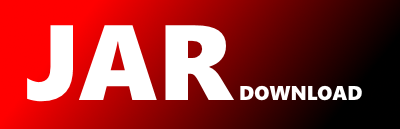
com.caseystella.analytics.outlier.batch.rpca.AugmentedDickeyFuller Maven / Gradle / Ivy
/**
* Copyright (C) 2016 Hurence ([email protected])
*
* Licensed under the Apache License, Version 2.0 (the "License");
* you may not use this file except in compliance with the License.
* You may obtain a copy of the License at
*
* http://www.apache.org/licenses/LICENSE-2.0
*
* Unless required by applicable law or agreed to in writing, software
* distributed under the License is distributed on an "AS IS" BASIS,
* WITHOUT WARRANTIES OR CONDITIONS OF ANY KIND, either express or implied.
* See the License for the specific language governing permissions and
* limitations under the License.
*/
package com.caseystella.analytics.outlier.batch.rpca;
import org.apache.commons.math3.linear.MatrixUtils;
import org.apache.commons.math3.linear.RealMatrix;
import org.apache.commons.math3.linear.RealVector;
public class AugmentedDickeyFuller {
private double[] ts;
private int lag;
private boolean needsDiff = true;
private double[] zeroPaddedDiff;
private double PVALUE_THRESHOLD = -3.45;
/**
* Uses the Augmented Dickey Fuller test to determine
* if ts is a stationary time series
* @param ts
* @param lag
*/
public AugmentedDickeyFuller(double[] ts, int lag) {
this.ts = ts;
this.lag = lag;
computeADFStatistics();
}
/**
* Uses the Augmented Dickey Fuller test to determine
* if ts is a stationary time series
* @param ts
*/
public AugmentedDickeyFuller(double[] ts) {
this.ts = ts;
this.lag = (int) Math.floor(Math.cbrt((ts.length - 1)));
computeADFStatistics();
}
private void computeADFStatistics() {
double[] y = diff(ts);
RealMatrix designMatrix = null;
int k = lag+1;
int n = ts.length - 1;
RealMatrix z = MatrixUtils.createRealMatrix(laggedMatrix(y, k)); //has rows length(ts) - 1 - k + 1
RealVector zcol1 = z.getColumnVector(0); //has length length(ts) - 1 - k + 1
double[] xt1 = subsetArray(ts, k-1, n-1); //ts[k:(length(ts) - 1)], has length length(ts) - 1 - k + 1
double[] trend = sequence(k,n); //trend k:n, has length length(ts) - 1 - k + 1
if (k > 1) {
RealMatrix yt1 = z.getSubMatrix(0, ts.length - 1 - k, 1, k-1); //same as z but skips first column
//build design matrix as cbind(xt1, 1, trend, yt1)
designMatrix = MatrixUtils.createRealMatrix(ts.length - 1 - k + 1, 3 + k - 1);
designMatrix.setColumn(0, xt1);
designMatrix.setColumn(1, ones(ts.length - 1 - k + 1));
designMatrix.setColumn(2, trend);
designMatrix.setSubMatrix(yt1.getData(), 0, 3);
} else {
//build design matrix as cbind(xt1, 1, tt)
designMatrix = MatrixUtils.createRealMatrix(ts.length - 1 - k + 1, 3);
designMatrix.setColumn(0, xt1);
designMatrix.setColumn(1, ones(ts.length - 1 - k + 1));
designMatrix.setColumn(2, trend);
}
/*OLSMultipleLinearRegression regression = new OLSMultipleLinearRegression();
regression.setNoIntercept(true);
regression.newSampleData(zcol1.toArray(), designMatrix.getData());
double[] beta = regression.estimateRegressionParameters();
double[] sd = regression.estimateRegressionParametersStandardErrors();
*/
RidgeRegression regression = new RidgeRegression(designMatrix.getData(), zcol1.toArray());
regression.updateCoefficients(.0001);
double[] beta = regression.getCoefficients();
double[] sd = regression.getStandarderrors();
double t = beta[0] / sd[0];
if (t <= PVALUE_THRESHOLD) {
this.needsDiff = true;
} else {
this.needsDiff = false;
}
}
/**
* Takes finite differences of x
* @param x
* @return Returns an array of length x.length-1 of
* the first differences of x
*/
private double[] diff(double[] x) {
double[] diff = new double[x.length - 1];
double[] zeroPaddedDiff = new double[x.length];
zeroPaddedDiff[0] = 0;
for (int i = 0; i < diff.length; i++) {
double diff_i = x[i+1] - x[i];
diff[i] = diff_i;
zeroPaddedDiff[i+1] = diff_i;
}
this.zeroPaddedDiff = zeroPaddedDiff;
return diff;
}
/**
* Equivalent to matlab and python ones
* @param n
* @return an array of doubles of length n that are
* initialized to 1
*/
private double[] ones(int n) {
double[] ones = new double[n];
for (int i = 0; i < n; i++) {
ones[i] = 1;
}
return ones;
}
/**
* Equivalent to R's embed function
* @param x time series vector
* @param lag number of lags, where lag=1 is the same as no lags
* @return a matrix that has x.length - lag + 1 rows by lag columns.
*/
private double[][] laggedMatrix(double[]x, int lag) {
double[][] laggedMatrix = new double[x.length - lag + 1][lag];
for (int j = 0; j < lag; j++) { //loop through columns
for (int i = 0; i < laggedMatrix.length; i++) {
laggedMatrix[i][j] = x[lag - j - 1 + i];
}
}
return laggedMatrix;
}
/**
* Takes x[start] through x[end - 1]
* @param x
* @param start
* @param end
* @return
*/
private double[] subsetArray(double[] x, int start, int end) {
double[] subset = new double[end - start + 1];
System.arraycopy(x, start, subset, 0, end - start + 1);
return subset;
}
/**
* Generates a sequence of ints [start, end]
* @param start
* @param end
* @return
*/
private double[] sequence(int start, int end) {
double[] sequence = new double[end - start + 1];
for (int i = start; i <= end; i++) {
sequence[i - start] = i;
}
return sequence;
}
public boolean isNeedsDiff() {
return needsDiff;
}
public double[] getZeroPaddedDiff() {
return zeroPaddedDiff;
}
}
© 2015 - 2025 Weber Informatics LLC | Privacy Policy