAccessorTable
internalGetFieldAccessorTable() {
return com.google.cloud.automl.v1beta1.ImageProto
.internal_static_google_cloud_automl_v1beta1_ImageObjectDetectionModelMetadata_fieldAccessorTable
.ensureFieldAccessorsInitialized(
com.google.cloud.automl.v1beta1.ImageObjectDetectionModelMetadata.class,
com.google.cloud.automl.v1beta1.ImageObjectDetectionModelMetadata.Builder.class);
}
public static final int MODEL_TYPE_FIELD_NUMBER = 1;
private volatile java.lang.Object modelType_;
/**
*
*
*
* Optional. Type of the model. The available values are:
* * `cloud-high-accuracy-1` - (default) A model to be used via prediction
* calls to AutoML API. Expected to have a higher latency, but
* should also have a higher prediction quality than other
* models.
* * `cloud-low-latency-1` - A model to be used via prediction
* calls to AutoML API. Expected to have low latency, but may
* have lower prediction quality than other models.
* * `mobile-low-latency-1` - A model that, in addition to providing
* prediction via AutoML API, can also be exported (see
* [AutoMl.ExportModel][google.cloud.automl.v1beta1.AutoMl.ExportModel]) and used on a mobile or edge device
* with TensorFlow afterwards. Expected to have low latency, but
* may have lower prediction quality than other models.
* * `mobile-versatile-1` - A model that, in addition to providing
* prediction via AutoML API, can also be exported (see
* [AutoMl.ExportModel][google.cloud.automl.v1beta1.AutoMl.ExportModel]) and used on a mobile or edge device
* with TensorFlow afterwards.
* * `mobile-high-accuracy-1` - A model that, in addition to providing
* prediction via AutoML API, can also be exported (see
* [AutoMl.ExportModel][google.cloud.automl.v1beta1.AutoMl.ExportModel]) and used on a mobile or edge device
* with TensorFlow afterwards. Expected to have a higher
* latency, but should also have a higher prediction quality
* than other models.
*
*
* string model_type = 1;
*
* @return The modelType.
*/
@java.lang.Override
public java.lang.String getModelType() {
java.lang.Object ref = modelType_;
if (ref instanceof java.lang.String) {
return (java.lang.String) ref;
} else {
com.google.protobuf.ByteString bs = (com.google.protobuf.ByteString) ref;
java.lang.String s = bs.toStringUtf8();
modelType_ = s;
return s;
}
}
/**
*
*
*
* Optional. Type of the model. The available values are:
* * `cloud-high-accuracy-1` - (default) A model to be used via prediction
* calls to AutoML API. Expected to have a higher latency, but
* should also have a higher prediction quality than other
* models.
* * `cloud-low-latency-1` - A model to be used via prediction
* calls to AutoML API. Expected to have low latency, but may
* have lower prediction quality than other models.
* * `mobile-low-latency-1` - A model that, in addition to providing
* prediction via AutoML API, can also be exported (see
* [AutoMl.ExportModel][google.cloud.automl.v1beta1.AutoMl.ExportModel]) and used on a mobile or edge device
* with TensorFlow afterwards. Expected to have low latency, but
* may have lower prediction quality than other models.
* * `mobile-versatile-1` - A model that, in addition to providing
* prediction via AutoML API, can also be exported (see
* [AutoMl.ExportModel][google.cloud.automl.v1beta1.AutoMl.ExportModel]) and used on a mobile or edge device
* with TensorFlow afterwards.
* * `mobile-high-accuracy-1` - A model that, in addition to providing
* prediction via AutoML API, can also be exported (see
* [AutoMl.ExportModel][google.cloud.automl.v1beta1.AutoMl.ExportModel]) and used on a mobile or edge device
* with TensorFlow afterwards. Expected to have a higher
* latency, but should also have a higher prediction quality
* than other models.
*
*
* string model_type = 1;
*
* @return The bytes for modelType.
*/
@java.lang.Override
public com.google.protobuf.ByteString getModelTypeBytes() {
java.lang.Object ref = modelType_;
if (ref instanceof java.lang.String) {
com.google.protobuf.ByteString b =
com.google.protobuf.ByteString.copyFromUtf8((java.lang.String) ref);
modelType_ = b;
return b;
} else {
return (com.google.protobuf.ByteString) ref;
}
}
public static final int NODE_COUNT_FIELD_NUMBER = 3;
private long nodeCount_;
/**
*
*
*
* Output only. The number of nodes this model is deployed on. A node is an
* abstraction of a machine resource, which can handle online prediction QPS
* as given in the qps_per_node field.
*
*
* int64 node_count = 3;
*
* @return The nodeCount.
*/
@java.lang.Override
public long getNodeCount() {
return nodeCount_;
}
public static final int NODE_QPS_FIELD_NUMBER = 4;
private double nodeQps_;
/**
*
*
*
* Output only. An approximate number of online prediction QPS that can
* be supported by this model per each node on which it is deployed.
*
*
* double node_qps = 4;
*
* @return The nodeQps.
*/
@java.lang.Override
public double getNodeQps() {
return nodeQps_;
}
public static final int STOP_REASON_FIELD_NUMBER = 5;
private volatile java.lang.Object stopReason_;
/**
*
*
*
* Output only. The reason that this create model operation stopped,
* e.g. `BUDGET_REACHED`, `MODEL_CONVERGED`.
*
*
* string stop_reason = 5;
*
* @return The stopReason.
*/
@java.lang.Override
public java.lang.String getStopReason() {
java.lang.Object ref = stopReason_;
if (ref instanceof java.lang.String) {
return (java.lang.String) ref;
} else {
com.google.protobuf.ByteString bs = (com.google.protobuf.ByteString) ref;
java.lang.String s = bs.toStringUtf8();
stopReason_ = s;
return s;
}
}
/**
*
*
*
* Output only. The reason that this create model operation stopped,
* e.g. `BUDGET_REACHED`, `MODEL_CONVERGED`.
