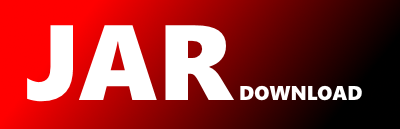
com.google.cloud.automl.v1beta1.TablesModelMetadata Maven / Gradle / Ivy
Go to download
Show more of this group Show more artifacts with this name
Show all versions of proto-google-cloud-automl-v1beta1 Show documentation
Show all versions of proto-google-cloud-automl-v1beta1 Show documentation
PROTO library for proto-google-cloud-automl-v1beta1
/*
* Copyright 2020 Google LLC
*
* Licensed under the Apache License, Version 2.0 (the "License");
* you may not use this file except in compliance with the License.
* You may obtain a copy of the License at
*
* https://www.apache.org/licenses/LICENSE-2.0
*
* Unless required by applicable law or agreed to in writing, software
* distributed under the License is distributed on an "AS IS" BASIS,
* WITHOUT WARRANTIES OR CONDITIONS OF ANY KIND, either express or implied.
* See the License for the specific language governing permissions and
* limitations under the License.
*/
// Generated by the protocol buffer compiler. DO NOT EDIT!
// source: google/cloud/automl/v1beta1/tables.proto
package com.google.cloud.automl.v1beta1;
/**
*
*
*
* Model metadata specific to AutoML Tables.
*
*
* Protobuf type {@code google.cloud.automl.v1beta1.TablesModelMetadata}
*/
public final class TablesModelMetadata extends com.google.protobuf.GeneratedMessageV3
implements
// @@protoc_insertion_point(message_implements:google.cloud.automl.v1beta1.TablesModelMetadata)
TablesModelMetadataOrBuilder {
private static final long serialVersionUID = 0L;
// Use TablesModelMetadata.newBuilder() to construct.
private TablesModelMetadata(com.google.protobuf.GeneratedMessageV3.Builder> builder) {
super(builder);
}
private TablesModelMetadata() {
inputFeatureColumnSpecs_ = java.util.Collections.emptyList();
optimizationObjective_ = "";
tablesModelColumnInfo_ = java.util.Collections.emptyList();
}
@java.lang.Override
@SuppressWarnings({"unused"})
protected java.lang.Object newInstance(UnusedPrivateParameter unused) {
return new TablesModelMetadata();
}
@java.lang.Override
public final com.google.protobuf.UnknownFieldSet getUnknownFields() {
return this.unknownFields;
}
public static final com.google.protobuf.Descriptors.Descriptor getDescriptor() {
return com.google.cloud.automl.v1beta1.Tables
.internal_static_google_cloud_automl_v1beta1_TablesModelMetadata_descriptor;
}
@java.lang.Override
protected com.google.protobuf.GeneratedMessageV3.FieldAccessorTable
internalGetFieldAccessorTable() {
return com.google.cloud.automl.v1beta1.Tables
.internal_static_google_cloud_automl_v1beta1_TablesModelMetadata_fieldAccessorTable
.ensureFieldAccessorsInitialized(
com.google.cloud.automl.v1beta1.TablesModelMetadata.class,
com.google.cloud.automl.v1beta1.TablesModelMetadata.Builder.class);
}
private int additionalOptimizationObjectiveConfigCase_ = 0;
private java.lang.Object additionalOptimizationObjectiveConfig_;
public enum AdditionalOptimizationObjectiveConfigCase
implements
com.google.protobuf.Internal.EnumLite,
com.google.protobuf.AbstractMessage.InternalOneOfEnum {
OPTIMIZATION_OBJECTIVE_RECALL_VALUE(17),
OPTIMIZATION_OBJECTIVE_PRECISION_VALUE(18),
ADDITIONALOPTIMIZATIONOBJECTIVECONFIG_NOT_SET(0);
private final int value;
private AdditionalOptimizationObjectiveConfigCase(int value) {
this.value = value;
}
/**
* @param value The number of the enum to look for.
* @return The enum associated with the given number.
* @deprecated Use {@link #forNumber(int)} instead.
*/
@java.lang.Deprecated
public static AdditionalOptimizationObjectiveConfigCase valueOf(int value) {
return forNumber(value);
}
public static AdditionalOptimizationObjectiveConfigCase forNumber(int value) {
switch (value) {
case 17:
return OPTIMIZATION_OBJECTIVE_RECALL_VALUE;
case 18:
return OPTIMIZATION_OBJECTIVE_PRECISION_VALUE;
case 0:
return ADDITIONALOPTIMIZATIONOBJECTIVECONFIG_NOT_SET;
default:
return null;
}
}
public int getNumber() {
return this.value;
}
};
public AdditionalOptimizationObjectiveConfigCase getAdditionalOptimizationObjectiveConfigCase() {
return AdditionalOptimizationObjectiveConfigCase.forNumber(
additionalOptimizationObjectiveConfigCase_);
}
public static final int OPTIMIZATION_OBJECTIVE_RECALL_VALUE_FIELD_NUMBER = 17;
/**
*
*
*
* Required when optimization_objective is "MAXIMIZE_PRECISION_AT_RECALL".
* Must be between 0 and 1, inclusive.
*
*
* float optimization_objective_recall_value = 17;
*
* @return Whether the optimizationObjectiveRecallValue field is set.
*/
@java.lang.Override
public boolean hasOptimizationObjectiveRecallValue() {
return additionalOptimizationObjectiveConfigCase_ == 17;
}
/**
*
*
*
* Required when optimization_objective is "MAXIMIZE_PRECISION_AT_RECALL".
* Must be between 0 and 1, inclusive.
*
*
* float optimization_objective_recall_value = 17;
*
* @return The optimizationObjectiveRecallValue.
*/
@java.lang.Override
public float getOptimizationObjectiveRecallValue() {
if (additionalOptimizationObjectiveConfigCase_ == 17) {
return (java.lang.Float) additionalOptimizationObjectiveConfig_;
}
return 0F;
}
public static final int OPTIMIZATION_OBJECTIVE_PRECISION_VALUE_FIELD_NUMBER = 18;
/**
*
*
*
* Required when optimization_objective is "MAXIMIZE_RECALL_AT_PRECISION".
* Must be between 0 and 1, inclusive.
*
*
* float optimization_objective_precision_value = 18;
*
* @return Whether the optimizationObjectivePrecisionValue field is set.
*/
@java.lang.Override
public boolean hasOptimizationObjectivePrecisionValue() {
return additionalOptimizationObjectiveConfigCase_ == 18;
}
/**
*
*
*
* Required when optimization_objective is "MAXIMIZE_RECALL_AT_PRECISION".
* Must be between 0 and 1, inclusive.
*
*
* float optimization_objective_precision_value = 18;
*
* @return The optimizationObjectivePrecisionValue.
*/
@java.lang.Override
public float getOptimizationObjectivePrecisionValue() {
if (additionalOptimizationObjectiveConfigCase_ == 18) {
return (java.lang.Float) additionalOptimizationObjectiveConfig_;
}
return 0F;
}
public static final int TARGET_COLUMN_SPEC_FIELD_NUMBER = 2;
private com.google.cloud.automl.v1beta1.ColumnSpec targetColumnSpec_;
/**
*
*
*
* Column spec of the dataset's primary table's column the model is
* predicting. Snapshotted when model creation started.
* Only 3 fields are used:
* name - May be set on CreateModel, if it's not then the ColumnSpec
* corresponding to the current target_column_spec_id of the dataset
* the model is trained from is used.
* If neither is set, CreateModel will error.
* display_name - Output only.
* data_type - Output only.
*
*
* .google.cloud.automl.v1beta1.ColumnSpec target_column_spec = 2;
*
* @return Whether the targetColumnSpec field is set.
*/
@java.lang.Override
public boolean hasTargetColumnSpec() {
return targetColumnSpec_ != null;
}
/**
*
*
*
* Column spec of the dataset's primary table's column the model is
* predicting. Snapshotted when model creation started.
* Only 3 fields are used:
* name - May be set on CreateModel, if it's not then the ColumnSpec
* corresponding to the current target_column_spec_id of the dataset
* the model is trained from is used.
* If neither is set, CreateModel will error.
* display_name - Output only.
* data_type - Output only.
*
*
* .google.cloud.automl.v1beta1.ColumnSpec target_column_spec = 2;
*
* @return The targetColumnSpec.
*/
@java.lang.Override
public com.google.cloud.automl.v1beta1.ColumnSpec getTargetColumnSpec() {
return targetColumnSpec_ == null
? com.google.cloud.automl.v1beta1.ColumnSpec.getDefaultInstance()
: targetColumnSpec_;
}
/**
*
*
*
* Column spec of the dataset's primary table's column the model is
* predicting. Snapshotted when model creation started.
* Only 3 fields are used:
* name - May be set on CreateModel, if it's not then the ColumnSpec
* corresponding to the current target_column_spec_id of the dataset
* the model is trained from is used.
* If neither is set, CreateModel will error.
* display_name - Output only.
* data_type - Output only.
*
*
* .google.cloud.automl.v1beta1.ColumnSpec target_column_spec = 2;
*/
@java.lang.Override
public com.google.cloud.automl.v1beta1.ColumnSpecOrBuilder getTargetColumnSpecOrBuilder() {
return getTargetColumnSpec();
}
public static final int INPUT_FEATURE_COLUMN_SPECS_FIELD_NUMBER = 3;
private java.util.List inputFeatureColumnSpecs_;
/**
*
*
*
* Column specs of the dataset's primary table's columns, on which
* the model is trained and which are used as the input for predictions.
* The
* [target_column][google.cloud.automl.v1beta1.TablesModelMetadata.target_column_spec]
* as well as, according to dataset's state upon model creation,
* [weight_column][google.cloud.automl.v1beta1.TablesDatasetMetadata.weight_column_spec_id],
* and
* [ml_use_column][google.cloud.automl.v1beta1.TablesDatasetMetadata.ml_use_column_spec_id]
* must never be included here.
* Only 3 fields are used:
* * name - May be set on CreateModel, if set only the columns specified are
* used, otherwise all primary table's columns (except the ones listed
* above) are used for the training and prediction input.
* * display_name - Output only.
* * data_type - Output only.
*
*
* repeated .google.cloud.automl.v1beta1.ColumnSpec input_feature_column_specs = 3;
*/
@java.lang.Override
public java.util.List
getInputFeatureColumnSpecsList() {
return inputFeatureColumnSpecs_;
}
/**
*
*
*
* Column specs of the dataset's primary table's columns, on which
* the model is trained and which are used as the input for predictions.
* The
* [target_column][google.cloud.automl.v1beta1.TablesModelMetadata.target_column_spec]
* as well as, according to dataset's state upon model creation,
* [weight_column][google.cloud.automl.v1beta1.TablesDatasetMetadata.weight_column_spec_id],
* and
* [ml_use_column][google.cloud.automl.v1beta1.TablesDatasetMetadata.ml_use_column_spec_id]
* must never be included here.
* Only 3 fields are used:
* * name - May be set on CreateModel, if set only the columns specified are
* used, otherwise all primary table's columns (except the ones listed
* above) are used for the training and prediction input.
* * display_name - Output only.
* * data_type - Output only.
*
*
* repeated .google.cloud.automl.v1beta1.ColumnSpec input_feature_column_specs = 3;
*/
@java.lang.Override
public java.util.List extends com.google.cloud.automl.v1beta1.ColumnSpecOrBuilder>
getInputFeatureColumnSpecsOrBuilderList() {
return inputFeatureColumnSpecs_;
}
/**
*
*
*
* Column specs of the dataset's primary table's columns, on which
* the model is trained and which are used as the input for predictions.
* The
* [target_column][google.cloud.automl.v1beta1.TablesModelMetadata.target_column_spec]
* as well as, according to dataset's state upon model creation,
* [weight_column][google.cloud.automl.v1beta1.TablesDatasetMetadata.weight_column_spec_id],
* and
* [ml_use_column][google.cloud.automl.v1beta1.TablesDatasetMetadata.ml_use_column_spec_id]
* must never be included here.
* Only 3 fields are used:
* * name - May be set on CreateModel, if set only the columns specified are
* used, otherwise all primary table's columns (except the ones listed
* above) are used for the training and prediction input.
* * display_name - Output only.
* * data_type - Output only.
*
*
* repeated .google.cloud.automl.v1beta1.ColumnSpec input_feature_column_specs = 3;
*/
@java.lang.Override
public int getInputFeatureColumnSpecsCount() {
return inputFeatureColumnSpecs_.size();
}
/**
*
*
*
* Column specs of the dataset's primary table's columns, on which
* the model is trained and which are used as the input for predictions.
* The
* [target_column][google.cloud.automl.v1beta1.TablesModelMetadata.target_column_spec]
* as well as, according to dataset's state upon model creation,
* [weight_column][google.cloud.automl.v1beta1.TablesDatasetMetadata.weight_column_spec_id],
* and
* [ml_use_column][google.cloud.automl.v1beta1.TablesDatasetMetadata.ml_use_column_spec_id]
* must never be included here.
* Only 3 fields are used:
* * name - May be set on CreateModel, if set only the columns specified are
* used, otherwise all primary table's columns (except the ones listed
* above) are used for the training and prediction input.
* * display_name - Output only.
* * data_type - Output only.
*
*
* repeated .google.cloud.automl.v1beta1.ColumnSpec input_feature_column_specs = 3;
*/
@java.lang.Override
public com.google.cloud.automl.v1beta1.ColumnSpec getInputFeatureColumnSpecs(int index) {
return inputFeatureColumnSpecs_.get(index);
}
/**
*
*
*
* Column specs of the dataset's primary table's columns, on which
* the model is trained and which are used as the input for predictions.
* The
* [target_column][google.cloud.automl.v1beta1.TablesModelMetadata.target_column_spec]
* as well as, according to dataset's state upon model creation,
* [weight_column][google.cloud.automl.v1beta1.TablesDatasetMetadata.weight_column_spec_id],
* and
* [ml_use_column][google.cloud.automl.v1beta1.TablesDatasetMetadata.ml_use_column_spec_id]
* must never be included here.
