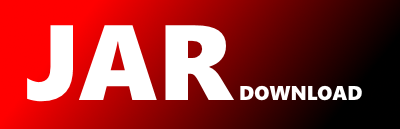
com.caseystella.analytics.outlier.batch.rpca.RPCA Maven / Gradle / Ivy
/**
* Copyright (C) 2016 Hurence ([email protected])
*
* Licensed under the Apache License, Version 2.0 (the "License");
* you may not use this file except in compliance with the License.
* You may obtain a copy of the License at
*
* http://www.apache.org/licenses/LICENSE-2.0
*
* Unless required by applicable law or agreed to in writing, software
* distributed under the License is distributed on an "AS IS" BASIS,
* WITHOUT WARRANTIES OR CONDITIONS OF ANY KIND, either express or implied.
* See the License for the specific language governing permissions and
* limitations under the License.
*/
package com.caseystella.analytics.outlier.batch.rpca;
import org.apache.commons.math3.linear.MatrixUtils;
import org.apache.commons.math3.linear.RealMatrix;
import org.apache.commons.math3.linear.SingularValueDecomposition;
import org.apache.commons.math3.stat.descriptive.DescriptiveStatistics;
public class RPCA {
private RealMatrix X;
private RealMatrix L;
private RealMatrix S;
private RealMatrix E;
private double lpenalty;
private double spenalty;
private static final int MAX_ITERS = 228;
public RPCA(double[][] data, double lpenalty, double spenalty) {
this.X = MatrixUtils.createRealMatrix(data);
this.lpenalty = lpenalty;
this.spenalty = spenalty;
initMatrices();
computeRSVD();
}
public RPCA(RealMatrix X, double lpenalty, double spenalty) {
this.X = X;
this.lpenalty = lpenalty;
this.spenalty = spenalty;
initMatrices();
computeRSVD();
}
private void initMatrices() {
this.L = MatrixUtils.createRealMatrix(this.X.getRowDimension(), this.X.getColumnDimension());
this.S = MatrixUtils.createRealMatrix(this.X.getRowDimension(), this.X.getColumnDimension());
this.E = MatrixUtils.createRealMatrix(this.X.getRowDimension(), this.X.getColumnDimension());
}
private void computeRSVD() {
double mu = X.getColumnDimension() * X.getRowDimension() / (4 * l1norm(X.getData()));
double objPrev = 0.5*Math.pow(X.getFrobeniusNorm(), 2);
double obj = objPrev;
double tol = 1e-8 * objPrev;
double diff = 2 * tol;
int iter = 0;
while(diff > tol && iter < MAX_ITERS) {
double nuclearNorm = computeS(mu);
double l1Norm = computeL(mu);
double l2Norm = computeE();
obj = computeObjective(nuclearNorm, l1Norm, l2Norm);
diff = Math.abs(objPrev - obj);
objPrev = obj;
mu = computeDynamicMu();
iter = iter + 1;
}
}
private double[] softThreshold(double[] x, double penalty) {
for(int i = 0; i < x.length; i++) {
x[i] = Math.signum(x[i]) * Math.max(Math.abs(x[i]) - penalty, 0);
}
return x;
}
private double[][] softThreshold(double[][] x, double penalty) {
for(int i = 0; i < x.length; i++) {
for(int j = 0; j < x[i].length; j++) {
x[i][j] = Math.signum(x[i][j]) * Math.max(Math.abs(x[i][j]) - penalty, 0);
}
}
return x;
}
private double sum(double[] x) {
double sum = 0;
for (int i = 0; i < x.length; i++)
sum += x[i];
return (sum);
}
private double l1norm(double[][] x) {
double l1norm = 0;
for (int i = 0; i < x.length; i++) {
for (int j = 0; j < x[i].length; j++) {
l1norm += Math.abs(x[i][j]);
}
}
return l1norm;
}
private double computeL(double mu) {
double LPenalty = lpenalty * mu;
SingularValueDecomposition svd = new SingularValueDecomposition(X.subtract(S));
double[] penalizedD = softThreshold(svd.getSingularValues(), LPenalty);
RealMatrix D_matrix = MatrixUtils.createRealDiagonalMatrix(penalizedD);
L = svd.getU().multiply(D_matrix).multiply(svd.getVT());
return sum(penalizedD) * LPenalty;
}
private double computeS(double mu) {
double SPenalty = spenalty * mu;
double[][] penalizedS = softThreshold(X.subtract(L).getData(), SPenalty);
S = MatrixUtils.createRealMatrix(penalizedS);
return l1norm(penalizedS) * SPenalty;
}
private double computeE() {
E = X.subtract(L).subtract(S);
double norm = E.getFrobeniusNorm();
return Math.pow(norm, 2);
}
private double computeObjective(double nuclearnorm, double l1norm, double l2norm) {
return 0.5*l2norm + nuclearnorm + l1norm;
}
private double computeDynamicMu() {
int m = E.getRowDimension();
int n = E.getColumnDimension();
double E_sd = standardDeviation(E.getData());
double mu = E_sd * Math.sqrt(2*Math.max(m,n));
return Math.max(.01, mu);
}
/*private double MedianAbsoluteDeviation(double[][] x) {
DescriptiveStatistics stats = new DescriptiveStatistics();
for (int i = 0; i < x.length; i ++)
for (int j = 0; j < x[i].length; j++)
stats.addValue(x[i][j]);
double median = stats.getPercentile(50);
DescriptiveStatistics absoluteDeviationStats = new DescriptiveStatistics();
for (int i = 0; i < x.length; i ++)
for (int j = 0; j < x[i].length; j++)
absoluteDeviationStats.addValue(Math.abs(x[i][j] - median));
return absoluteDeviationStats.getPercentile(50) * 1.4826;
}*/
private double standardDeviation(double[][] x) {
DescriptiveStatistics stats = new DescriptiveStatistics();
for (int i = 0; i < x.length; i ++)
for (int j = 0; j < x[i].length; j++)
stats.addValue(x[i][j]);
return stats.getStandardDeviation();
}
public RealMatrix getL() {
return L;
}
public RealMatrix getS() {
return S;
}
public RealMatrix getE() {
return E;
}
}
© 2015 - 2025 Weber Informatics LLC | Privacy Policy