*
*
* string stop_reason = 5;
*
* @return The bytes for stopReason.
*/
@java.lang.Override
public com.google.protobuf.ByteString getStopReasonBytes() {
java.lang.Object ref = stopReason_;
if (ref instanceof java.lang.String) {
com.google.protobuf.ByteString b =
com.google.protobuf.ByteString.copyFromUtf8((java.lang.String) ref);
stopReason_ = b;
return b;
} else {
return (com.google.protobuf.ByteString) ref;
}
}
public static final int TRAIN_BUDGET_MILLI_NODE_HOURS_FIELD_NUMBER = 6;
private long trainBudgetMilliNodeHours_;
/**
*
*
*
* The train budget of creating this model, expressed in milli node
* hours i.e. 1,000 value in this field means 1 node hour. The actual
* `train_cost` will be equal or less than this value. If further model
* training ceases to provide any improvements, it will stop without using
* full budget and the stop_reason will be `MODEL_CONVERGED`.
* Note, node_hour = actual_hour * number_of_nodes_invovled.
* For model type `cloud-high-accuracy-1`(default) and `cloud-low-latency-1`,
* the train budget must be between 20,000 and 900,000 milli node hours,
* inclusive. The default value is 216, 000 which represents one day in
* wall time.
* For model type `mobile-low-latency-1`, `mobile-versatile-1`,
* `mobile-high-accuracy-1`, `mobile-core-ml-low-latency-1`,
* `mobile-core-ml-versatile-1`, `mobile-core-ml-high-accuracy-1`, the train
* budget must be between 1,000 and 100,000 milli node hours, inclusive.
* The default value is 24, 000 which represents one day in wall time.
*
*
* int64 train_budget_milli_node_hours = 6;
*
* @return The trainBudgetMilliNodeHours.
*/
@java.lang.Override
public long getTrainBudgetMilliNodeHours() {
return trainBudgetMilliNodeHours_;
}
public static final int TRAIN_COST_MILLI_NODE_HOURS_FIELD_NUMBER = 7;
private long trainCostMilliNodeHours_;
/**
*
*
*
* Output only. The actual train cost of creating this model, expressed in
* milli node hours, i.e. 1,000 value in this field means 1 node hour.
* Guaranteed to not exceed the train budget.
*
*
* int64 train_cost_milli_node_hours = 7;
*
* @return The trainCostMilliNodeHours.
*/
@java.lang.Override
public long getTrainCostMilliNodeHours() {
return trainCostMilliNodeHours_;
}
private byte memoizedIsInitialized = -1;
@java.lang.Override
public final boolean isInitialized() {
byte isInitialized = memoizedIsInitialized;
if (isInitialized == 1) return true;
if (isInitialized == 0) return false;
memoizedIsInitialized = 1;
return true;
}
@java.lang.Override
public void writeTo(com.google.protobuf.CodedOutputStream output) throws java.io.IOException {
if (!com.google.protobuf.GeneratedMessageV3.isStringEmpty(modelType_)) {
com.google.protobuf.GeneratedMessageV3.writeString(output, 1, modelType_);
}
if (nodeCount_ != 0L) {
output.writeInt64(3, nodeCount_);
}
if (java.lang.Double.doubleToRawLongBits(nodeQps_) != 0) {
output.writeDouble(4, nodeQps_);
}
if (!com.google.protobuf.GeneratedMessageV3.isStringEmpty(stopReason_)) {
com.google.protobuf.GeneratedMessageV3.writeString(output, 5, stopReason_);
}
if (trainBudgetMilliNodeHours_ != 0L) {
output.writeInt64(6, trainBudgetMilliNodeHours_);
}
if (trainCostMilliNodeHours_ != 0L) {
output.writeInt64(7, trainCostMilliNodeHours_);
}
getUnknownFields().writeTo(output);
}
@java.lang.Override
public int getSerializedSize() {
int size = memoizedSize;
if (size != -1) return size;
size = 0;
if (!com.google.protobuf.GeneratedMessageV3.isStringEmpty(modelType_)) {
size += com.google.protobuf.GeneratedMessageV3.computeStringSize(1, modelType_);
}
if (nodeCount_ != 0L) {
size += com.google.protobuf.CodedOutputStream.computeInt64Size(3, nodeCount_);
}
if (java.lang.Double.doubleToRawLongBits(nodeQps_) != 0) {
size += com.google.protobuf.CodedOutputStream.computeDoubleSize(4, nodeQps_);
}
if (!com.google.protobuf.GeneratedMessageV3.isStringEmpty(stopReason_)) {
size += com.google.protobuf.GeneratedMessageV3.computeStringSize(5, stopReason_);
}
if (trainBudgetMilliNodeHours_ != 0L) {
size += com.google.protobuf.CodedOutputStream.computeInt64Size(6, trainBudgetMilliNodeHours_);
}
if (trainCostMilliNodeHours_ != 0L) {
size += com.google.protobuf.CodedOutputStream.computeInt64Size(7, trainCostMilliNodeHours_);
}
size += getUnknownFields().getSerializedSize();
memoizedSize = size;
return size;
}
@java.lang.Override
public boolean equals(final java.lang.Object obj) {
if (obj == this) {
return true;
}
if (!(obj instanceof com.google.cloud.automl.v1beta1.ImageObjectDetectionModelMetadata)) {
return super.equals(obj);
}
com.google.cloud.automl.v1beta1.ImageObjectDetectionModelMetadata other =
(com.google.cloud.automl.v1beta1.ImageObjectDetectionModelMetadata) obj;
if (!getModelType().equals(other.getModelType())) return false;
if (getNodeCount() != other.getNodeCount()) return false;
if (java.lang.Double.doubleToLongBits(getNodeQps())
!= java.lang.Double.doubleToLongBits(other.getNodeQps())) return false;
if (!getStopReason().equals(other.getStopReason())) return false;
if (getTrainBudgetMilliNodeHours() != other.getTrainBudgetMilliNodeHours()) return false;