* Only 3 fields are used:
* * name - May be set on CreateModel, if set only the columns specified are
* used, otherwise all primary table's columns (except the ones listed
* above) are used for the training and prediction input.
* * display_name - Output only.
* * data_type - Output only.
*
*
* repeated .google.cloud.automl.v1beta1.ColumnSpec input_feature_column_specs = 3;
*/
@java.lang.Override
public com.google.cloud.automl.v1beta1.ColumnSpecOrBuilder getInputFeatureColumnSpecsOrBuilder(
int index) {
return inputFeatureColumnSpecs_.get(index);
}
public static final int OPTIMIZATION_OBJECTIVE_FIELD_NUMBER = 4;
private volatile java.lang.Object optimizationObjective_;
/**
*
*
*
* Objective function the model is optimizing towards. The training process
* creates a model that maximizes/minimizes the value of the objective
* function over the validation set.
* The supported optimization objectives depend on the prediction type.
* If the field is not set, a default objective function is used.
* CLASSIFICATION_BINARY:
* "MAXIMIZE_AU_ROC" (default) - Maximize the area under the receiver
* operating characteristic (ROC) curve.
* "MINIMIZE_LOG_LOSS" - Minimize log loss.
* "MAXIMIZE_AU_PRC" - Maximize the area under the precision-recall curve.
* "MAXIMIZE_PRECISION_AT_RECALL" - Maximize precision for a specified
* recall value.
* "MAXIMIZE_RECALL_AT_PRECISION" - Maximize recall for a specified
* precision value.
* CLASSIFICATION_MULTI_CLASS :
* "MINIMIZE_LOG_LOSS" (default) - Minimize log loss.
* REGRESSION:
* "MINIMIZE_RMSE" (default) - Minimize root-mean-squared error (RMSE).
* "MINIMIZE_MAE" - Minimize mean-absolute error (MAE).
* "MINIMIZE_RMSLE" - Minimize root-mean-squared log error (RMSLE).
*
*
* string optimization_objective = 4;
*
* @return The optimizationObjective.
*/
@java.lang.Override
public java.lang.String getOptimizationObjective() {
java.lang.Object ref = optimizationObjective_;
if (ref instanceof java.lang.String) {
return (java.lang.String) ref;
} else {
com.google.protobuf.ByteString bs = (com.google.protobuf.ByteString) ref;
java.lang.String s = bs.toStringUtf8();
optimizationObjective_ = s;
return s;
}
}
/**
*
*
*
* Objective function the model is optimizing towards. The training process
* creates a model that maximizes/minimizes the value of the objective
* function over the validation set.
* The supported optimization objectives depend on the prediction type.
* If the field is not set, a default objective function is used.
* CLASSIFICATION_BINARY:
* "MAXIMIZE_AU_ROC" (default) - Maximize the area under the receiver
* operating characteristic (ROC) curve.
* "MINIMIZE_LOG_LOSS" - Minimize log loss.
* "MAXIMIZE_AU_PRC" - Maximize the area under the precision-recall curve.
* "MAXIMIZE_PRECISION_AT_RECALL" - Maximize precision for a specified
* recall value.
* "MAXIMIZE_RECALL_AT_PRECISION" - Maximize recall for a specified
* precision value.
* CLASSIFICATION_MULTI_CLASS :
* "MINIMIZE_LOG_LOSS" (default) - Minimize log loss.
* REGRESSION:
* "MINIMIZE_RMSE" (default) - Minimize root-mean-squared error (RMSE).
* "MINIMIZE_MAE" - Minimize mean-absolute error (MAE).
* "MINIMIZE_RMSLE" - Minimize root-mean-squared log error (RMSLE).
*
*
* string optimization_objective = 4;
*
* @return The bytes for optimizationObjective.
*/
@java.lang.Override
public com.google.protobuf.ByteString getOptimizationObjectiveBytes() {
java.lang.Object ref = optimizationObjective_;
if (ref instanceof java.lang.String) {
com.google.protobuf.ByteString b =
com.google.protobuf.ByteString.copyFromUtf8((java.lang.String) ref);
optimizationObjective_ = b;
return b;
} else {
return (com.google.protobuf.ByteString) ref;
}
}
public static final int TABLES_MODEL_COLUMN_INFO_FIELD_NUMBER = 5;
private java.util.List
tablesModelColumnInfo_;
/**
*
*
*
* Output only. Auxiliary information for each of the
* input_feature_column_specs with respect to this particular model.
*
*
* repeated .google.cloud.automl.v1beta1.TablesModelColumnInfo tables_model_column_info = 5;
*
*/
@java.lang.Override
public java.util.List
getTablesModelColumnInfoList() {
return tablesModelColumnInfo_;
}
/**
*
*
*
* Output only. Auxiliary information for each of the
* input_feature_column_specs with respect to this particular model.
*
*
* repeated .google.cloud.automl.v1beta1.TablesModelColumnInfo tables_model_column_info = 5;
*
*/
@java.lang.Override
public java.util.List extends com.google.cloud.automl.v1beta1.TablesModelColumnInfoOrBuilder>
getTablesModelColumnInfoOrBuilderList() {
return tablesModelColumnInfo_;
}
/**
*
*
*
* Output only. Auxiliary information for each of the
* input_feature_column_specs with respect to this particular model.
*
*
* repeated .google.cloud.automl.v1beta1.TablesModelColumnInfo tables_model_column_info = 5;
*
*/
@java.lang.Override
public int getTablesModelColumnInfoCount() {
return tablesModelColumnInfo_.size();
}
/**
*
*
*
* Output only. Auxiliary information for each of the
* input_feature_column_specs with respect to this particular model.
*
*
* repeated .google.cloud.automl.v1beta1.TablesModelColumnInfo tables_model_column_info = 5;
*
*/
@java.lang.Override
public com.google.cloud.automl.v1beta1.TablesModelColumnInfo getTablesModelColumnInfo(int index) {
return tablesModelColumnInfo_.get(index);
}
/**
*
*
*
* Output only. Auxiliary information for each of the
* input_feature_column_specs with respect to this particular model.
*
*
* repeated .google.cloud.automl.v1beta1.TablesModelColumnInfo tables_model_column_info = 5;
*
*/
@java.lang.Override
public com.google.cloud.automl.v1beta1.TablesModelColumnInfoOrBuilder
getTablesModelColumnInfoOrBuilder(int index) {
return tablesModelColumnInfo_.get(index);
}
public static final int TRAIN_BUDGET_MILLI_NODE_HOURS_FIELD_NUMBER = 6;
private long trainBudgetMilliNodeHours_;
/**
*
*
*
* Required. The train budget of creating this model, expressed in milli node
* hours i.e. 1,000 value in this field means 1 node hour.
* The training cost of the model will not exceed this budget. The final cost
* will be attempted to be close to the budget, though may end up being (even)
* noticeably smaller - at the backend's discretion. This especially may
* happen when further model training ceases to provide any improvements.
* If the budget is set to a value known to be insufficient to train a
* model for the given dataset, the training won't be attempted and
* will error.
* The train budget must be between 1,000 and 72,000 milli node hours,
* inclusive.
*
*
* int64 train_budget_milli_node_hours = 6;
*
* @return The trainBudgetMilliNodeHours.
*/
@java.lang.Override
public long getTrainBudgetMilliNodeHours() {
return trainBudgetMilliNodeHours_;
}
public static final int TRAIN_COST_MILLI_NODE_HOURS_FIELD_NUMBER = 7;
private long trainCostMilliNodeHours_;
/**
*
*
*
* Output only. The actual training cost of the model, expressed in milli
* node hours, i.e. 1,000 value in this field means 1 node hour. Guaranteed
* to not exceed the train budget.
*
*
* int64 train_cost_milli_node_hours = 7;
*
* @return The trainCostMilliNodeHours.
*/
@java.lang.Override
public long getTrainCostMilliNodeHours() {
return trainCostMilliNodeHours_;
}
public static final int DISABLE_EARLY_STOPPING_FIELD_NUMBER = 12;
private boolean disableEarlyStopping_;
/**
*
*
*
* Use the entire training budget. This disables the early stopping feature.
* By default, the early stopping feature is enabled, which means that AutoML
* Tables might stop training before the entire training budget has been used.
*
*
* bool disable_early_stopping = 12;
*
* @return The disableEarlyStopping.
*/
@java.lang.Override
public boolean getDisableEarlyStopping() {
return disableEarlyStopping_;
}
private byte memoizedIsInitialized = -1;
@java.lang.Override
public final boolean isInitialized() {
byte isInitialized = memoizedIsInitialized;
if (isInitialized == 1) return true;
if (isInitialized == 0) return false;
memoizedIsInitialized = 1;
return true;
}
@java.lang.Override
public void writeTo(com.google.protobuf.CodedOutputStream output) throws java.io.IOException {
if (targetColumnSpec_ != null) {
output.writeMessage(2, getTargetColumnSpec());
}
for (int i = 0; i < inputFeatureColumnSpecs_.size(); i++) {
output.writeMessage(3, inputFeatureColumnSpecs_.get(i));
}
if (!com.google.protobuf.GeneratedMessageV3.isStringEmpty(optimizationObjective_)) {
com.google.protobuf.GeneratedMessageV3.writeString(output, 4, optimizationObjective_);
}
for (int i = 0; i < tablesModelColumnInfo_.size(); i++) {
output.writeMessage(5, tablesModelColumnInfo_.get(i));
}
if (trainBudgetMilliNodeHours_ != 0L) {
output.writeInt64(6, trainBudgetMilliNodeHours_);
}
if (trainCostMilliNodeHours_ != 0L) {
output.writeInt64(7, trainCostMilliNodeHours_);
}
if (disableEarlyStopping_ != false) {
output.writeBool(12, disableEarlyStopping_);
}
if (additionalOptimizationObjectiveConfigCase_ == 17) {
output.writeFloat(17, (float) ((java.lang.Float) additionalOptimizationObjectiveConfig_));
}
if (additionalOptimizationObjectiveConfigCase_ == 18) {
output.writeFloat(18, (float) ((java.lang.Float) additionalOptimizationObjectiveConfig_));
}
getUnknownFields().writeTo(output);
}
@java.lang.Override
public int getSerializedSize() {
int size = memoizedSize;
if (size != -1) return size;
size = 0;
if (targetColumnSpec_ != null) {
size += com.google.protobuf.CodedOutputStream.computeMessageSize(2, getTargetColumnSpec());
}
for (int i = 0; i < inputFeatureColumnSpecs_.size(); i++) {
size +=
com.google.protobuf.CodedOutputStream.computeMessageSize(
3, inputFeatureColumnSpecs_.get(i));
}
if (!com.google.protobuf.GeneratedMessageV3.isStringEmpty(optimizationObjective_)) {
size += com.google.protobuf.GeneratedMessageV3.computeStringSize(4, optimizationObjective_);
}
for (int i = 0; i < tablesModelColumnInfo_.size(); i++) {
size +=
com.google.protobuf.CodedOutputStream.computeMessageSize(
5, tablesModelColumnInfo_.get(i));
}
if (trainBudgetMilliNodeHours_ != 0L) {
size += com.google.protobuf.CodedOutputStream.computeInt64Size(6, trainBudgetMilliNodeHours_);
}
if (trainCostMilliNodeHours_ != 0L) {
size += com.google.protobuf.CodedOutputStream.computeInt64Size(7, trainCostMilliNodeHours_);
}
if (disableEarlyStopping_ != false) {
size += com.google.protobuf.CodedOutputStream.computeBoolSize(12, disableEarlyStopping_);
}
if (additionalOptimizationObjectiveConfigCase_ == 17) {
size +=
com.google.protobuf.CodedOutputStream.computeFloatSize(
17, (float) ((java.lang.Float) additionalOptimizationObjectiveConfig_));
}
if (additionalOptimizationObjectiveConfigCase_ == 18) {
size +=
com.google.protobuf.CodedOutputStream.computeFloatSize(
18, (float) ((java.lang.Float) additionalOptimizationObjectiveConfig_));
}
size += getUnknownFields().getSerializedSize();
memoizedSize = size;
return size;
}
@java.lang.Override
public boolean equals(final java.lang.Object obj) {
if (obj == this) {
return true;
}
if (!(obj instanceof com.google.cloud.automl.v1beta1.TablesModelMetadata)) {
return super.equals(obj);
}
com.google.cloud.automl.v1beta1.TablesModelMetadata other =
(com.google.cloud.automl.v1beta1.TablesModelMetadata) obj;
if (hasTargetColumnSpec() != other.hasTargetColumnSpec()) return false;
if (hasTargetColumnSpec()) {
if (!getTargetColumnSpec().equals(other.getTargetColumnSpec())) return false;
}
if (!getInputFeatureColumnSpecsList().equals(other.getInputFeatureColumnSpecsList()))
return false;
if (!getOptimizationObjective().equals(other.getOptimizationObjective())) return false;
if (!getTablesModelColumnInfoList().equals(other.getTablesModelColumnInfoList())) return false;
if (getTrainBudgetMilliNodeHours() != other.getTrainBudgetMilliNodeHours()) return false;
if (getTrainCostMilliNodeHours() != other.getTrainCostMilliNodeHours()) return false;
if (getDisableEarlyStopping() != other.getDisableEarlyStopping()) return false;
if (!getAdditionalOptimizationObjectiveConfigCase()
.equals(other.getAdditionalOptimizationObjectiveConfigCase())) return false;
switch (additionalOptimizationObjectiveConfigCase_) {
case 17:
if (java.lang.Float.floatToIntBits(getOptimizationObjectiveRecallValue())
!= java.lang.Float.floatToIntBits(other.getOptimizationObjectiveRecallValue()))
return false;
break;
case 18:
if (java.lang.Float.floatToIntBits(getOptimizationObjectivePrecisionValue())
!= java.lang.Float.floatToIntBits(other.getOptimizationObjectivePrecisionValue()))
return false;
break;
case 0:
default:
}
if (!getUnknownFields().equals(other.getUnknownFields())) return false;
return true;
}
@java.lang.Override
public int hashCode() {
if (memoizedHashCode != 0) {
return memoizedHashCode;
}
int hash = 41;
hash = (19 * hash) + getDescriptor().hashCode();
if (hasTargetColumnSpec()) {
hash = (37 * hash) + TARGET_COLUMN_SPEC_FIELD_NUMBER;
hash = (53 * hash) + getTargetColumnSpec().hashCode();
}
if (getInputFeatureColumnSpecsCount() > 0) {
hash = (37 * hash) + INPUT_FEATURE_COLUMN_SPECS_FIELD_NUMBER;
hash = (53 * hash) + getInputFeatureColumnSpecsList().hashCode();
}
hash = (37 * hash) + OPTIMIZATION_OBJECTIVE_FIELD_NUMBER;
hash = (53 * hash) + getOptimizationObjective().hashCode();
if (getTablesModelColumnInfoCount() > 0) {
hash = (37 * hash) + TABLES_MODEL_COLUMN_INFO_FIELD_NUMBER;
hash = (53 * hash) + getTablesModelColumnInfoList().hashCode();
}
hash = (37 * hash) + TRAIN_BUDGET_MILLI_NODE_HOURS_FIELD_NUMBER;
hash = (53 * hash) + com.google.protobuf.Internal.hashLong(getTrainBudgetMilliNodeHours());
hash = (37 * hash) + TRAIN_COST_MILLI_NODE_HOURS_FIELD_NUMBER;
hash = (53 * hash) + com.google.protobuf.Internal.hashLong(getTrainCostMilliNodeHours());
hash = (37 * hash) + DISABLE_EARLY_STOPPING_FIELD_NUMBER;
hash = (53 * hash) + com.google.protobuf.Internal.hashBoolean(getDisableEarlyStopping());
switch (additionalOptimizationObjectiveConfigCase_) {
case 17:
hash = (37 * hash) + OPTIMIZATION_OBJECTIVE_RECALL_VALUE_FIELD_NUMBER;
hash = (53 * hash) + java.lang.Float.floatToIntBits(getOptimizationObjectiveRecallValue());
break;
case 18:
hash = (37 * hash) + OPTIMIZATION_OBJECTIVE_PRECISION_VALUE_FIELD_NUMBER;
hash =
(53 * hash) + java.lang.Float.floatToIntBits(getOptimizationObjectivePrecisionValue());
break;
case 0:
default:
}
hash = (29 * hash) + getUnknownFields().hashCode();
memoizedHashCode = hash;
return hash;
}
public static com.google.cloud.automl.v1beta1.TablesModelMetadata parseFrom(
java.nio.ByteBuffer data) throws com.google.protobuf.InvalidProtocolBufferException {
return PARSER.parseFrom(data);
}
public static com.google.cloud.automl.v1beta1.TablesModelMetadata parseFrom(
java.nio.ByteBuffer data, com.google.protobuf.ExtensionRegistryLite extensionRegistry)
throws com.google.protobuf.InvalidProtocolBufferException {
return PARSER.parseFrom(data, extensionRegistry);
}
public static com.google.cloud.automl.v1beta1.TablesModelMetadata parseFrom(
com.google.protobuf.ByteString data)
throws com.google.protobuf.InvalidProtocolBufferException {
return PARSER.parseFrom(data);
}
public static com.google.cloud.automl.v1beta1.TablesModelMetadata parseFrom(
com.google.protobuf.ByteString data,
com.google.protobuf.ExtensionRegistryLite extensionRegistry)
throws com.google.protobuf.InvalidProtocolBufferException {
return PARSER.parseFrom(data, extensionRegistry);
}
public static com.google.cloud.automl.v1beta1.TablesModelMetadata parseFrom(byte[] data)
throws com.google.protobuf.InvalidProtocolBufferException {
return PARSER.parseFrom(data);
}
public static com.google.cloud.automl.v1beta1.TablesModelMetadata parseFrom(
byte[] data, com.google.protobuf.ExtensionRegistryLite extensionRegistry)
throws com.google.protobuf.InvalidProtocolBufferException {
return PARSER.parseFrom(data, extensionRegistry);
}
public static com.google.cloud.automl.v1beta1.TablesModelMetadata parseFrom(
java.io.InputStream input) throws java.io.IOException {
return com.google.protobuf.GeneratedMessageV3.parseWithIOException(PARSER, input);
}
public static com.google.cloud.automl.v1beta1.TablesModelMetadata parseFrom(
java.io.InputStream input, com.google.protobuf.ExtensionRegistryLite extensionRegistry)
throws java.io.IOException {
return com.google.protobuf.GeneratedMessageV3.parseWithIOException(
PARSER, input, extensionRegistry);
}
public static com.google.cloud.automl.v1beta1.TablesModelMetadata parseDelimitedFrom(
java.io.InputStream input) throws java.io.IOException {
return com.google.protobuf.GeneratedMessageV3.parseDelimitedWithIOException(PARSER, input);
}
public static com.google.cloud.automl.v1beta1.TablesModelMetadata parseDelimitedFrom(
java.io.InputStream input, com.google.protobuf.ExtensionRegistryLite extensionRegistry)
throws java.io.IOException {
return com.google.protobuf.GeneratedMessageV3.parseDelimitedWithIOException(
PARSER, input, extensionRegistry);
}
public static com.google.cloud.automl.v1beta1.TablesModelMetadata parseFrom(
com.google.protobuf.CodedInputStream input) throws java.io.IOException {
return com.google.protobuf.GeneratedMessageV3.parseWithIOException(PARSER, input);
}
public static com.google.cloud.automl.v1beta1.TablesModelMetadata parseFrom(
com.google.protobuf.CodedInputStream input,
com.google.protobuf.ExtensionRegistryLite extensionRegistry)
throws java.io.IOException {
return com.google.protobuf.GeneratedMessageV3.parseWithIOException(
PARSER, input, extensionRegistry);
}
@java.lang.Override
public Builder newBuilderForType() {
return newBuilder();
}
public static Builder newBuilder() {
return DEFAULT_INSTANCE.toBuilder();
}
public static Builder newBuilder(com.google.cloud.automl.v1beta1.TablesModelMetadata prototype) {
return DEFAULT_INSTANCE.toBuilder().mergeFrom(prototype);
}
@java.lang.Override
public Builder toBuilder() {
return this == DEFAULT_INSTANCE ? new Builder() : new Builder().mergeFrom(this);
}
@java.lang.Override
protected Builder newBuilderForType(com.google.protobuf.GeneratedMessageV3.BuilderParent parent) {
Builder builder = new Builder(parent);
return builder;
}
/**
*
*
*
* Model metadata specific to AutoML Tables.
*
*
* Protobuf type {@code google.cloud.automl.v1beta1.TablesModelMetadata}
*/
public static final class Builder extends com.google.protobuf.GeneratedMessageV3.Builder
implements
// @@protoc_insertion_point(builder_implements:google.cloud.automl.v1beta1.TablesModelMetadata)
com.google.cloud.automl.v1beta1.TablesModelMetadataOrBuilder {
public static final com.google.protobuf.Descriptors.Descriptor getDescriptor() {
return com.google.cloud.automl.v1beta1.Tables
.internal_static_google_cloud_automl_v1beta1_TablesModelMetadata_descriptor;
}
@java.lang.Override
protected com.google.protobuf.GeneratedMessageV3.FieldAccessorTable
internalGetFieldAccessorTable() {
return com.google.cloud.automl.v1beta1.Tables
.internal_static_google_cloud_automl_v1beta1_TablesModelMetadata_fieldAccessorTable
.ensureFieldAccessorsInitialized(
com.google.cloud.automl.v1beta1.TablesModelMetadata.class,
com.google.cloud.automl.v1beta1.TablesModelMetadata.Builder.class);
}
// Construct using com.google.cloud.automl.v1beta1.TablesModelMetadata.newBuilder()
private Builder() {}
private Builder(com.google.protobuf.GeneratedMessageV3.BuilderParent parent) {
super(parent);
}
@java.lang.Override
public Builder clear() {
super.clear();
if (targetColumnSpecBuilder_ == null) {
targetColumnSpec_ = null;
} else {
targetColumnSpec_ = null;
targetColumnSpecBuilder_ = null;
}
if (inputFeatureColumnSpecsBuilder_ == null) {
inputFeatureColumnSpecs_ = java.util.Collections.emptyList();
} else {
inputFeatureColumnSpecs_ = null;
inputFeatureColumnSpecsBuilder_.clear();
}
bitField0_ = (bitField0_ & ~0x00000001);
optimizationObjective_ = "";
if (tablesModelColumnInfoBuilder_ == null) {
tablesModelColumnInfo_ = java.util.Collections.emptyList();
} else {
tablesModelColumnInfo_ = null;
tablesModelColumnInfoBuilder_.clear();
}
bitField0_ = (bitField0_ & ~0x00000002);
trainBudgetMilliNodeHours_ = 0L;
trainCostMilliNodeHours_ = 0L;
disableEarlyStopping_ = false;
additionalOptimizationObjectiveConfigCase_ = 0;
additionalOptimizationObjectiveConfig_ = null;
return this;
}
@java.lang.Override
public com.google.protobuf.Descriptors.Descriptor getDescriptorForType() {
return com.google.cloud.automl.v1beta1.Tables
.internal_static_google_cloud_automl_v1beta1_TablesModelMetadata_descriptor;
}
@java.lang.Override
public com.google.cloud.automl.v1beta1.TablesModelMetadata getDefaultInstanceForType() {
return com.google.cloud.automl.v1beta1.TablesModelMetadata.getDefaultInstance();
}
@java.lang.Override
public com.google.cloud.automl.v1beta1.TablesModelMetadata build() {
com.google.cloud.automl.v1beta1.TablesModelMetadata result = buildPartial();
if (!result.isInitialized()) {
throw newUninitializedMessageException(result);
}
return result;
}
@java.lang.Override
public com.google.cloud.automl.v1beta1.TablesModelMetadata buildPartial() {
com.google.cloud.automl.v1beta1.TablesModelMetadata result =
new com.google.cloud.automl.v1beta1.TablesModelMetadata(this);
int from_bitField0_ = bitField0_;
if (additionalOptimizationObjectiveConfigCase_ == 17) {
result.additionalOptimizationObjectiveConfig_ = additionalOptimizationObjectiveConfig_;
}
if (additionalOptimizationObjectiveConfigCase_ == 18) {
result.additionalOptimizationObjectiveConfig_ = additionalOptimizationObjectiveConfig_;
}
if (targetColumnSpecBuilder_ == null) {
result.targetColumnSpec_ = targetColumnSpec_;
} else {
result.targetColumnSpec_ = targetColumnSpecBuilder_.build();
}
if (inputFeatureColumnSpecsBuilder_ == null) {
if (((bitField0_ & 0x00000001) != 0)) {
inputFeatureColumnSpecs_ =
java.util.Collections.unmodifiableList(inputFeatureColumnSpecs_);
bitField0_ = (bitField0_ & ~0x00000001);
}
result.inputFeatureColumnSpecs_ = inputFeatureColumnSpecs_;
} else {
result.inputFeatureColumnSpecs_ = inputFeatureColumnSpecsBuilder_.build();
}
result.optimizationObjective_ = optimizationObjective_;
if (tablesModelColumnInfoBuilder_ == null) {
if (((bitField0_ & 0x00000002) != 0)) {
tablesModelColumnInfo_ = java.util.Collections.unmodifiableList(tablesModelColumnInfo_);
bitField0_ = (bitField0_ & ~0x00000002);
}
result.tablesModelColumnInfo_ = tablesModelColumnInfo_;
} else {
result.tablesModelColumnInfo_ = tablesModelColumnInfoBuilder_.build();
}
result.trainBudgetMilliNodeHours_ = trainBudgetMilliNodeHours_;
result.trainCostMilliNodeHours_ = trainCostMilliNodeHours_;
result.disableEarlyStopping_ = disableEarlyStopping_;
result.additionalOptimizationObjectiveConfigCase_ =
additionalOptimizationObjectiveConfigCase_;
onBuilt();
return result;
}
@java.lang.Override
public Builder clone() {
return super.clone();
}
@java.lang.Override
public Builder setField(
com.google.protobuf.Descriptors.FieldDescriptor field, java.lang.Object value) {
return super.setField(field, value);
}
@java.lang.Override
public Builder clearField(com.google.protobuf.Descriptors.FieldDescriptor field) {
return super.clearField(field);
}
@java.lang.Override
public Builder clearOneof(com.google.protobuf.Descriptors.OneofDescriptor oneof) {
return super.clearOneof(oneof);
}
@java.lang.Override
public Builder setRepeatedField(
com.google.protobuf.Descriptors.FieldDescriptor field, int index, java.lang.Object value) {
return super.setRepeatedField(field, index, value);
}
@java.lang.Override
public Builder addRepeatedField(
com.google.protobuf.Descriptors.FieldDescriptor field, java.lang.Object value) {
return super.addRepeatedField(field, value);
}
@java.lang.Override
public Builder mergeFrom(com.google.protobuf.Message other) {
if (other instanceof com.google.cloud.automl.v1beta1.TablesModelMetadata) {
return mergeFrom((com.google.cloud.automl.v1beta1.TablesModelMetadata) other);
} else {
super.mergeFrom(other);
return this;
}
}
public Builder mergeFrom(com.google.cloud.automl.v1beta1.TablesModelMetadata other) {
if (other == com.google.cloud.automl.v1beta1.TablesModelMetadata.getDefaultInstance())
return this;
if (other.hasTargetColumnSpec()) {
mergeTargetColumnSpec(other.getTargetColumnSpec());
}
if (inputFeatureColumnSpecsBuilder_ == null) {
if (!other.inputFeatureColumnSpecs_.isEmpty()) {
if (inputFeatureColumnSpecs_.isEmpty()) {
inputFeatureColumnSpecs_ = other.inputFeatureColumnSpecs_;
bitField0_ = (bitField0_ & ~0x00000001);
} else {
ensureInputFeatureColumnSpecsIsMutable();