if (getTrainCostMilliNodeHours() != other.getTrainCostMilliNodeHours()) return false;
if (!getUnknownFields().equals(other.getUnknownFields())) return false;
return true;
}
@java.lang.Override
public int hashCode() {
if (memoizedHashCode != 0) {
return memoizedHashCode;
}
int hash = 41;
hash = (19 * hash) + getDescriptor().hashCode();
hash = (37 * hash) + MODEL_TYPE_FIELD_NUMBER;
hash = (53 * hash) + getModelType().hashCode();
hash = (37 * hash) + NODE_COUNT_FIELD_NUMBER;
hash = (53 * hash) + com.google.protobuf.Internal.hashLong(getNodeCount());
hash = (37 * hash) + NODE_QPS_FIELD_NUMBER;
hash =
(53 * hash)
+ com.google.protobuf.Internal.hashLong(
java.lang.Double.doubleToLongBits(getNodeQps()));
hash = (37 * hash) + STOP_REASON_FIELD_NUMBER;
hash = (53 * hash) + getStopReason().hashCode();
hash = (37 * hash) + TRAIN_BUDGET_MILLI_NODE_HOURS_FIELD_NUMBER;
hash = (53 * hash) + com.google.protobuf.Internal.hashLong(getTrainBudgetMilliNodeHours());
hash = (37 * hash) + TRAIN_COST_MILLI_NODE_HOURS_FIELD_NUMBER;
hash = (53 * hash) + com.google.protobuf.Internal.hashLong(getTrainCostMilliNodeHours());
hash = (29 * hash) + getUnknownFields().hashCode();
memoizedHashCode = hash;
return hash;
}
public static com.google.cloud.automl.v1beta1.ImageObjectDetectionModelMetadata parseFrom(
java.nio.ByteBuffer data) throws com.google.protobuf.InvalidProtocolBufferException {
return PARSER.parseFrom(data);
}
public static com.google.cloud.automl.v1beta1.ImageObjectDetectionModelMetadata parseFrom(
java.nio.ByteBuffer data, com.google.protobuf.ExtensionRegistryLite extensionRegistry)
throws com.google.protobuf.InvalidProtocolBufferException {
return PARSER.parseFrom(data, extensionRegistry);
}
public static com.google.cloud.automl.v1beta1.ImageObjectDetectionModelMetadata parseFrom(
com.google.protobuf.ByteString data)
throws com.google.protobuf.InvalidProtocolBufferException {
return PARSER.parseFrom(data);
}
public static com.google.cloud.automl.v1beta1.ImageObjectDetectionModelMetadata parseFrom(
com.google.protobuf.ByteString data,
com.google.protobuf.ExtensionRegistryLite extensionRegistry)
throws com.google.protobuf.InvalidProtocolBufferException {
return PARSER.parseFrom(data, extensionRegistry);
}
public static com.google.cloud.automl.v1beta1.ImageObjectDetectionModelMetadata parseFrom(
byte[] data) throws com.google.protobuf.InvalidProtocolBufferException {
return PARSER.parseFrom(data);
}
public static com.google.cloud.automl.v1beta1.ImageObjectDetectionModelMetadata parseFrom(
byte[] data, com.google.protobuf.ExtensionRegistryLite extensionRegistry)
throws com.google.protobuf.InvalidProtocolBufferException {
return PARSER.parseFrom(data, extensionRegistry);
}
public static com.google.cloud.automl.v1beta1.ImageObjectDetectionModelMetadata parseFrom(
java.io.InputStream input) throws java.io.IOException {
return com.google.protobuf.GeneratedMessageV3.parseWithIOException(PARSER, input);
}
public static com.google.cloud.automl.v1beta1.ImageObjectDetectionModelMetadata parseFrom(
java.io.InputStream input, com.google.protobuf.ExtensionRegistryLite extensionRegistry)
throws java.io.IOException {
return com.google.protobuf.GeneratedMessageV3.parseWithIOException(
PARSER, input, extensionRegistry);
}
public static com.google.cloud.automl.v1beta1.ImageObjectDetectionModelMetadata
parseDelimitedFrom(java.io.InputStream input) throws java.io.IOException {
return com.google.protobuf.GeneratedMessageV3.parseDelimitedWithIOException(PARSER, input);
}
public static com.google.cloud.automl.v1beta1.ImageObjectDetectionModelMetadata
parseDelimitedFrom(
java.io.InputStream input, com.google.protobuf.ExtensionRegistryLite extensionRegistry)
throws java.io.IOException {
return com.google.protobuf.GeneratedMessageV3.parseDelimitedWithIOException(
PARSER, input, extensionRegistry);
}
public static com.google.cloud.automl.v1beta1.ImageObjectDetectionModelMetadata parseFrom(
com.google.protobuf.CodedInputStream input) throws java.io.IOException {
return com.google.protobuf.GeneratedMessageV3.parseWithIOException(PARSER, input);
}
public static com.google.cloud.automl.v1beta1.ImageObjectDetectionModelMetadata parseFrom(
com.google.protobuf.CodedInputStream input,
com.google.protobuf.ExtensionRegistryLite extensionRegistry)
throws java.io.IOException {
return com.google.protobuf.GeneratedMessageV3.parseWithIOException(
PARSER, input, extensionRegistry);
}
@java.lang.Override
public Builder newBuilderForType() {
return newBuilder();
}
public static Builder newBuilder() {
return DEFAULT_INSTANCE.toBuilder();
}
public static Builder newBuilder(
com.google.cloud.automl.v1beta1.ImageObjectDetectionModelMetadata prototype) {
return DEFAULT_INSTANCE.toBuilder().mergeFrom(prototype);
}
@java.lang.Override
public Builder toBuilder() {
return this == DEFAULT_INSTANCE ? new Builder() : new Builder().mergeFrom(this);
}
@java.lang.Override
protected Builder newBuilderForType(com.google.protobuf.GeneratedMessageV3.BuilderParent parent) {
Builder builder = new Builder(parent);
return builder;
}
/**
*
*
*
* Model metadata specific to image object detection.