inputFeatureColumnSpecs_.addAll(other.inputFeatureColumnSpecs_);
}
onChanged();
}
} else {
if (!other.inputFeatureColumnSpecs_.isEmpty()) {
if (inputFeatureColumnSpecsBuilder_.isEmpty()) {
inputFeatureColumnSpecsBuilder_.dispose();
inputFeatureColumnSpecsBuilder_ = null;
inputFeatureColumnSpecs_ = other.inputFeatureColumnSpecs_;
bitField0_ = (bitField0_ & ~0x00000001);
inputFeatureColumnSpecsBuilder_ =
com.google.protobuf.GeneratedMessageV3.alwaysUseFieldBuilders
? getInputFeatureColumnSpecsFieldBuilder()
: null;
} else {
inputFeatureColumnSpecsBuilder_.addAllMessages(other.inputFeatureColumnSpecs_);
}
}
}
if (!other.getOptimizationObjective().isEmpty()) {
optimizationObjective_ = other.optimizationObjective_;
onChanged();
}
if (tablesModelColumnInfoBuilder_ == null) {
if (!other.tablesModelColumnInfo_.isEmpty()) {
if (tablesModelColumnInfo_.isEmpty()) {
tablesModelColumnInfo_ = other.tablesModelColumnInfo_;
bitField0_ = (bitField0_ & ~0x00000002);
} else {
ensureTablesModelColumnInfoIsMutable();
tablesModelColumnInfo_.addAll(other.tablesModelColumnInfo_);
}
onChanged();
}
} else {
if (!other.tablesModelColumnInfo_.isEmpty()) {
if (tablesModelColumnInfoBuilder_.isEmpty()) {
tablesModelColumnInfoBuilder_.dispose();
tablesModelColumnInfoBuilder_ = null;
tablesModelColumnInfo_ = other.tablesModelColumnInfo_;
bitField0_ = (bitField0_ & ~0x00000002);
tablesModelColumnInfoBuilder_ =
com.google.protobuf.GeneratedMessageV3.alwaysUseFieldBuilders
? getTablesModelColumnInfoFieldBuilder()
: null;
} else {
tablesModelColumnInfoBuilder_.addAllMessages(other.tablesModelColumnInfo_);
}
}
}
if (other.getTrainBudgetMilliNodeHours() != 0L) {
setTrainBudgetMilliNodeHours(other.getTrainBudgetMilliNodeHours());
}
if (other.getTrainCostMilliNodeHours() != 0L) {
setTrainCostMilliNodeHours(other.getTrainCostMilliNodeHours());
}
if (other.getDisableEarlyStopping() != false) {
setDisableEarlyStopping(other.getDisableEarlyStopping());
}
switch (other.getAdditionalOptimizationObjectiveConfigCase()) {
case OPTIMIZATION_OBJECTIVE_RECALL_VALUE:
{
setOptimizationObjectiveRecallValue(other.getOptimizationObjectiveRecallValue());
break;
}
case OPTIMIZATION_OBJECTIVE_PRECISION_VALUE:
{
setOptimizationObjectivePrecisionValue(other.getOptimizationObjectivePrecisionValue());
break;
}
case ADDITIONALOPTIMIZATIONOBJECTIVECONFIG_NOT_SET:
{
break;
}
}
this.mergeUnknownFields(other.getUnknownFields());
onChanged();
return this;
}
@java.lang.Override
public final boolean isInitialized() {
return true;
}
@java.lang.Override
public Builder mergeFrom(
com.google.protobuf.CodedInputStream input,
com.google.protobuf.ExtensionRegistryLite extensionRegistry)
throws java.io.IOException {
if (extensionRegistry == null) {
throw new java.lang.NullPointerException();
}
try {
boolean done = false;
while (!done) {
int tag = input.readTag();
switch (tag) {
case 0:
done = true;
break;
case 18:
{
input.readMessage(
getTargetColumnSpecFieldBuilder().getBuilder(), extensionRegistry);
break;
} // case 18
case 26:
{
com.google.cloud.automl.v1beta1.ColumnSpec m =
input.readMessage(
com.google.cloud.automl.v1beta1.ColumnSpec.parser(), extensionRegistry);
if (inputFeatureColumnSpecsBuilder_ == null) {
ensureInputFeatureColumnSpecsIsMutable();
inputFeatureColumnSpecs_.add(m);
} else {
inputFeatureColumnSpecsBuilder_.addMessage(m);
}
break;
} // case 26
case 34:
{
optimizationObjective_ = input.readStringRequireUtf8();
break;
} // case 34
case 42:
{
com.google.cloud.automl.v1beta1.TablesModelColumnInfo m =
input.readMessage(
com.google.cloud.automl.v1beta1.TablesModelColumnInfo.parser(),
extensionRegistry);
if (tablesModelColumnInfoBuilder_ == null) {
ensureTablesModelColumnInfoIsMutable();
tablesModelColumnInfo_.add(m);
} else {
tablesModelColumnInfoBuilder_.addMessage(m);
}
break;
} // case 42
case 48:
{
trainBudgetMilliNodeHours_ = input.readInt64();
break;
} // case 48
case 56:
{
trainCostMilliNodeHours_ = input.readInt64();
break;
} // case 56
case 96:
{
disableEarlyStopping_ = input.readBool();
break;
} // case 96
case 141:
{
additionalOptimizationObjectiveConfig_ = input.readFloat();
additionalOptimizationObjectiveConfigCase_ = 17;
break;
} // case 141
case 149:
{
additionalOptimizationObjectiveConfig_ = input.readFloat();
additionalOptimizationObjectiveConfigCase_ = 18;
break;
} // case 149
default:
{
if (!super.parseUnknownField(input, extensionRegistry, tag)) {
done = true; // was an endgroup tag
}
break;
} // default:
} // switch (tag)
} // while (!done)
} catch (com.google.protobuf.InvalidProtocolBufferException e) {
throw e.unwrapIOException();
} finally {
onChanged();
} // finally
return this;
}
private int additionalOptimizationObjectiveConfigCase_ = 0;
private java.lang.Object additionalOptimizationObjectiveConfig_;
public AdditionalOptimizationObjectiveConfigCase
getAdditionalOptimizationObjectiveConfigCase() {
return AdditionalOptimizationObjectiveConfigCase.forNumber(
additionalOptimizationObjectiveConfigCase_);
}
public Builder clearAdditionalOptimizationObjectiveConfig() {
additionalOptimizationObjectiveConfigCase_ = 0;
additionalOptimizationObjectiveConfig_ = null;
onChanged();
return this;
}
private int bitField0_;
/**
*
*
*
* Required when optimization_objective is "MAXIMIZE_PRECISION_AT_RECALL".
* Must be between 0 and 1, inclusive.
*
*
* float optimization_objective_recall_value = 17;
*
* @return Whether the optimizationObjectiveRecallValue field is set.
*/
public boolean hasOptimizationObjectiveRecallValue() {
return additionalOptimizationObjectiveConfigCase_ == 17;
}
/**
*
*
*
* Required when optimization_objective is "MAXIMIZE_PRECISION_AT_RECALL".
* Must be between 0 and 1, inclusive.
*
*
* float optimization_objective_recall_value = 17;
*
* @return The optimizationObjectiveRecallValue.
*/
public float getOptimizationObjectiveRecallValue() {
if (additionalOptimizationObjectiveConfigCase_ == 17) {
return (java.lang.Float) additionalOptimizationObjectiveConfig_;
}
return 0F;
}
/**
*
*
*
* Required when optimization_objective is "MAXIMIZE_PRECISION_AT_RECALL".
* Must be between 0 and 1, inclusive.
*
*
* float optimization_objective_recall_value = 17;
*
* @param value The optimizationObjectiveRecallValue to set.
* @return This builder for chaining.
*/
public Builder setOptimizationObjectiveRecallValue(float value) {
additionalOptimizationObjectiveConfigCase_ = 17;
additionalOptimizationObjectiveConfig_ = value;
onChanged();
return this;
}
/**
*
*
*
* Required when optimization_objective is "MAXIMIZE_PRECISION_AT_RECALL".
* Must be between 0 and 1, inclusive.
*
*
* float optimization_objective_recall_value = 17;
*
* @return This builder for chaining.
*/
public Builder clearOptimizationObjectiveRecallValue() {
if (additionalOptimizationObjectiveConfigCase_ == 17) {
additionalOptimizationObjectiveConfigCase_ = 0;
additionalOptimizationObjectiveConfig_ = null;
onChanged();
}
return this;
}
/**
*
*
*
* Required when optimization_objective is "MAXIMIZE_RECALL_AT_PRECISION".
* Must be between 0 and 1, inclusive.
*
*
* float optimization_objective_precision_value = 18;
*
* @return Whether the optimizationObjectivePrecisionValue field is set.
*/
public boolean hasOptimizationObjectivePrecisionValue() {
return additionalOptimizationObjectiveConfigCase_ == 18;
}
/**
*
*
*
* Required when optimization_objective is "MAXIMIZE_RECALL_AT_PRECISION".
* Must be between 0 and 1, inclusive.
*
*
* float optimization_objective_precision_value = 18;
*
* @return The optimizationObjectivePrecisionValue.
*/
public float getOptimizationObjectivePrecisionValue() {
if (additionalOptimizationObjectiveConfigCase_ == 18) {
return (java.lang.Float) additionalOptimizationObjectiveConfig_;
}
return 0F;
}
/**
*
*
*
* Required when optimization_objective is "MAXIMIZE_RECALL_AT_PRECISION".
* Must be between 0 and 1, inclusive.
*
*
* float optimization_objective_precision_value = 18;
*
* @param value The optimizationObjectivePrecisionValue to set.
* @return This builder for chaining.
*/
public Builder setOptimizationObjectivePrecisionValue(float value) {
additionalOptimizationObjectiveConfigCase_ = 18;
additionalOptimizationObjectiveConfig_ = value;
onChanged();
return this;
}
/**
*
*
*
* Required when optimization_objective is "MAXIMIZE_RECALL_AT_PRECISION".
* Must be between 0 and 1, inclusive.
*
*
* float optimization_objective_precision_value = 18;
*
* @return This builder for chaining.
*/
public Builder clearOptimizationObjectivePrecisionValue() {
if (additionalOptimizationObjectiveConfigCase_ == 18) {
additionalOptimizationObjectiveConfigCase_ = 0;
additionalOptimizationObjectiveConfig_ = null;
onChanged();
}
return this;
}
private com.google.cloud.automl.v1beta1.ColumnSpec targetColumnSpec_;
private com.google.protobuf.SingleFieldBuilderV3<
com.google.cloud.automl.v1beta1.ColumnSpec,
com.google.cloud.automl.v1beta1.ColumnSpec.Builder,
com.google.cloud.automl.v1beta1.ColumnSpecOrBuilder>
targetColumnSpecBuilder_;
/**
*
*
*
* Column spec of the dataset's primary table's column the model is
* predicting. Snapshotted when model creation started.
* Only 3 fields are used:
* name - May be set on CreateModel, if it's not then the ColumnSpec
* corresponding to the current target_column_spec_id of the dataset
* the model is trained from is used.
* If neither is set, CreateModel will error.
* display_name - Output only.
* data_type - Output only.
*
*
* .google.cloud.automl.v1beta1.ColumnSpec target_column_spec = 2;
*
* @return Whether the targetColumnSpec field is set.
*/
public boolean hasTargetColumnSpec() {
return targetColumnSpecBuilder_ != null || targetColumnSpec_ != null;
}
/**
*
*
*
* Column spec of the dataset's primary table's column the model is
* predicting. Snapshotted when model creation started.
* Only 3 fields are used:
* name - May be set on CreateModel, if it's not then the ColumnSpec
* corresponding to the current target_column_spec_id of the dataset
* the model is trained from is used.
* If neither is set, CreateModel will error.
* display_name - Output only.
* data_type - Output only.
*
*
* .google.cloud.automl.v1beta1.ColumnSpec target_column_spec = 2;
*
* @return The targetColumnSpec.
*/
public com.google.cloud.automl.v1beta1.ColumnSpec getTargetColumnSpec() {
if (targetColumnSpecBuilder_ == null) {
return targetColumnSpec_ == null
? com.google.cloud.automl.v1beta1.ColumnSpec.getDefaultInstance()
: targetColumnSpec_;
} else {
return targetColumnSpecBuilder_.getMessage();
}
}
/**
*
*
*
* Column spec of the dataset's primary table's column the model is
* predicting. Snapshotted when model creation started.
* Only 3 fields are used:
* name - May be set on CreateModel, if it's not then the ColumnSpec
* corresponding to the current target_column_spec_id of the dataset
* the model is trained from is used.
* If neither is set, CreateModel will error.
* display_name - Output only.
* data_type - Output only.
*
*
* .google.cloud.automl.v1beta1.ColumnSpec target_column_spec = 2;
*/
public Builder setTargetColumnSpec(com.google.cloud.automl.v1beta1.ColumnSpec value) {
if (targetColumnSpecBuilder_ == null) {
if (value == null) {
throw new NullPointerException();
}
targetColumnSpec_ = value;
onChanged();
} else {
targetColumnSpecBuilder_.setMessage(value);
}
return this;
}
/**
*
*
*
* Column spec of the dataset's primary table's column the model is
* predicting. Snapshotted when model creation started.
* Only 3 fields are used:
* name - May be set on CreateModel, if it's not then the ColumnSpec
* corresponding to the current target_column_spec_id of the dataset
* the model is trained from is used.
* If neither is set, CreateModel will error.
* display_name - Output only.
* data_type - Output only.
*
*
* .google.cloud.automl.v1beta1.ColumnSpec target_column_spec = 2;
*/
public Builder setTargetColumnSpec(
com.google.cloud.automl.v1beta1.ColumnSpec.Builder builderForValue) {
if (targetColumnSpecBuilder_ == null) {
targetColumnSpec_ = builderForValue.build();
onChanged();
} else {
targetColumnSpecBuilder_.setMessage(builderForValue.build());
}
return this;
}
/**
*
*
*
* Column spec of the dataset's primary table's column the model is
* predicting. Snapshotted when model creation started.