*
*
* Protobuf type {@code google.cloud.automl.v1beta1.ImageObjectDetectionModelMetadata}
*/
public static final class Builder extends com.google.protobuf.GeneratedMessageV3.Builder
implements
// @@protoc_insertion_point(builder_implements:google.cloud.automl.v1beta1.ImageObjectDetectionModelMetadata)
com.google.cloud.automl.v1beta1.ImageObjectDetectionModelMetadataOrBuilder {
public static final com.google.protobuf.Descriptors.Descriptor getDescriptor() {
return com.google.cloud.automl.v1beta1.ImageProto
.internal_static_google_cloud_automl_v1beta1_ImageObjectDetectionModelMetadata_descriptor;
}
@java.lang.Override
protected com.google.protobuf.GeneratedMessageV3.FieldAccessorTable
internalGetFieldAccessorTable() {
return com.google.cloud.automl.v1beta1.ImageProto
.internal_static_google_cloud_automl_v1beta1_ImageObjectDetectionModelMetadata_fieldAccessorTable
.ensureFieldAccessorsInitialized(
com.google.cloud.automl.v1beta1.ImageObjectDetectionModelMetadata.class,
com.google.cloud.automl.v1beta1.ImageObjectDetectionModelMetadata.Builder.class);
}
// Construct using
// com.google.cloud.automl.v1beta1.ImageObjectDetectionModelMetadata.newBuilder()
private Builder() {}
private Builder(com.google.protobuf.GeneratedMessageV3.BuilderParent parent) {
super(parent);
}
@java.lang.Override
public Builder clear() {
super.clear();
modelType_ = "";
nodeCount_ = 0L;
nodeQps_ = 0D;
stopReason_ = "";
trainBudgetMilliNodeHours_ = 0L;
trainCostMilliNodeHours_ = 0L;
return this;
}
@java.lang.Override
public com.google.protobuf.Descriptors.Descriptor getDescriptorForType() {
return com.google.cloud.automl.v1beta1.ImageProto
.internal_static_google_cloud_automl_v1beta1_ImageObjectDetectionModelMetadata_descriptor;
}
@java.lang.Override
public com.google.cloud.automl.v1beta1.ImageObjectDetectionModelMetadata
getDefaultInstanceForType() {
return com.google.cloud.automl.v1beta1.ImageObjectDetectionModelMetadata.getDefaultInstance();
}
@java.lang.Override
public com.google.cloud.automl.v1beta1.ImageObjectDetectionModelMetadata build() {
com.google.cloud.automl.v1beta1.ImageObjectDetectionModelMetadata result = buildPartial();
if (!result.isInitialized()) {
throw newUninitializedMessageException(result);
}
return result;
}
@java.lang.Override
public com.google.cloud.automl.v1beta1.ImageObjectDetectionModelMetadata buildPartial() {
com.google.cloud.automl.v1beta1.ImageObjectDetectionModelMetadata result =
new com.google.cloud.automl.v1beta1.ImageObjectDetectionModelMetadata(this);
result.modelType_ = modelType_;
result.nodeCount_ = nodeCount_;
result.nodeQps_ = nodeQps_;
result.stopReason_ = stopReason_;
result.trainBudgetMilliNodeHours_ = trainBudgetMilliNodeHours_;
result.trainCostMilliNodeHours_ = trainCostMilliNodeHours_;
onBuilt();
return result;
}
@java.lang.Override
public Builder clone() {
return super.clone();
}
@java.lang.Override
public Builder setField(
com.google.protobuf.Descriptors.FieldDescriptor field, java.lang.Object value) {
return super.setField(field, value);
}
@java.lang.Override
public Builder clearField(com.google.protobuf.Descriptors.FieldDescriptor field) {
return super.clearField(field);
}
@java.lang.Override
public Builder clearOneof(com.google.protobuf.Descriptors.OneofDescriptor oneof) {
return super.clearOneof(oneof);
}
@java.lang.Override
public Builder setRepeatedField(
com.google.protobuf.Descriptors.FieldDescriptor field, int index, java.lang.Object value) {
return super.setRepeatedField(field, index, value);
}
@java.lang.Override
public Builder addRepeatedField(
com.google.protobuf.Descriptors.FieldDescriptor field, java.lang.Object value) {
return super.addRepeatedField(field, value);
}
@java.lang.Override
public Builder mergeFrom(com.google.protobuf.Message other) {
if (other instanceof com.google.cloud.automl.v1beta1.ImageObjectDetectionModelMetadata) {
return mergeFrom((com.google.cloud.automl.v1beta1.ImageObjectDetectionModelMetadata) other);
} else {
super.mergeFrom(other);
return this;
}
}
public Builder mergeFrom(
com.google.cloud.automl.v1beta1.ImageObjectDetectionModelMetadata other) {
if (other
== com.google.cloud.automl.v1beta1.ImageObjectDetectionModelMetadata.getDefaultInstance())
return this;
if (!other.getModelType().isEmpty()) {
modelType_ = other.modelType_;
onChanged();
}
if (other.getNodeCount() != 0L) {
setNodeCount(other.getNodeCount());
}
if (other.getNodeQps() != 0D) {
setNodeQps(other.getNodeQps());
}
if (!other.getStopReason().isEmpty()) {
stopReason_ = other.stopReason_;
onChanged();
}
if (other.getTrainBudgetMilliNodeHours() != 0L) {
setTrainBudgetMilliNodeHours(other.getTrainBudgetMilliNodeHours());
}
if (other.getTrainCostMilliNodeHours() != 0L) {
setTrainCostMilliNodeHours(other.getTrainCostMilliNodeHours());
}
this.mergeUnknownFields(other.getUnknownFields());
onChanged();
return this;
}
@java.lang.Override
public final boolean isInitialized() {
return true;
}
@java.lang.Override
public Builder mergeFrom(
com.google.protobuf.CodedInputStream input,
com.google.protobuf.ExtensionRegistryLite extensionRegistry)
throws java.io.IOException {
if (extensionRegistry == null) {
throw new java.lang.NullPointerException();
}
try {
boolean done = false;
while (!done) {
int tag = input.readTag();
switch (tag) {
case 0:
done = true;
break;
case 10:
{
modelType_ = input.readStringRequireUtf8();
break;
} // case 10
case 24:
{
nodeCount_ = input.readInt64();
break;
} // case 24
case 33:
{
nodeQps_ = input.readDouble();
break;
} // case 33
case 42:
{
stopReason_ = input.readStringRequireUtf8();
break;
} // case 42
case 48:
{
trainBudgetMilliNodeHours_ = input.readInt64();
break;
} // case 48
case 56:
{
trainCostMilliNodeHours_ = input.readInt64();
break;
} // case 56
default:
{
if (!super.parseUnknownField(input, extensionRegistry, tag)) {
done = true; // was an endgroup tag
}
break;
} // default:
} // switch (tag)
} // while (!done)
} catch (com.google.protobuf.InvalidProtocolBufferException e) {
throw e.unwrapIOException();
} finally {
onChanged();
} // finally
return this;
}
private java.lang.Object modelType_ = "";
/**
*
*
*
* Optional. Type of the model. The available values are:
* * `cloud-high-accuracy-1` - (default) A model to be used via prediction
* calls to AutoML API. Expected to have a higher latency, but
* should also have a higher prediction quality than other
* models.
* * `cloud-low-latency-1` - A model to be used via prediction
* calls to AutoML API. Expected to have low latency, but may
* have lower prediction quality than other models.
* * `mobile-low-latency-1` - A model that, in addition to providing
* prediction via AutoML API, can also be exported (see
* [AutoMl.ExportModel][google.cloud.automl.v1beta1.AutoMl.ExportModel]) and used on a mobile or edge device
* with TensorFlow afterwards. Expected to have low latency, but
* may have lower prediction quality than other models.
* * `mobile-versatile-1` - A model that, in addition to providing
* prediction via AutoML API, can also be exported (see
* [AutoMl.ExportModel][google.cloud.automl.v1beta1.AutoMl.ExportModel]) and used on a mobile or edge device
* with TensorFlow afterwards.
* * `mobile-high-accuracy-1` - A model that, in addition to providing
* prediction via AutoML API, can also be exported (see
* [AutoMl.ExportModel][google.cloud.automl.v1beta1.AutoMl.ExportModel]) and used on a mobile or edge device
* with TensorFlow afterwards. Expected to have a higher
* latency, but should also have a higher prediction quality
* than other models.
*
*
* string model_type = 1;
*
* @return The modelType.
*/
public java.lang.String getModelType() {
java.lang.Object ref = modelType_;
if (!(ref instanceof java.lang.String)) {
com.google.protobuf.ByteString bs = (com.google.protobuf.ByteString) ref;
java.lang.String s = bs.toStringUtf8();
modelType_ = s;
return s;
} else {
return (java.lang.String) ref;
}
}
/**
*
*
*
* Optional. Type of the model. The available values are:
* * `cloud-high-accuracy-1` - (default) A model to be used via prediction
* calls to AutoML API. Expected to have a higher latency, but
* should also have a higher prediction quality than other
* models.
* * `cloud-low-latency-1` - A model to be used via prediction
* calls to AutoML API. Expected to have low latency, but may
* have lower prediction quality than other models.
* * `mobile-low-latency-1` - A model that, in addition to providing
* prediction via AutoML API, can also be exported (see
* [AutoMl.ExportModel][google.cloud.automl.v1beta1.AutoMl.ExportModel]) and used on a mobile or edge device
* with TensorFlow afterwards. Expected to have low latency, but
* may have lower prediction quality than other models.
* * `mobile-versatile-1` - A model that, in addition to providing
* prediction via AutoML API, can also be exported (see
* [AutoMl.ExportModel][google.cloud.automl.v1beta1.AutoMl.ExportModel]) and used on a mobile or edge device
* with TensorFlow afterwards.
* * `mobile-high-accuracy-1` - A model that, in addition to providing
* prediction via AutoML API, can also be exported (see
* [AutoMl.ExportModel][google.cloud.automl.v1beta1.AutoMl.ExportModel]) and used on a mobile or edge device
* with TensorFlow afterwards. Expected to have a higher
* latency, but should also have a higher prediction quality
* than other models.
*
*
* string model_type = 1;
*
* @return The bytes for modelType.
*/
public com.google.protobuf.ByteString getModelTypeBytes() {
java.lang.Object ref = modelType_;
if (ref instanceof String) {
com.google.protobuf.ByteString b =
com.google.protobuf.ByteString.copyFromUtf8((java.lang.String) ref);
modelType_ = b;
return b;
} else {
return (com.google.protobuf.ByteString) ref;
}
}
/**
*
*
*
* Optional. Type of the model. The available values are:
* * `cloud-high-accuracy-1` - (default) A model to be used via prediction
* calls to AutoML API. Expected to have a higher latency, but
* should also have a higher prediction quality than other
* models.