* Only 3 fields are used:
* name - May be set on CreateModel, if it's not then the ColumnSpec
* corresponding to the current target_column_spec_id of the dataset
* the model is trained from is used.
* If neither is set, CreateModel will error.
* display_name - Output only.
* data_type - Output only.
*
*
* .google.cloud.automl.v1beta1.ColumnSpec target_column_spec = 2;
*/
public Builder mergeTargetColumnSpec(com.google.cloud.automl.v1beta1.ColumnSpec value) {
if (targetColumnSpecBuilder_ == null) {
if (targetColumnSpec_ != null) {
targetColumnSpec_ =
com.google.cloud.automl.v1beta1.ColumnSpec.newBuilder(targetColumnSpec_)
.mergeFrom(value)
.buildPartial();
} else {
targetColumnSpec_ = value;
}
onChanged();
} else {
targetColumnSpecBuilder_.mergeFrom(value);
}
return this;
}
/**
*
*
*
* Column spec of the dataset's primary table's column the model is
* predicting. Snapshotted when model creation started.
* Only 3 fields are used:
* name - May be set on CreateModel, if it's not then the ColumnSpec
* corresponding to the current target_column_spec_id of the dataset
* the model is trained from is used.
* If neither is set, CreateModel will error.
* display_name - Output only.
* data_type - Output only.
*
*
* .google.cloud.automl.v1beta1.ColumnSpec target_column_spec = 2;
*/
public Builder clearTargetColumnSpec() {
if (targetColumnSpecBuilder_ == null) {
targetColumnSpec_ = null;
onChanged();
} else {
targetColumnSpec_ = null;
targetColumnSpecBuilder_ = null;
}
return this;
}
/**
*
*
*
* Column spec of the dataset's primary table's column the model is
* predicting. Snapshotted when model creation started.
* Only 3 fields are used:
* name - May be set on CreateModel, if it's not then the ColumnSpec
* corresponding to the current target_column_spec_id of the dataset
* the model is trained from is used.
* If neither is set, CreateModel will error.
* display_name - Output only.
* data_type - Output only.
*
*
* .google.cloud.automl.v1beta1.ColumnSpec target_column_spec = 2;
*/
public com.google.cloud.automl.v1beta1.ColumnSpec.Builder getTargetColumnSpecBuilder() {
onChanged();
return getTargetColumnSpecFieldBuilder().getBuilder();
}
/**
*
*
*
* Column spec of the dataset's primary table's column the model is
* predicting. Snapshotted when model creation started.
* Only 3 fields are used:
* name - May be set on CreateModel, if it's not then the ColumnSpec
* corresponding to the current target_column_spec_id of the dataset
* the model is trained from is used.
* If neither is set, CreateModel will error.
* display_name - Output only.
* data_type - Output only.
*
*
* .google.cloud.automl.v1beta1.ColumnSpec target_column_spec = 2;
*/
public com.google.cloud.automl.v1beta1.ColumnSpecOrBuilder getTargetColumnSpecOrBuilder() {
if (targetColumnSpecBuilder_ != null) {
return targetColumnSpecBuilder_.getMessageOrBuilder();
} else {
return targetColumnSpec_ == null
? com.google.cloud.automl.v1beta1.ColumnSpec.getDefaultInstance()
: targetColumnSpec_;
}
}
/**
*
*
*
* Column spec of the dataset's primary table's column the model is
* predicting. Snapshotted when model creation started.
* Only 3 fields are used:
* name - May be set on CreateModel, if it's not then the ColumnSpec
* corresponding to the current target_column_spec_id of the dataset
* the model is trained from is used.
* If neither is set, CreateModel will error.
* display_name - Output only.
* data_type - Output only.
*
*
* .google.cloud.automl.v1beta1.ColumnSpec target_column_spec = 2;
*/
private com.google.protobuf.SingleFieldBuilderV3<
com.google.cloud.automl.v1beta1.ColumnSpec,
com.google.cloud.automl.v1beta1.ColumnSpec.Builder,
com.google.cloud.automl.v1beta1.ColumnSpecOrBuilder>
getTargetColumnSpecFieldBuilder() {
if (targetColumnSpecBuilder_ == null) {
targetColumnSpecBuilder_ =
new com.google.protobuf.SingleFieldBuilderV3<
com.google.cloud.automl.v1beta1.ColumnSpec,
com.google.cloud.automl.v1beta1.ColumnSpec.Builder,
com.google.cloud.automl.v1beta1.ColumnSpecOrBuilder>(
getTargetColumnSpec(), getParentForChildren(), isClean());
targetColumnSpec_ = null;
}
return targetColumnSpecBuilder_;
}
private java.util.List inputFeatureColumnSpecs_ =
java.util.Collections.emptyList();
private void ensureInputFeatureColumnSpecsIsMutable() {
if (!((bitField0_ & 0x00000001) != 0)) {
inputFeatureColumnSpecs_ =
new java.util.ArrayList(
inputFeatureColumnSpecs_);
bitField0_ |= 0x00000001;
}
}
private com.google.protobuf.RepeatedFieldBuilderV3<
com.google.cloud.automl.v1beta1.ColumnSpec,
com.google.cloud.automl.v1beta1.ColumnSpec.Builder,
com.google.cloud.automl.v1beta1.ColumnSpecOrBuilder>
inputFeatureColumnSpecsBuilder_;
/**
*
*
*
* Column specs of the dataset's primary table's columns, on which
* the model is trained and which are used as the input for predictions.
* The
* [target_column][google.cloud.automl.v1beta1.TablesModelMetadata.target_column_spec]
* as well as, according to dataset's state upon model creation,
* [weight_column][google.cloud.automl.v1beta1.TablesDatasetMetadata.weight_column_spec_id],
* and
* [ml_use_column][google.cloud.automl.v1beta1.TablesDatasetMetadata.ml_use_column_spec_id]
* must never be included here.
* Only 3 fields are used:
* * name - May be set on CreateModel, if set only the columns specified are
* used, otherwise all primary table's columns (except the ones listed
* above) are used for the training and prediction input.
* * display_name - Output only.
* * data_type - Output only.
*
*
* repeated .google.cloud.automl.v1beta1.ColumnSpec input_feature_column_specs = 3;
*/
public java.util.List
getInputFeatureColumnSpecsList() {
if (inputFeatureColumnSpecsBuilder_ == null) {
return java.util.Collections.unmodifiableList(inputFeatureColumnSpecs_);
} else {
return inputFeatureColumnSpecsBuilder_.getMessageList();
}
}
/**
*
*
*
* Column specs of the dataset's primary table's columns, on which
* the model is trained and which are used as the input for predictions.
* The
* [target_column][google.cloud.automl.v1beta1.TablesModelMetadata.target_column_spec]
* as well as, according to dataset's state upon model creation,
* [weight_column][google.cloud.automl.v1beta1.TablesDatasetMetadata.weight_column_spec_id],
* and
* [ml_use_column][google.cloud.automl.v1beta1.TablesDatasetMetadata.ml_use_column_spec_id]
* must never be included here.
* Only 3 fields are used:
* * name - May be set on CreateModel, if set only the columns specified are
* used, otherwise all primary table's columns (except the ones listed
* above) are used for the training and prediction input.
* * display_name - Output only.
* * data_type - Output only.
*
*
* repeated .google.cloud.automl.v1beta1.ColumnSpec input_feature_column_specs = 3;
*/
public int getInputFeatureColumnSpecsCount() {
if (inputFeatureColumnSpecsBuilder_ == null) {
return inputFeatureColumnSpecs_.size();
} else {
return inputFeatureColumnSpecsBuilder_.getCount();
}
}
/**
*
*
*
* Column specs of the dataset's primary table's columns, on which
* the model is trained and which are used as the input for predictions.
* The
* [target_column][google.cloud.automl.v1beta1.TablesModelMetadata.target_column_spec]
* as well as, according to dataset's state upon model creation,
* [weight_column][google.cloud.automl.v1beta1.TablesDatasetMetadata.weight_column_spec_id],
* and
* [ml_use_column][google.cloud.automl.v1beta1.TablesDatasetMetadata.ml_use_column_spec_id]
* must never be included here.
* Only 3 fields are used:
* * name - May be set on CreateModel, if set only the columns specified are
* used, otherwise all primary table's columns (except the ones listed
* above) are used for the training and prediction input.
* * display_name - Output only.
* * data_type - Output only.
*
*
* repeated .google.cloud.automl.v1beta1.ColumnSpec input_feature_column_specs = 3;
*/
public com.google.cloud.automl.v1beta1.ColumnSpec getInputFeatureColumnSpecs(int index) {
if (inputFeatureColumnSpecsBuilder_ == null) {
return inputFeatureColumnSpecs_.get(index);
} else {
return inputFeatureColumnSpecsBuilder_.getMessage(index);
}
}
/**
*
*
*
* Column specs of the dataset's primary table's columns, on which
* the model is trained and which are used as the input for predictions.
* The
* [target_column][google.cloud.automl.v1beta1.TablesModelMetadata.target_column_spec]
* as well as, according to dataset's state upon model creation,
* [weight_column][google.cloud.automl.v1beta1.TablesDatasetMetadata.weight_column_spec_id],
* and
* [ml_use_column][google.cloud.automl.v1beta1.TablesDatasetMetadata.ml_use_column_spec_id]
* must never be included here.
* Only 3 fields are used:
* * name - May be set on CreateModel, if set only the columns specified are
* used, otherwise all primary table's columns (except the ones listed
* above) are used for the training and prediction input.
* * display_name - Output only.
* * data_type - Output only.
*
*
* repeated .google.cloud.automl.v1beta1.ColumnSpec input_feature_column_specs = 3;
*/
public Builder setInputFeatureColumnSpecs(
int index, com.google.cloud.automl.v1beta1.ColumnSpec value) {
if (inputFeatureColumnSpecsBuilder_ == null) {
if (value == null) {
throw new NullPointerException();
}
ensureInputFeatureColumnSpecsIsMutable();
inputFeatureColumnSpecs_.set(index, value);
onChanged();
} else {
inputFeatureColumnSpecsBuilder_.setMessage(index, value);
}
return this;
}
/**
*
*
*
* Column specs of the dataset's primary table's columns, on which
* the model is trained and which are used as the input for predictions.
* The
* [target_column][google.cloud.automl.v1beta1.TablesModelMetadata.target_column_spec]
* as well as, according to dataset's state upon model creation,
* [weight_column][google.cloud.automl.v1beta1.TablesDatasetMetadata.weight_column_spec_id],
* and
* [ml_use_column][google.cloud.automl.v1beta1.TablesDatasetMetadata.ml_use_column_spec_id]
* must never be included here.
* Only 3 fields are used:
* * name - May be set on CreateModel, if set only the columns specified are
* used, otherwise all primary table's columns (except the ones listed
* above) are used for the training and prediction input.
* * display_name - Output only.
* * data_type - Output only.
*
*
* repeated .google.cloud.automl.v1beta1.ColumnSpec input_feature_column_specs = 3;
*/
public Builder setInputFeatureColumnSpecs(
int index, com.google.cloud.automl.v1beta1.ColumnSpec.Builder builderForValue) {
if (inputFeatureColumnSpecsBuilder_ == null) {
ensureInputFeatureColumnSpecsIsMutable();
inputFeatureColumnSpecs_.set(index, builderForValue.build());
onChanged();
} else {
inputFeatureColumnSpecsBuilder_.setMessage(index, builderForValue.build());
}
return this;
}
/**
*
*
*
* Column specs of the dataset's primary table's columns, on which
* the model is trained and which are used as the input for predictions.
* The
* [target_column][google.cloud.automl.v1beta1.TablesModelMetadata.target_column_spec]
* as well as, according to dataset's state upon model creation,
* [weight_column][google.cloud.automl.v1beta1.TablesDatasetMetadata.weight_column_spec_id],
* and
* [ml_use_column][google.cloud.automl.v1beta1.TablesDatasetMetadata.ml_use_column_spec_id]
* must never be included here.
* Only 3 fields are used:
* * name - May be set on CreateModel, if set only the columns specified are
* used, otherwise all primary table's columns (except the ones listed
* above) are used for the training and prediction input.
* * display_name - Output only.
* * data_type - Output only.
*
*
* repeated .google.cloud.automl.v1beta1.ColumnSpec input_feature_column_specs = 3;
*/
public Builder addInputFeatureColumnSpecs(com.google.cloud.automl.v1beta1.ColumnSpec value) {
if (inputFeatureColumnSpecsBuilder_ == null) {
if (value == null) {
throw new NullPointerException();
}
ensureInputFeatureColumnSpecsIsMutable();
inputFeatureColumnSpecs_.add(value);
onChanged();
} else {
inputFeatureColumnSpecsBuilder_.addMessage(value);
}
return this;
}
/**
*
*
*
* Column specs of the dataset's primary table's columns, on which
* the model is trained and which are used as the input for predictions.
* The
* [target_column][google.cloud.automl.v1beta1.TablesModelMetadata.target_column_spec]
* as well as, according to dataset's state upon model creation,
* [weight_column][google.cloud.automl.v1beta1.TablesDatasetMetadata.weight_column_spec_id],
* and
* [ml_use_column][google.cloud.automl.v1beta1.TablesDatasetMetadata.ml_use_column_spec_id]
* must never be included here.
* Only 3 fields are used:
* * name - May be set on CreateModel, if set only the columns specified are
* used, otherwise all primary table's columns (except the ones listed
* above) are used for the training and prediction input.
* * display_name - Output only.
* * data_type - Output only.
*
*
* repeated .google.cloud.automl.v1beta1.ColumnSpec input_feature_column_specs = 3;
*/
public Builder addInputFeatureColumnSpecs(
int index, com.google.cloud.automl.v1beta1.ColumnSpec value) {
if (inputFeatureColumnSpecsBuilder_ == null) {
if (value == null) {
throw new NullPointerException();
}
ensureInputFeatureColumnSpecsIsMutable();
inputFeatureColumnSpecs_.add(index, value);
onChanged();
} else {
inputFeatureColumnSpecsBuilder_.addMessage(index, value);
}
return this;
}
/**
*
*
*
* Column specs of the dataset's primary table's columns, on which
* the model is trained and which are used as the input for predictions.