* * `cloud-low-latency-1` - A model to be used via prediction
* calls to AutoML API. Expected to have low latency, but may
* have lower prediction quality than other models.
* * `mobile-low-latency-1` - A model that, in addition to providing
* prediction via AutoML API, can also be exported (see
* [AutoMl.ExportModel][google.cloud.automl.v1beta1.AutoMl.ExportModel]) and used on a mobile or edge device
* with TensorFlow afterwards. Expected to have low latency, but
* may have lower prediction quality than other models.
* * `mobile-versatile-1` - A model that, in addition to providing
* prediction via AutoML API, can also be exported (see
* [AutoMl.ExportModel][google.cloud.automl.v1beta1.AutoMl.ExportModel]) and used on a mobile or edge device
* with TensorFlow afterwards.
* * `mobile-high-accuracy-1` - A model that, in addition to providing
* prediction via AutoML API, can also be exported (see
* [AutoMl.ExportModel][google.cloud.automl.v1beta1.AutoMl.ExportModel]) and used on a mobile or edge device
* with TensorFlow afterwards. Expected to have a higher
* latency, but should also have a higher prediction quality
* than other models.
*
*
* string model_type = 1;
*
* @param value The modelType to set.
* @return This builder for chaining.
*/
public Builder setModelType(java.lang.String value) {
if (value == null) {
throw new NullPointerException();
}
modelType_ = value;
onChanged();
return this;
}
/**
*
*
*
* Optional. Type of the model. The available values are:
* * `cloud-high-accuracy-1` - (default) A model to be used via prediction
* calls to AutoML API. Expected to have a higher latency, but
* should also have a higher prediction quality than other
* models.
* * `cloud-low-latency-1` - A model to be used via prediction
* calls to AutoML API. Expected to have low latency, but may
* have lower prediction quality than other models.
* * `mobile-low-latency-1` - A model that, in addition to providing
* prediction via AutoML API, can also be exported (see
* [AutoMl.ExportModel][google.cloud.automl.v1beta1.AutoMl.ExportModel]) and used on a mobile or edge device
* with TensorFlow afterwards. Expected to have low latency, but
* may have lower prediction quality than other models.
* * `mobile-versatile-1` - A model that, in addition to providing
* prediction via AutoML API, can also be exported (see
* [AutoMl.ExportModel][google.cloud.automl.v1beta1.AutoMl.ExportModel]) and used on a mobile or edge device
* with TensorFlow afterwards.
* * `mobile-high-accuracy-1` - A model that, in addition to providing
* prediction via AutoML API, can also be exported (see
* [AutoMl.ExportModel][google.cloud.automl.v1beta1.AutoMl.ExportModel]) and used on a mobile or edge device
* with TensorFlow afterwards. Expected to have a higher
* latency, but should also have a higher prediction quality
* than other models.
*
*
* string model_type = 1;
*
* @return This builder for chaining.
*/
public Builder clearModelType() {
modelType_ = getDefaultInstance().getModelType();
onChanged();
return this;
}
/**
*
*
*
* Optional. Type of the model. The available values are:
* * `cloud-high-accuracy-1` - (default) A model to be used via prediction
* calls to AutoML API. Expected to have a higher latency, but
* should also have a higher prediction quality than other
* models.
* * `cloud-low-latency-1` - A model to be used via prediction
* calls to AutoML API. Expected to have low latency, but may
* have lower prediction quality than other models.
* * `mobile-low-latency-1` - A model that, in addition to providing
* prediction via AutoML API, can also be exported (see
* [AutoMl.ExportModel][google.cloud.automl.v1beta1.AutoMl.ExportModel]) and used on a mobile or edge device
* with TensorFlow afterwards. Expected to have low latency, but
* may have lower prediction quality than other models.
* * `mobile-versatile-1` - A model that, in addition to providing
* prediction via AutoML API, can also be exported (see
* [AutoMl.ExportModel][google.cloud.automl.v1beta1.AutoMl.ExportModel]) and used on a mobile or edge device
* with TensorFlow afterwards.
* * `mobile-high-accuracy-1` - A model that, in addition to providing
* prediction via AutoML API, can also be exported (see
* [AutoMl.ExportModel][google.cloud.automl.v1beta1.AutoMl.ExportModel]) and used on a mobile or edge device
* with TensorFlow afterwards. Expected to have a higher
* latency, but should also have a higher prediction quality
* than other models.
*
*
* string model_type = 1;
*
* @param value The bytes for modelType to set.
* @return This builder for chaining.
*/
public Builder setModelTypeBytes(com.google.protobuf.ByteString value) {
if (value == null) {
throw new NullPointerException();
}
checkByteStringIsUtf8(value);
modelType_ = value;
onChanged();
return this;
}
private long nodeCount_;
/**
*
*
*
* Output only. The number of nodes this model is deployed on. A node is an
* abstraction of a machine resource, which can handle online prediction QPS
* as given in the qps_per_node field.
*
*
* int64 node_count = 3;
*
* @return The nodeCount.
*/
@java.lang.Override
public long getNodeCount() {
return nodeCount_;
}
/**
*
*
*
* Output only. The number of nodes this model is deployed on. A node is an
* abstraction of a machine resource, which can handle online prediction QPS
* as given in the qps_per_node field.
*
*
* int64 node_count = 3;
*
* @param value The nodeCount to set.
* @return This builder for chaining.
*/
public Builder setNodeCount(long value) {
nodeCount_ = value;
onChanged();
return this;
}
/**
*
*
*
* Output only. The number of nodes this model is deployed on. A node is an
* abstraction of a machine resource, which can handle online prediction QPS
* as given in the qps_per_node field.