* The
* [target_column][google.cloud.automl.v1beta1.TablesModelMetadata.target_column_spec]
* as well as, according to dataset's state upon model creation,
* [weight_column][google.cloud.automl.v1beta1.TablesDatasetMetadata.weight_column_spec_id],
* and
* [ml_use_column][google.cloud.automl.v1beta1.TablesDatasetMetadata.ml_use_column_spec_id]
* must never be included here.
* Only 3 fields are used:
* * name - May be set on CreateModel, if set only the columns specified are
* used, otherwise all primary table's columns (except the ones listed
* above) are used for the training and prediction input.
* * display_name - Output only.
* * data_type - Output only.
*
*
* repeated .google.cloud.automl.v1beta1.ColumnSpec input_feature_column_specs = 3;
*/
public Builder addInputFeatureColumnSpecs(
com.google.cloud.automl.v1beta1.ColumnSpec.Builder builderForValue) {
if (inputFeatureColumnSpecsBuilder_ == null) {
ensureInputFeatureColumnSpecsIsMutable();
inputFeatureColumnSpecs_.add(builderForValue.build());
onChanged();
} else {
inputFeatureColumnSpecsBuilder_.addMessage(builderForValue.build());
}
return this;
}
/**
*
*
*
* Column specs of the dataset's primary table's columns, on which
* the model is trained and which are used as the input for predictions.
* The
* [target_column][google.cloud.automl.v1beta1.TablesModelMetadata.target_column_spec]
* as well as, according to dataset's state upon model creation,
* [weight_column][google.cloud.automl.v1beta1.TablesDatasetMetadata.weight_column_spec_id],
* and
* [ml_use_column][google.cloud.automl.v1beta1.TablesDatasetMetadata.ml_use_column_spec_id]
* must never be included here.
* Only 3 fields are used:
* * name - May be set on CreateModel, if set only the columns specified are
* used, otherwise all primary table's columns (except the ones listed
* above) are used for the training and prediction input.
* * display_name - Output only.
* * data_type - Output only.
*
*
* repeated .google.cloud.automl.v1beta1.ColumnSpec input_feature_column_specs = 3;
*/
public Builder addInputFeatureColumnSpecs(
int index, com.google.cloud.automl.v1beta1.ColumnSpec.Builder builderForValue) {
if (inputFeatureColumnSpecsBuilder_ == null) {
ensureInputFeatureColumnSpecsIsMutable();
inputFeatureColumnSpecs_.add(index, builderForValue.build());
onChanged();
} else {
inputFeatureColumnSpecsBuilder_.addMessage(index, builderForValue.build());
}
return this;
}
/**
*
*
*
* Column specs of the dataset's primary table's columns, on which
* the model is trained and which are used as the input for predictions.
* The
* [target_column][google.cloud.automl.v1beta1.TablesModelMetadata.target_column_spec]
* as well as, according to dataset's state upon model creation,
* [weight_column][google.cloud.automl.v1beta1.TablesDatasetMetadata.weight_column_spec_id],
* and
* [ml_use_column][google.cloud.automl.v1beta1.TablesDatasetMetadata.ml_use_column_spec_id]
* must never be included here.
* Only 3 fields are used:
* * name - May be set on CreateModel, if set only the columns specified are
* used, otherwise all primary table's columns (except the ones listed
* above) are used for the training and prediction input.
* * display_name - Output only.
* * data_type - Output only.
*
*
* repeated .google.cloud.automl.v1beta1.ColumnSpec input_feature_column_specs = 3;
*/
public Builder addAllInputFeatureColumnSpecs(
java.lang.Iterable extends com.google.cloud.automl.v1beta1.ColumnSpec> values) {
if (inputFeatureColumnSpecsBuilder_ == null) {
ensureInputFeatureColumnSpecsIsMutable();
com.google.protobuf.AbstractMessageLite.Builder.addAll(values, inputFeatureColumnSpecs_);
onChanged();
} else {
inputFeatureColumnSpecsBuilder_.addAllMessages(values);
}
return this;
}
/**
*
*
*
* Column specs of the dataset's primary table's columns, on which
* the model is trained and which are used as the input for predictions.
* The
* [target_column][google.cloud.automl.v1beta1.TablesModelMetadata.target_column_spec]
* as well as, according to dataset's state upon model creation,
* [weight_column][google.cloud.automl.v1beta1.TablesDatasetMetadata.weight_column_spec_id],
* and
* [ml_use_column][google.cloud.automl.v1beta1.TablesDatasetMetadata.ml_use_column_spec_id]
* must never be included here.
* Only 3 fields are used:
* * name - May be set on CreateModel, if set only the columns specified are
* used, otherwise all primary table's columns (except the ones listed
* above) are used for the training and prediction input.
* * display_name - Output only.
* * data_type - Output only.
*
*
* repeated .google.cloud.automl.v1beta1.ColumnSpec input_feature_column_specs = 3;
*/
public Builder clearInputFeatureColumnSpecs() {
if (inputFeatureColumnSpecsBuilder_ == null) {
inputFeatureColumnSpecs_ = java.util.Collections.emptyList();
bitField0_ = (bitField0_ & ~0x00000001);
onChanged();
} else {
inputFeatureColumnSpecsBuilder_.clear();
}
return this;
}
/**
*
*
*
* Column specs of the dataset's primary table's columns, on which
* the model is trained and which are used as the input for predictions.
* The
* [target_column][google.cloud.automl.v1beta1.TablesModelMetadata.target_column_spec]
* as well as, according to dataset's state upon model creation,
* [weight_column][google.cloud.automl.v1beta1.TablesDatasetMetadata.weight_column_spec_id],
* and
* [ml_use_column][google.cloud.automl.v1beta1.TablesDatasetMetadata.ml_use_column_spec_id]
* must never be included here.
* Only 3 fields are used:
* * name - May be set on CreateModel, if set only the columns specified are
* used, otherwise all primary table's columns (except the ones listed
* above) are used for the training and prediction input.
* * display_name - Output only.
* * data_type - Output only.
*
*
* repeated .google.cloud.automl.v1beta1.ColumnSpec input_feature_column_specs = 3;
*/
public Builder removeInputFeatureColumnSpecs(int index) {
if (inputFeatureColumnSpecsBuilder_ == null) {
ensureInputFeatureColumnSpecsIsMutable();
inputFeatureColumnSpecs_.remove(index);
onChanged();
} else {
inputFeatureColumnSpecsBuilder_.remove(index);
}
return this;
}
/**
*
*
*
* Column specs of the dataset's primary table's columns, on which
* the model is trained and which are used as the input for predictions.
* The
* [target_column][google.cloud.automl.v1beta1.TablesModelMetadata.target_column_spec]
* as well as, according to dataset's state upon model creation,
* [weight_column][google.cloud.automl.v1beta1.TablesDatasetMetadata.weight_column_spec_id],
* and
* [ml_use_column][google.cloud.automl.v1beta1.TablesDatasetMetadata.ml_use_column_spec_id]
* must never be included here.
* Only 3 fields are used:
* * name - May be set on CreateModel, if set only the columns specified are
* used, otherwise all primary table's columns (except the ones listed
* above) are used for the training and prediction input.
* * display_name - Output only.
* * data_type - Output only.
*
*
* repeated .google.cloud.automl.v1beta1.ColumnSpec input_feature_column_specs = 3;
*/
public com.google.cloud.automl.v1beta1.ColumnSpec.Builder getInputFeatureColumnSpecsBuilder(
int index) {
return getInputFeatureColumnSpecsFieldBuilder().getBuilder(index);
}
/**
*
*
*
* Column specs of the dataset's primary table's columns, on which
* the model is trained and which are used as the input for predictions.
* The
* [target_column][google.cloud.automl.v1beta1.TablesModelMetadata.target_column_spec]
* as well as, according to dataset's state upon model creation,
* [weight_column][google.cloud.automl.v1beta1.TablesDatasetMetadata.weight_column_spec_id],
* and
* [ml_use_column][google.cloud.automl.v1beta1.TablesDatasetMetadata.ml_use_column_spec_id]
* must never be included here.
* Only 3 fields are used:
* * name - May be set on CreateModel, if set only the columns specified are
* used, otherwise all primary table's columns (except the ones listed
* above) are used for the training and prediction input.
* * display_name - Output only.
* * data_type - Output only.
*
*
* repeated .google.cloud.automl.v1beta1.ColumnSpec input_feature_column_specs = 3;
*/
public com.google.cloud.automl.v1beta1.ColumnSpecOrBuilder getInputFeatureColumnSpecsOrBuilder(
int index) {
if (inputFeatureColumnSpecsBuilder_ == null) {
return inputFeatureColumnSpecs_.get(index);
} else {
return inputFeatureColumnSpecsBuilder_.getMessageOrBuilder(index);
}
}
/**
*
*
*
* Column specs of the dataset's primary table's columns, on which
* the model is trained and which are used as the input for predictions.
* The
* [target_column][google.cloud.automl.v1beta1.TablesModelMetadata.target_column_spec]
* as well as, according to dataset's state upon model creation,
* [weight_column][google.cloud.automl.v1beta1.TablesDatasetMetadata.weight_column_spec_id],
* and
* [ml_use_column][google.cloud.automl.v1beta1.TablesDatasetMetadata.ml_use_column_spec_id]
* must never be included here.
* Only 3 fields are used:
* * name - May be set on CreateModel, if set only the columns specified are
* used, otherwise all primary table's columns (except the ones listed
* above) are used for the training and prediction input.
* * display_name - Output only.
* * data_type - Output only.
*
*
* repeated .google.cloud.automl.v1beta1.ColumnSpec input_feature_column_specs = 3;
*/
public java.util.List extends com.google.cloud.automl.v1beta1.ColumnSpecOrBuilder>
getInputFeatureColumnSpecsOrBuilderList() {
if (inputFeatureColumnSpecsBuilder_ != null) {
return inputFeatureColumnSpecsBuilder_.getMessageOrBuilderList();
} else {
return java.util.Collections.unmodifiableList(inputFeatureColumnSpecs_);
}
}
/**
*
*
*
* Column specs of the dataset's primary table's columns, on which
* the model is trained and which are used as the input for predictions.
* The
* [target_column][google.cloud.automl.v1beta1.TablesModelMetadata.target_column_spec]
* as well as, according to dataset's state upon model creation,
* [weight_column][google.cloud.automl.v1beta1.TablesDatasetMetadata.weight_column_spec_id],
* and
* [ml_use_column][google.cloud.automl.v1beta1.TablesDatasetMetadata.ml_use_column_spec_id]
* must never be included here.
* Only 3 fields are used:
* * name - May be set on CreateModel, if set only the columns specified are
* used, otherwise all primary table's columns (except the ones listed
* above) are used for the training and prediction input.
* * display_name - Output only.
* * data_type - Output only.
*
*
* repeated .google.cloud.automl.v1beta1.ColumnSpec input_feature_column_specs = 3;
*/
public com.google.cloud.automl.v1beta1.ColumnSpec.Builder addInputFeatureColumnSpecsBuilder() {
return getInputFeatureColumnSpecsFieldBuilder()
.addBuilder(com.google.cloud.automl.v1beta1.ColumnSpec.getDefaultInstance());
}
/**
*
*
*
* Column specs of the dataset's primary table's columns, on which
* the model is trained and which are used as the input for predictions.
* The
* [target_column][google.cloud.automl.v1beta1.TablesModelMetadata.target_column_spec]
* as well as, according to dataset's state upon model creation,
* [weight_column][google.cloud.automl.v1beta1.TablesDatasetMetadata.weight_column_spec_id],
* and
* [ml_use_column][google.cloud.automl.v1beta1.TablesDatasetMetadata.ml_use_column_spec_id]
* must never be included here.
* Only 3 fields are used:
* * name - May be set on CreateModel, if set only the columns specified are
* used, otherwise all primary table's columns (except the ones listed
* above) are used for the training and prediction input.
* * display_name - Output only.
* * data_type - Output only.
*
*
* repeated .google.cloud.automl.v1beta1.ColumnSpec input_feature_column_specs = 3;
*/
public com.google.cloud.automl.v1beta1.ColumnSpec.Builder addInputFeatureColumnSpecsBuilder(
int index) {
return getInputFeatureColumnSpecsFieldBuilder()
.addBuilder(index, com.google.cloud.automl.v1beta1.ColumnSpec.getDefaultInstance());
}
/**
*
*
*
* Column specs of the dataset's primary table's columns, on which
* the model is trained and which are used as the input for predictions.
* The
* [target_column][google.cloud.automl.v1beta1.TablesModelMetadata.target_column_spec]
* as well as, according to dataset's state upon model creation,
* [weight_column][google.cloud.automl.v1beta1.TablesDatasetMetadata.weight_column_spec_id],
* and
* [ml_use_column][google.cloud.automl.v1beta1.TablesDatasetMetadata.ml_use_column_spec_id]
* must never be included here.
* Only 3 fields are used:
* * name - May be set on CreateModel, if set only the columns specified are
* used, otherwise all primary table's columns (except the ones listed
* above) are used for the training and prediction input.
* * display_name - Output only.
* * data_type - Output only.
*
*
* repeated .google.cloud.automl.v1beta1.ColumnSpec input_feature_column_specs = 3;
*/
public java.util.List
getInputFeatureColumnSpecsBuilderList() {
return getInputFeatureColumnSpecsFieldBuilder().getBuilderList();
}
private com.google.protobuf.RepeatedFieldBuilderV3<
com.google.cloud.automl.v1beta1.ColumnSpec,
com.google.cloud.automl.v1beta1.ColumnSpec.Builder,
com.google.cloud.automl.v1beta1.ColumnSpecOrBuilder>
getInputFeatureColumnSpecsFieldBuilder() {
if (inputFeatureColumnSpecsBuilder_ == null) {
inputFeatureColumnSpecsBuilder_ =
new com.google.protobuf.RepeatedFieldBuilderV3<
com.google.cloud.automl.v1beta1.ColumnSpec,
com.google.cloud.automl.v1beta1.ColumnSpec.Builder,
com.google.cloud.automl.v1beta1.ColumnSpecOrBuilder>(
inputFeatureColumnSpecs_,
((bitField0_ & 0x00000001) != 0),
getParentForChildren(),
isClean());
inputFeatureColumnSpecs_ = null;
}
return inputFeatureColumnSpecsBuilder_;
}
private java.lang.Object optimizationObjective_ = "";
/**
*
*
*
* Objective function the model is optimizing towards. The training process
* creates a model that maximizes/minimizes the value of the objective
* function over the validation set.