*
*
* int64 node_count = 3;
*
* @return This builder for chaining.
*/
public Builder clearNodeCount() {
nodeCount_ = 0L;
onChanged();
return this;
}
private double nodeQps_;
/**
*
*
*
* Output only. An approximate number of online prediction QPS that can
* be supported by this model per each node on which it is deployed.
*
*
* double node_qps = 4;
*
* @return The nodeQps.
*/
@java.lang.Override
public double getNodeQps() {
return nodeQps_;
}
/**
*
*
*
* Output only. An approximate number of online prediction QPS that can
* be supported by this model per each node on which it is deployed.
*
*
* double node_qps = 4;
*
* @param value The nodeQps to set.
* @return This builder for chaining.
*/
public Builder setNodeQps(double value) {
nodeQps_ = value;
onChanged();
return this;
}
/**
*
*
*
* Output only. An approximate number of online prediction QPS that can
* be supported by this model per each node on which it is deployed.
*
*
* double node_qps = 4;
*
* @return This builder for chaining.
*/
public Builder clearNodeQps() {
nodeQps_ = 0D;
onChanged();
return this;
}
private java.lang.Object stopReason_ = "";
/**
*
*
*
* Output only. The reason that this create model operation stopped,
* e.g. `BUDGET_REACHED`, `MODEL_CONVERGED`.
*
*
* string stop_reason = 5;
*
* @return The stopReason.
*/
public java.lang.String getStopReason() {
java.lang.Object ref = stopReason_;
if (!(ref instanceof java.lang.String)) {
com.google.protobuf.ByteString bs = (com.google.protobuf.ByteString) ref;
java.lang.String s = bs.toStringUtf8();
stopReason_ = s;
return s;
} else {
return (java.lang.String) ref;
}
}
/**
*
*
*
* Output only. The reason that this create model operation stopped,
* e.g. `BUDGET_REACHED`, `MODEL_CONVERGED`.
*
*
* string stop_reason = 5;
*
* @return The bytes for stopReason.
*/
public com.google.protobuf.ByteString getStopReasonBytes() {
java.lang.Object ref = stopReason_;
if (ref instanceof String) {
com.google.protobuf.ByteString b =
com.google.protobuf.ByteString.copyFromUtf8((java.lang.String) ref);
stopReason_ = b;
return b;
} else {
return (com.google.protobuf.ByteString) ref;
}
}
/**
*
*
*
* Output only. The reason that this create model operation stopped,
* e.g. `BUDGET_REACHED`, `MODEL_CONVERGED`.
*
*
* string stop_reason = 5;
*
* @param value The stopReason to set.
* @return This builder for chaining.
*/
public Builder setStopReason(java.lang.String value) {
if (value == null) {
throw new NullPointerException();
}
stopReason_ = value;
onChanged();
return this;
}
/**
*
*
*
* Output only. The reason that this create model operation stopped,
* e.g. `BUDGET_REACHED`, `MODEL_CONVERGED`.
*
*
* string stop_reason = 5;
*
* @return This builder for chaining.
*/
public Builder clearStopReason() {
stopReason_ = getDefaultInstance().getStopReason();
onChanged();
return this;
}
/**
*
*
*
* Output only. The reason that this create model operation stopped,
* e.g. `BUDGET_REACHED`, `MODEL_CONVERGED`.
*
*
* string stop_reason = 5;
*
* @param value The bytes for stopReason to set.
* @return This builder for chaining.
*/
public Builder setStopReasonBytes(com.google.protobuf.ByteString value) {
if (value == null) {
throw new NullPointerException();
}
checkByteStringIsUtf8(value);
stopReason_ = value;
onChanged();
return this;
}
private long trainBudgetMilliNodeHours_;
/**
*
*
*
* The train budget of creating this model, expressed in milli node
* hours i.e. 1,000 value in this field means 1 node hour. The actual
* `train_cost` will be equal or less than this value. If further model
* training ceases to provide any improvements, it will stop without using
* full budget and the stop_reason will be `MODEL_CONVERGED`.
* Note, node_hour = actual_hour * number_of_nodes_invovled.
* For model type `cloud-high-accuracy-1`(default) and `cloud-low-latency-1`,
* the train budget must be between 20,000 and 900,000 milli node hours,
* inclusive. The default value is 216, 000 which represents one day in
* wall time.
* For model type `mobile-low-latency-1`, `mobile-versatile-1`,
* `mobile-high-accuracy-1`, `mobile-core-ml-low-latency-1`,
* `mobile-core-ml-versatile-1`, `mobile-core-ml-high-accuracy-1`, the train
* budget must be between 1,000 and 100,000 milli node hours, inclusive.
* The default value is 24, 000 which represents one day in wall time.
*
*
* int64 train_budget_milli_node_hours = 6;
*
* @return The trainBudgetMilliNodeHours.
*/
@java.lang.Override
public long getTrainBudgetMilliNodeHours() {
return trainBudgetMilliNodeHours_;
}
/**
*
*
*
* The train budget of creating this model, expressed in milli node
* hours i.e. 1,000 value in this field means 1 node hour. The actual
* `train_cost` will be equal or less than this value. If further model
* training ceases to provide any improvements, it will stop without using
* full budget and the stop_reason will be `MODEL_CONVERGED`.
* Note, node_hour = actual_hour * number_of_nodes_invovled.
* For model type `cloud-high-accuracy-1`(default) and `cloud-low-latency-1`,
* the train budget must be between 20,000 and 900,000 milli node hours,
* inclusive. The default value is 216, 000 which represents one day in
* wall time.