* The supported optimization objectives depend on the prediction type.
* If the field is not set, a default objective function is used.
* CLASSIFICATION_BINARY:
* "MAXIMIZE_AU_ROC" (default) - Maximize the area under the receiver
* operating characteristic (ROC) curve.
* "MINIMIZE_LOG_LOSS" - Minimize log loss.
* "MAXIMIZE_AU_PRC" - Maximize the area under the precision-recall curve.
* "MAXIMIZE_PRECISION_AT_RECALL" - Maximize precision for a specified
* recall value.
* "MAXIMIZE_RECALL_AT_PRECISION" - Maximize recall for a specified
* precision value.
* CLASSIFICATION_MULTI_CLASS :
* "MINIMIZE_LOG_LOSS" (default) - Minimize log loss.
* REGRESSION:
* "MINIMIZE_RMSE" (default) - Minimize root-mean-squared error (RMSE).
* "MINIMIZE_MAE" - Minimize mean-absolute error (MAE).
* "MINIMIZE_RMSLE" - Minimize root-mean-squared log error (RMSLE).
*
*
* string optimization_objective = 4;
*
* @return The optimizationObjective.
*/
public java.lang.String getOptimizationObjective() {
java.lang.Object ref = optimizationObjective_;
if (!(ref instanceof java.lang.String)) {
com.google.protobuf.ByteString bs = (com.google.protobuf.ByteString) ref;
java.lang.String s = bs.toStringUtf8();
optimizationObjective_ = s;
return s;
} else {
return (java.lang.String) ref;
}
}
/**
*
*
*
* Objective function the model is optimizing towards. The training process
* creates a model that maximizes/minimizes the value of the objective
* function over the validation set.
* The supported optimization objectives depend on the prediction type.
* If the field is not set, a default objective function is used.
* CLASSIFICATION_BINARY:
* "MAXIMIZE_AU_ROC" (default) - Maximize the area under the receiver
* operating characteristic (ROC) curve.
* "MINIMIZE_LOG_LOSS" - Minimize log loss.
* "MAXIMIZE_AU_PRC" - Maximize the area under the precision-recall curve.
* "MAXIMIZE_PRECISION_AT_RECALL" - Maximize precision for a specified
* recall value.
* "MAXIMIZE_RECALL_AT_PRECISION" - Maximize recall for a specified
* precision value.
* CLASSIFICATION_MULTI_CLASS :
* "MINIMIZE_LOG_LOSS" (default) - Minimize log loss.
* REGRESSION:
* "MINIMIZE_RMSE" (default) - Minimize root-mean-squared error (RMSE).
* "MINIMIZE_MAE" - Minimize mean-absolute error (MAE).
* "MINIMIZE_RMSLE" - Minimize root-mean-squared log error (RMSLE).
*
*
* string optimization_objective = 4;
*
* @return The bytes for optimizationObjective.
*/
public com.google.protobuf.ByteString getOptimizationObjectiveBytes() {
java.lang.Object ref = optimizationObjective_;
if (ref instanceof String) {
com.google.protobuf.ByteString b =
com.google.protobuf.ByteString.copyFromUtf8((java.lang.String) ref);
optimizationObjective_ = b;
return b;
} else {
return (com.google.protobuf.ByteString) ref;
}
}
/**
*
*
*
* Objective function the model is optimizing towards. The training process
* creates a model that maximizes/minimizes the value of the objective
* function over the validation set.
* The supported optimization objectives depend on the prediction type.
* If the field is not set, a default objective function is used.
* CLASSIFICATION_BINARY:
* "MAXIMIZE_AU_ROC" (default) - Maximize the area under the receiver
* operating characteristic (ROC) curve.
* "MINIMIZE_LOG_LOSS" - Minimize log loss.
* "MAXIMIZE_AU_PRC" - Maximize the area under the precision-recall curve.
* "MAXIMIZE_PRECISION_AT_RECALL" - Maximize precision for a specified
* recall value.
* "MAXIMIZE_RECALL_AT_PRECISION" - Maximize recall for a specified
* precision value.
* CLASSIFICATION_MULTI_CLASS :
* "MINIMIZE_LOG_LOSS" (default) - Minimize log loss.
* REGRESSION:
* "MINIMIZE_RMSE" (default) - Minimize root-mean-squared error (RMSE).
* "MINIMIZE_MAE" - Minimize mean-absolute error (MAE).
* "MINIMIZE_RMSLE" - Minimize root-mean-squared log error (RMSLE).
*
*
* string optimization_objective = 4;
*
* @param value The optimizationObjective to set.
* @return This builder for chaining.
*/
public Builder setOptimizationObjective(java.lang.String value) {
if (value == null) {
throw new NullPointerException();
}
optimizationObjective_ = value;
onChanged();
return this;
}
/**
*
*
*
* Objective function the model is optimizing towards. The training process
* creates a model that maximizes/minimizes the value of the objective
* function over the validation set.
* The supported optimization objectives depend on the prediction type.
* If the field is not set, a default objective function is used.
* CLASSIFICATION_BINARY:
* "MAXIMIZE_AU_ROC" (default) - Maximize the area under the receiver
* operating characteristic (ROC) curve.
* "MINIMIZE_LOG_LOSS" - Minimize log loss.
* "MAXIMIZE_AU_PRC" - Maximize the area under the precision-recall curve.
* "MAXIMIZE_PRECISION_AT_RECALL" - Maximize precision for a specified
* recall value.
* "MAXIMIZE_RECALL_AT_PRECISION" - Maximize recall for a specified
* precision value.
* CLASSIFICATION_MULTI_CLASS :
* "MINIMIZE_LOG_LOSS" (default) - Minimize log loss.
* REGRESSION:
* "MINIMIZE_RMSE" (default) - Minimize root-mean-squared error (RMSE).
* "MINIMIZE_MAE" - Minimize mean-absolute error (MAE).
* "MINIMIZE_RMSLE" - Minimize root-mean-squared log error (RMSLE).
*
*
* string optimization_objective = 4;
*
* @return This builder for chaining.
*/
public Builder clearOptimizationObjective() {
optimizationObjective_ = getDefaultInstance().getOptimizationObjective();
onChanged();
return this;
}
/**
*
*
*
* Objective function the model is optimizing towards. The training process
* creates a model that maximizes/minimizes the value of the objective
* function over the validation set.
* The supported optimization objectives depend on the prediction type.
* If the field is not set, a default objective function is used.
* CLASSIFICATION_BINARY:
* "MAXIMIZE_AU_ROC" (default) - Maximize the area under the receiver
* operating characteristic (ROC) curve.
* "MINIMIZE_LOG_LOSS" - Minimize log loss.
* "MAXIMIZE_AU_PRC" - Maximize the area under the precision-recall curve.
* "MAXIMIZE_PRECISION_AT_RECALL" - Maximize precision for a specified
* recall value.
* "MAXIMIZE_RECALL_AT_PRECISION" - Maximize recall for a specified
* precision value.
* CLASSIFICATION_MULTI_CLASS :
* "MINIMIZE_LOG_LOSS" (default) - Minimize log loss.
* REGRESSION:
* "MINIMIZE_RMSE" (default) - Minimize root-mean-squared error (RMSE).
* "MINIMIZE_MAE" - Minimize mean-absolute error (MAE).
* "MINIMIZE_RMSLE" - Minimize root-mean-squared log error (RMSLE).
*
*
* string optimization_objective = 4;
*
* @param value The bytes for optimizationObjective to set.
* @return This builder for chaining.
*/
public Builder setOptimizationObjectiveBytes(com.google.protobuf.ByteString value) {
if (value == null) {
throw new NullPointerException();
}
checkByteStringIsUtf8(value);
optimizationObjective_ = value;
onChanged();
return this;
}
private java.util.List
tablesModelColumnInfo_ = java.util.Collections.emptyList();
private void ensureTablesModelColumnInfoIsMutable() {
if (!((bitField0_ & 0x00000002) != 0)) {
tablesModelColumnInfo_ =
new java.util.ArrayList(
tablesModelColumnInfo_);
bitField0_ |= 0x00000002;
}
}
private com.google.protobuf.RepeatedFieldBuilderV3<
com.google.cloud.automl.v1beta1.TablesModelColumnInfo,
com.google.cloud.automl.v1beta1.TablesModelColumnInfo.Builder,
com.google.cloud.automl.v1beta1.TablesModelColumnInfoOrBuilder>
tablesModelColumnInfoBuilder_;
/**
*
*
*
* Output only. Auxiliary information for each of the
* input_feature_column_specs with respect to this particular model.
*
*
*
* repeated .google.cloud.automl.v1beta1.TablesModelColumnInfo tables_model_column_info = 5;
*
*/
public java.util.List
getTablesModelColumnInfoList() {
if (tablesModelColumnInfoBuilder_ == null) {
return java.util.Collections.unmodifiableList(tablesModelColumnInfo_);
} else {
return tablesModelColumnInfoBuilder_.getMessageList();
}
}
/**
*
*
*
* Output only. Auxiliary information for each of the
* input_feature_column_specs with respect to this particular model.
*
*
*
* repeated .google.cloud.automl.v1beta1.TablesModelColumnInfo tables_model_column_info = 5;
*
*/
public int getTablesModelColumnInfoCount() {
if (tablesModelColumnInfoBuilder_ == null) {
return tablesModelColumnInfo_.size();
} else {
return tablesModelColumnInfoBuilder_.getCount();
}
}
/**
*
*
*
* Output only. Auxiliary information for each of the
* input_feature_column_specs with respect to this particular model.
*
*
*
* repeated .google.cloud.automl.v1beta1.TablesModelColumnInfo tables_model_column_info = 5;
*
*/
public com.google.cloud.automl.v1beta1.TablesModelColumnInfo getTablesModelColumnInfo(
int index) {
if (tablesModelColumnInfoBuilder_ == null) {
return tablesModelColumnInfo_.get(index);
} else {
return tablesModelColumnInfoBuilder_.getMessage(index);
}
}
/**
*
*
*
* Output only. Auxiliary information for each of the
* input_feature_column_specs with respect to this particular model.
*
*
*
* repeated .google.cloud.automl.v1beta1.TablesModelColumnInfo tables_model_column_info = 5;
*
*/
public Builder setTablesModelColumnInfo(
int index, com.google.cloud.automl.v1beta1.TablesModelColumnInfo value) {
if (tablesModelColumnInfoBuilder_ == null) {
if (value == null) {
throw new NullPointerException();
}
ensureTablesModelColumnInfoIsMutable();
tablesModelColumnInfo_.set(index, value);
onChanged();
} else {
tablesModelColumnInfoBuilder_.setMessage(index, value);
}
return this;
}
/**
*
*
*
* Output only. Auxiliary information for each of the
* input_feature_column_specs with respect to this particular model.
*
*
*
* repeated .google.cloud.automl.v1beta1.TablesModelColumnInfo tables_model_column_info = 5;
*
*/
public Builder setTablesModelColumnInfo(
int index, com.google.cloud.automl.v1beta1.TablesModelColumnInfo.Builder builderForValue) {
if (tablesModelColumnInfoBuilder_ == null) {
ensureTablesModelColumnInfoIsMutable();
tablesModelColumnInfo_.set(index, builderForValue.build());
onChanged();
} else {
tablesModelColumnInfoBuilder_.setMessage(index, builderForValue.build());
}
return this;
}
/**
*
*
*
* Output only. Auxiliary information for each of the
* input_feature_column_specs with respect to this particular model.
*
*
*
* repeated .google.cloud.automl.v1beta1.TablesModelColumnInfo tables_model_column_info = 5;
*
*/
public Builder addTablesModelColumnInfo(
com.google.cloud.automl.v1beta1.TablesModelColumnInfo value) {
if (tablesModelColumnInfoBuilder_ == null) {
if (value == null) {
throw new NullPointerException();
}
ensureTablesModelColumnInfoIsMutable();
tablesModelColumnInfo_.add(value);
onChanged();
} else {
tablesModelColumnInfoBuilder_.addMessage(value);
}
return this;
}
/**
*
*
*
* Output only. Auxiliary information for each of the
* input_feature_column_specs with respect to this particular model.
*
*
*
* repeated .google.cloud.automl.v1beta1.TablesModelColumnInfo tables_model_column_info = 5;
*
*/
public Builder addTablesModelColumnInfo(
int index, com.google.cloud.automl.v1beta1.TablesModelColumnInfo value) {
if (tablesModelColumnInfoBuilder_ == null) {
if (value == null) {
throw new NullPointerException();
}
ensureTablesModelColumnInfoIsMutable();
tablesModelColumnInfo_.add(index, value);
onChanged();
} else {
tablesModelColumnInfoBuilder_.addMessage(index, value);
}
return this;
}
/**
*
*
*
* Output only. Auxiliary information for each of the
* input_feature_column_specs with respect to this particular model.
*
*
*
* repeated .google.cloud.automl.v1beta1.TablesModelColumnInfo tables_model_column_info = 5;
*
*/
public Builder addTablesModelColumnInfo(
com.google.cloud.automl.v1beta1.TablesModelColumnInfo.Builder builderForValue) {
if (tablesModelColumnInfoBuilder_ == null) {
ensureTablesModelColumnInfoIsMutable();
tablesModelColumnInfo_.add(builderForValue.build());
onChanged();
} else {
tablesModelColumnInfoBuilder_.addMessage(builderForValue.build());
}
return this;
}
/**
*
*
*
* Output only. Auxiliary information for each of the
* input_feature_column_specs with respect to this particular model.
*
*
*
* repeated .google.cloud.automl.v1beta1.TablesModelColumnInfo tables_model_column_info = 5;
*
*/
public Builder addTablesModelColumnInfo(
int index, com.google.cloud.automl.v1beta1.TablesModelColumnInfo.Builder builderForValue) {
if (tablesModelColumnInfoBuilder_ == null) {
ensureTablesModelColumnInfoIsMutable();
tablesModelColumnInfo_.add(index, builderForValue.build());
onChanged();
} else {
tablesModelColumnInfoBuilder_.addMessage(index, builderForValue.build());
}
return this;
}
/**
*
*
*
* Output only. Auxiliary information for each of the
* input_feature_column_specs with respect to this particular model.
*
*
*
* repeated .google.cloud.automl.v1beta1.TablesModelColumnInfo tables_model_column_info = 5;
*
*/
public Builder addAllTablesModelColumnInfo(
java.lang.Iterable extends com.google.cloud.automl.v1beta1.TablesModelColumnInfo>
values) {
if (tablesModelColumnInfoBuilder_ == null) {
ensureTablesModelColumnInfoIsMutable();
com.google.protobuf.AbstractMessageLite.Builder.addAll(values, tablesModelColumnInfo_);
onChanged();
} else {
tablesModelColumnInfoBuilder_.addAllMessages(values);
}
return this;
}
/**
*
*
*
* Output only. Auxiliary information for each of the
* input_feature_column_specs with respect to this particular model.
*
*
*
* repeated .google.cloud.automl.v1beta1.TablesModelColumnInfo tables_model_column_info = 5;
*
*/
public Builder clearTablesModelColumnInfo() {
if (tablesModelColumnInfoBuilder_ == null) {
tablesModelColumnInfo_ = java.util.Collections.emptyList();
bitField0_ = (bitField0_ & ~0x00000002);
onChanged();
} else {
tablesModelColumnInfoBuilder_.clear();
}
return this;
}
/**
*
*
*
* Output only. Auxiliary information for each of the
* input_feature_column_specs with respect to this particular model.
*
*
*
* repeated .google.cloud.automl.v1beta1.TablesModelColumnInfo tables_model_column_info = 5;
*
*/
public Builder removeTablesModelColumnInfo(int index) {
if (tablesModelColumnInfoBuilder_ == null) {
ensureTablesModelColumnInfoIsMutable();
tablesModelColumnInfo_.remove(index);
onChanged();
} else {
tablesModelColumnInfoBuilder_.remove(index);
}
return this;
}
/**
*
*
*
* Output only. Auxiliary information for each of the
* input_feature_column_specs with respect to this particular model.
*
*
*
* repeated .google.cloud.automl.v1beta1.TablesModelColumnInfo tables_model_column_info = 5;
*
*/
public com.google.cloud.automl.v1beta1.TablesModelColumnInfo.Builder
getTablesModelColumnInfoBuilder(int index) {
return getTablesModelColumnInfoFieldBuilder().getBuilder(index);
}
/**
*
*
*
* Output only. Auxiliary information for each of the
* input_feature_column_specs with respect to this particular model.
*
*
*
* repeated .google.cloud.automl.v1beta1.TablesModelColumnInfo tables_model_column_info = 5;
*
*/
public com.google.cloud.automl.v1beta1.TablesModelColumnInfoOrBuilder
getTablesModelColumnInfoOrBuilder(int index) {
if (tablesModelColumnInfoBuilder_ == null) {
return tablesModelColumnInfo_.get(index);
} else {
return tablesModelColumnInfoBuilder_.getMessageOrBuilder(index);
}
}
/**
*
*
*
* Output only. Auxiliary information for each of the
* input_feature_column_specs with respect to this particular model.
*
*
*
* repeated .google.cloud.automl.v1beta1.TablesModelColumnInfo tables_model_column_info = 5;
*
*/
public java.util.List extends com.google.cloud.automl.v1beta1.TablesModelColumnInfoOrBuilder>
getTablesModelColumnInfoOrBuilderList() {
if (tablesModelColumnInfoBuilder_ != null) {
return tablesModelColumnInfoBuilder_.getMessageOrBuilderList();
} else {
return java.util.Collections.unmodifiableList(tablesModelColumnInfo_);
}
}
/**
*
*
*
* Output only. Auxiliary information for each of the
* input_feature_column_specs with respect to this particular model.
*
*
*
* repeated .google.cloud.automl.v1beta1.TablesModelColumnInfo tables_model_column_info = 5;
*
*/
public com.google.cloud.automl.v1beta1.TablesModelColumnInfo.Builder
addTablesModelColumnInfoBuilder() {
return getTablesModelColumnInfoFieldBuilder()
.addBuilder(com.google.cloud.automl.v1beta1.TablesModelColumnInfo.getDefaultInstance());
}
/**
*
*
*
* Output only. Auxiliary information for each of the
* input_feature_column_specs with respect to this particular model.
*
*
*
* repeated .google.cloud.automl.v1beta1.TablesModelColumnInfo tables_model_column_info = 5;
*
*/
public com.google.cloud.automl.v1beta1.TablesModelColumnInfo.Builder
addTablesModelColumnInfoBuilder(int index) {
return getTablesModelColumnInfoFieldBuilder()
.addBuilder(
index, com.google.cloud.automl.v1beta1.TablesModelColumnInfo.getDefaultInstance());
}
/**
*
*
*
* Output only. Auxiliary information for each of the
* input_feature_column_specs with respect to this particular model.
*
*
*
* repeated .google.cloud.automl.v1beta1.TablesModelColumnInfo tables_model_column_info = 5;
*
*/
public java.util.List
getTablesModelColumnInfoBuilderList() {
return getTablesModelColumnInfoFieldBuilder().getBuilderList();
}
private com.google.protobuf.RepeatedFieldBuilderV3<
com.google.cloud.automl.v1beta1.TablesModelColumnInfo,
com.google.cloud.automl.v1beta1.TablesModelColumnInfo.Builder,
com.google.cloud.automl.v1beta1.TablesModelColumnInfoOrBuilder>
getTablesModelColumnInfoFieldBuilder() {
if (tablesModelColumnInfoBuilder_ == null) {
tablesModelColumnInfoBuilder_ =
new com.google.protobuf.RepeatedFieldBuilderV3<
com.google.cloud.automl.v1beta1.TablesModelColumnInfo,
com.google.cloud.automl.v1beta1.TablesModelColumnInfo.Builder,
com.google.cloud.automl.v1beta1.TablesModelColumnInfoOrBuilder>(
tablesModelColumnInfo_,
((bitField0_ & 0x00000002) != 0),
getParentForChildren(),
isClean());
tablesModelColumnInfo_ = null;
}
return tablesModelColumnInfoBuilder_;
}
private long trainBudgetMilliNodeHours_;
/**
*
*
*
* Required. The train budget of creating this model, expressed in milli node
* hours i.e. 1,000 value in this field means 1 node hour.
* The training cost of the model will not exceed this budget. The final cost
* will be attempted to be close to the budget, though may end up being (even)
* noticeably smaller - at the backend's discretion. This especially may
* happen when further model training ceases to provide any improvements.
* If the budget is set to a value known to be insufficient to train a
* model for the given dataset, the training won't be attempted and
* will error.
* The train budget must be between 1,000 and 72,000 milli node hours,
* inclusive.
*
*
* int64 train_budget_milli_node_hours = 6;
*
* @return The trainBudgetMilliNodeHours.
*/
@java.lang.Override
public long getTrainBudgetMilliNodeHours() {
return trainBudgetMilliNodeHours_;
}
/**
*
*
*
* Required. The train budget of creating this model, expressed in milli node
* hours i.e. 1,000 value in this field means 1 node hour.
* The training cost of the model will not exceed this budget. The final cost
* will be attempted to be close to the budget, though may end up being (even)
* noticeably smaller - at the backend's discretion. This especially may
* happen when further model training ceases to provide any improvements.
* If the budget is set to a value known to be insufficient to train a
* model for the given dataset, the training won't be attempted and
* will error.
* The train budget must be between 1,000 and 72,000 milli node hours,
* inclusive.
*
*
* int64 train_budget_milli_node_hours = 6;
*
* @param value The trainBudgetMilliNodeHours to set.
* @return This builder for chaining.
*/
public Builder setTrainBudgetMilliNodeHours(long value) {
trainBudgetMilliNodeHours_ = value;
onChanged();
return this;
}
/**
*
*
*
* Required. The train budget of creating this model, expressed in milli node
* hours i.e. 1,000 value in this field means 1 node hour.
* The training cost of the model will not exceed this budget. The final cost
* will be attempted to be close to the budget, though may end up being (even)
* noticeably smaller - at the backend's discretion. This especially may
* happen when further model training ceases to provide any improvements.
* If the budget is set to a value known to be insufficient to train a
* model for the given dataset, the training won't be attempted and
* will error.
* The train budget must be between 1,000 and 72,000 milli node hours,
* inclusive.
*
*
* int64 train_budget_milli_node_hours = 6;
*
* @return This builder for chaining.
*/
public Builder clearTrainBudgetMilliNodeHours() {
trainBudgetMilliNodeHours_ = 0L;
onChanged();
return this;
}
private long trainCostMilliNodeHours_;
/**
*
*
*
* Output only. The actual training cost of the model, expressed in milli
* node hours, i.e. 1,000 value in this field means 1 node hour. Guaranteed
* to not exceed the train budget.
*
*
* int64 train_cost_milli_node_hours = 7;
*
* @return The trainCostMilliNodeHours.
*/
@java.lang.Override
public long getTrainCostMilliNodeHours() {
return trainCostMilliNodeHours_;
}
/**
*
*
*
* Output only. The actual training cost of the model, expressed in milli
* node hours, i.e. 1,000 value in this field means 1 node hour. Guaranteed
* to not exceed the train budget.
*
*
* int64 train_cost_milli_node_hours = 7;
*
* @param value The trainCostMilliNodeHours to set.
* @return This builder for chaining.
*/
public Builder setTrainCostMilliNodeHours(long value) {
trainCostMilliNodeHours_ = value;
onChanged();
return this;
}
/**
*
*
*
* Output only. The actual training cost of the model, expressed in milli
* node hours, i.e. 1,000 value in this field means 1 node hour. Guaranteed
* to not exceed the train budget.
*
*
* int64 train_cost_milli_node_hours = 7;
*
* @return This builder for chaining.
*/
public Builder clearTrainCostMilliNodeHours() {
trainCostMilliNodeHours_ = 0L;
onChanged();
return this;
}
private boolean disableEarlyStopping_;
/**
*
*
*
* Use the entire training budget. This disables the early stopping feature.
* By default, the early stopping feature is enabled, which means that AutoML
* Tables might stop training before the entire training budget has been used.
*
*
* bool disable_early_stopping = 12;
*
* @return The disableEarlyStopping.
*/
@java.lang.Override
public boolean getDisableEarlyStopping() {
return disableEarlyStopping_;
}
/**
*
*
*
* Use the entire training budget. This disables the early stopping feature.
* By default, the early stopping feature is enabled, which means that AutoML
* Tables might stop training before the entire training budget has been used.
*
*
* bool disable_early_stopping = 12;
*
* @param value The disableEarlyStopping to set.
* @return This builder for chaining.
*/
public Builder setDisableEarlyStopping(boolean value) {
disableEarlyStopping_ = value;
onChanged();
return this;
}
/**
*
*
*
* Use the entire training budget. This disables the early stopping feature.
* By default, the early stopping feature is enabled, which means that AutoML
* Tables might stop training before the entire training budget has been used.
*
*
* bool disable_early_stopping = 12;
*
* @return This builder for chaining.
*/
public Builder clearDisableEarlyStopping() {
disableEarlyStopping_ = false;
onChanged();
return this;
}
@java.lang.Override
public final Builder setUnknownFields(final com.google.protobuf.UnknownFieldSet unknownFields) {
return super.setUnknownFields(unknownFields);
}
@java.lang.Override
public final Builder mergeUnknownFields(
final com.google.protobuf.UnknownFieldSet unknownFields) {
return super.mergeUnknownFields(unknownFields);
}
// @@protoc_insertion_point(builder_scope:google.cloud.automl.v1beta1.TablesModelMetadata)
}
// @@protoc_insertion_point(class_scope:google.cloud.automl.v1beta1.TablesModelMetadata)
private static final com.google.cloud.automl.v1beta1.TablesModelMetadata DEFAULT_INSTANCE;
static {
DEFAULT_INSTANCE = new com.google.cloud.automl.v1beta1.TablesModelMetadata();
}
public static com.google.cloud.automl.v1beta1.TablesModelMetadata getDefaultInstance() {
return DEFAULT_INSTANCE;
}
private static final com.google.protobuf.Parser PARSER =
new com.google.protobuf.AbstractParser() {
@java.lang.Override
public TablesModelMetadata parsePartialFrom(
com.google.protobuf.CodedInputStream input,
com.google.protobuf.ExtensionRegistryLite extensionRegistry)
throws com.google.protobuf.InvalidProtocolBufferException {
Builder builder = newBuilder();
try {
builder.mergeFrom(input, extensionRegistry);
} catch (com.google.protobuf.InvalidProtocolBufferException e) {
throw e.setUnfinishedMessage(builder.buildPartial());
} catch (com.google.protobuf.UninitializedMessageException e) {
throw e.asInvalidProtocolBufferException().setUnfinishedMessage(builder.buildPartial());
} catch (java.io.IOException e) {
throw new com.google.protobuf.InvalidProtocolBufferException(e)
.setUnfinishedMessage(builder.buildPartial());
}
return builder.buildPartial();
}
};
public static com.google.protobuf.Parser parser() {
return PARSER;
}
@java.lang.Override
public com.google.protobuf.Parser getParserForType() {
return PARSER;
}
@java.lang.Override
public com.google.cloud.automl.v1beta1.TablesModelMetadata getDefaultInstanceForType() {
return DEFAULT_INSTANCE;
}
}
© 2015 - 2025 Weber Informatics LLC | Privacy Policy