* For model type `mobile-low-latency-1`, `mobile-versatile-1`,
* `mobile-high-accuracy-1`, `mobile-core-ml-low-latency-1`,
* `mobile-core-ml-versatile-1`, `mobile-core-ml-high-accuracy-1`, the train
* budget must be between 1,000 and 100,000 milli node hours, inclusive.
* The default value is 24, 000 which represents one day in wall time.
*
*
* int64 train_budget_milli_node_hours = 6;
*
* @param value The trainBudgetMilliNodeHours to set.
* @return This builder for chaining.
*/
public Builder setTrainBudgetMilliNodeHours(long value) {
trainBudgetMilliNodeHours_ = value;
onChanged();
return this;
}
/**
*
*
*
* The train budget of creating this model, expressed in milli node
* hours i.e. 1,000 value in this field means 1 node hour. The actual
* `train_cost` will be equal or less than this value. If further model
* training ceases to provide any improvements, it will stop without using
* full budget and the stop_reason will be `MODEL_CONVERGED`.
* Note, node_hour = actual_hour * number_of_nodes_invovled.
* For model type `cloud-high-accuracy-1`(default) and `cloud-low-latency-1`,
* the train budget must be between 20,000 and 900,000 milli node hours,
* inclusive. The default value is 216, 000 which represents one day in
* wall time.
* For model type `mobile-low-latency-1`, `mobile-versatile-1`,
* `mobile-high-accuracy-1`, `mobile-core-ml-low-latency-1`,
* `mobile-core-ml-versatile-1`, `mobile-core-ml-high-accuracy-1`, the train
* budget must be between 1,000 and 100,000 milli node hours, inclusive.
* The default value is 24, 000 which represents one day in wall time.
*
*
* int64 train_budget_milli_node_hours = 6;
*
* @return This builder for chaining.
*/
public Builder clearTrainBudgetMilliNodeHours() {
trainBudgetMilliNodeHours_ = 0L;
onChanged();
return this;
}
private long trainCostMilliNodeHours_;
/**
*
*
*
* Output only. The actual train cost of creating this model, expressed in
* milli node hours, i.e. 1,000 value in this field means 1 node hour.
* Guaranteed to not exceed the train budget.
*
*
* int64 train_cost_milli_node_hours = 7;
*
* @return The trainCostMilliNodeHours.
*/
@java.lang.Override
public long getTrainCostMilliNodeHours() {
return trainCostMilliNodeHours_;
}
/**
*
*
*
* Output only. The actual train cost of creating this model, expressed in
* milli node hours, i.e. 1,000 value in this field means 1 node hour.
* Guaranteed to not exceed the train budget.
*
*
* int64 train_cost_milli_node_hours = 7;
*
* @param value The trainCostMilliNodeHours to set.
* @return This builder for chaining.
*/
public Builder setTrainCostMilliNodeHours(long value) {
trainCostMilliNodeHours_ = value;
onChanged();
return this;
}
/**
*
*
*
* Output only. The actual train cost of creating this model, expressed in
* milli node hours, i.e. 1,000 value in this field means 1 node hour.
* Guaranteed to not exceed the train budget.
*
*
* int64 train_cost_milli_node_hours = 7;
*
* @return This builder for chaining.
*/
public Builder clearTrainCostMilliNodeHours() {
trainCostMilliNodeHours_ = 0L;
onChanged();
return this;
}
@java.lang.Override
public final Builder setUnknownFields(final com.google.protobuf.UnknownFieldSet unknownFields) {
return super.setUnknownFields(unknownFields);
}
@java.lang.Override
public final Builder mergeUnknownFields(
final com.google.protobuf.UnknownFieldSet unknownFields) {
return super.mergeUnknownFields(unknownFields);
}
// @@protoc_insertion_point(builder_scope:google.cloud.automl.v1beta1.ImageObjectDetectionModelMetadata)
}
// @@protoc_insertion_point(class_scope:google.cloud.automl.v1beta1.ImageObjectDetectionModelMetadata)
private static final com.google.cloud.automl.v1beta1.ImageObjectDetectionModelMetadata
DEFAULT_INSTANCE;
static {
DEFAULT_INSTANCE = new com.google.cloud.automl.v1beta1.ImageObjectDetectionModelMetadata();
}
public static com.google.cloud.automl.v1beta1.ImageObjectDetectionModelMetadata
getDefaultInstance() {
return DEFAULT_INSTANCE;
}
private static final com.google.protobuf.Parser PARSER =
new com.google.protobuf.AbstractParser() {
@java.lang.Override
public ImageObjectDetectionModelMetadata parsePartialFrom(
com.google.protobuf.CodedInputStream input,
com.google.protobuf.ExtensionRegistryLite extensionRegistry)
throws com.google.protobuf.InvalidProtocolBufferException {
Builder builder = newBuilder();
try {
builder.mergeFrom(input, extensionRegistry);
} catch (com.google.protobuf.InvalidProtocolBufferException e) {
throw e.setUnfinishedMessage(builder.buildPartial());
} catch (com.google.protobuf.UninitializedMessageException e) {
throw e.asInvalidProtocolBufferException().setUnfinishedMessage(builder.buildPartial());
} catch (java.io.IOException e) {
throw new com.google.protobuf.InvalidProtocolBufferException(e)
.setUnfinishedMessage(builder.buildPartial());
}
return builder.buildPartial();
}
};
public static com.google.protobuf.Parser parser() {
return PARSER;
}
@java.lang.Override
public com.google.protobuf.Parser getParserForType() {
return PARSER;
}
@java.lang.Override
public com.google.cloud.automl.v1beta1.ImageObjectDetectionModelMetadata
getDefaultInstanceForType() {
return DEFAULT_INSTANCE;
}
}
© 2015 - 2025 Weber Informatics LLC | Privacy Policy