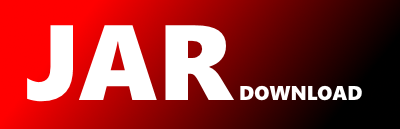
com.komputation.cpu.layers.forward.units.ShortTermResponse.kt Maven / Gradle / Ivy
Go to download
Show more of this group Show more artifacts with this name
Show all versions of komputation Show documentation
Show all versions of komputation Show documentation
Komputation is a neural network framework for the JVM written in the Kotlin programming language.
package com.komputation.cpu.layers.forward.units
import com.komputation.cpu.layers.combination.AdditionCombination
import com.komputation.cpu.layers.combination.HadamardCombination
import com.komputation.cpu.layers.combination.hadamardCombination
import com.komputation.cpu.layers.forward.activation.CpuTanhLayer
import com.komputation.cpu.layers.forward.projection.SeriesBias
import com.komputation.cpu.layers.forward.projection.SeriesWeighting
import com.komputation.cpu.layers.forward.projection.seriesBias
import com.komputation.cpu.layers.forward.projection.seriesWeighting
import com.komputation.initialization.InitializationStrategy
import com.komputation.layers.concatenateNames
import com.komputation.layers.forward.activation.tanhLayer
import com.komputation.optimization.OptimizationInstruction
class ShortTermResponse(
private val forgetting: Array,
private val memoryWeighting: SeriesWeighting,
private val inputWeighting: SeriesWeighting,
private val additions: Array,
private val bias: SeriesBias?,
private val activations: Array) {
fun getMemoryWeights() =
this.memoryWeighting.getWeights()
fun getInputWeights() =
this.inputWeighting.getWeights()
fun getBias() =
this.bias
fun forward(withinBatch : Int, step : Int, state : FloatArray, input : FloatArray, forget : FloatArray, isTraining : Boolean): FloatArray {
val shortTermMemory = this.forgetting[step].forward(state, forget)
val weightedShortTermMemory = this.memoryWeighting.forwardStep(withinBatch, step, shortTermMemory, isTraining)
val weightedInput = this.inputWeighting.forwardStep(withinBatch, step, input, isTraining)
val addition = this.additions[step].forward(weightedShortTermMemory, weightedInput)
val preActivation =
if (this.bias == null) {
addition
}
else {
this.bias.forwardStep(withinBatch, step, addition, isTraining)
}
return this.activations[step].forward(withinBatch, 1, preActivation, isTraining)
}
fun backward(withinBatch : Int, step: Int, chain: FloatArray): Pair> {
// short-term response = tanh(short-term response pre-activation)
// d short-term response / d short-term response pre-activation
val activation = this.activations[step]
activation.backward(withinBatch, chain)
val backwardShortTermResponseWrtPreActivation = activation.backwardResult
// short-term response pre-activation = weighted short-term memory + weighted input (+ short-term bias)
// d short-term response pre-activation / d weighted short-term memory
val backwardPreActivationWrtWeightedShortTermMemory = this.additions[step].backwardFirst(backwardShortTermResponseWrtPreActivation)
// d weighted short-term memory / d short-term memory
this.memoryWeighting.backwardStep(withinBatch, step, backwardPreActivationWrtWeightedShortTermMemory)
val backwardWeightedShortTermMemoryWrtShortTermMemory = this.memoryWeighting.backwardResult
// d short-term memory / d forget
val backwardShortTermMemoryWrtForget = this.forgetting[step].backwardFirst(backwardWeightedShortTermMemoryWrtShortTermMemory)
// d short-term memory / d previous state
val backwardShortTermMemoryWrtPreviousState = this.forgetting[step].backwardFirst(backwardWeightedShortTermMemoryWrtShortTermMemory)
// d short-term response pre-activation / d short-term weighted input
val backwardPreActivationWrtWeightedInput = this.additions[step].backwardSecond(backwardShortTermResponseWrtPreActivation)
// d short-term weighted input / d weighted input
this.inputWeighting.backwardStep(withinBatch, step, backwardPreActivationWrtWeightedInput)
val backwardWeightedInputWrtInput = this.inputWeighting.backwardResult
if (this.bias != null) {
this.bias.backwardStep(withinBatch, step, backwardShortTermResponseWrtPreActivation)
}
return backwardShortTermMemoryWrtForget to (backwardShortTermMemoryWrtPreviousState to backwardWeightedInputWrtInput)
}
fun backwardSeries() {
this.memoryWeighting.backwardSeries()
this.inputWeighting.backwardSeries()
this.bias?.backwardSeries()
}
fun optimize(batchSize : Int) {
this.memoryWeighting.optimize(batchSize)
this.inputWeighting.optimize(batchSize)
this.bias?.optimize(batchSize)
}
}
fun shortTermResponse(
name : String,
numberSteps : Int,
hiddenDimension : Int,
inputDimension : Int,
memoryWeightInitializationStrategy : InitializationStrategy,
inputWeightInitializationStrategy : InitializationStrategy,
biasInitializationStrategy: InitializationStrategy?,
optimizationStrategy : OptimizationInstruction?): ShortTermResponse {
val shortTermForgetting = Array(numberSteps) { indexStep ->
val shortTermForgettingName = concatenateNames(name, "forgetting-step-$indexStep")
hadamardCombination(shortTermForgettingName, hiddenDimension, 1)
}
val shortTermMemoryWeightingSeriesName = concatenateNames(name, "memory-weighting")
val shortTermMemoryWeightingStepName = concatenateNames(name, "memory-weighting-step")
val shortTermMemoryWeighting = seriesWeighting(shortTermMemoryWeightingSeriesName, shortTermMemoryWeightingStepName, numberSteps, true, hiddenDimension, 1, hiddenDimension, memoryWeightInitializationStrategy, optimizationStrategy)
val shortTermInputWeightingSeriesName = concatenateNames(name, "input-weighting")
val shortTermInputWeightingStepName = concatenateNames(name, "input-weighting-step")
val shortTermInputWeighting = seriesWeighting(shortTermInputWeightingSeriesName, shortTermInputWeightingStepName, numberSteps, false, inputDimension, 1, hiddenDimension, inputWeightInitializationStrategy, optimizationStrategy)
val shortTermAdditions = Array(numberSteps) { indexStep ->
val shortTermAdditionName = concatenateNames(name, "addition-step-$indexStep")
AdditionCombination(shortTermAdditionName, hiddenDimension, 1)
}
val shortTermBias =
if (biasInitializationStrategy != null) {
val shortTermBiasSeriesName = concatenateNames(name, "bias")
val shortTermBiasStepName = concatenateNames(shortTermBiasSeriesName, "step")
seriesBias(shortTermBiasSeriesName, shortTermBiasStepName, numberSteps, hiddenDimension, biasInitializationStrategy, optimizationStrategy)
}
else {
null
}
val shortTermActivations = Array(numberSteps) { indexStep ->
val shortTermActivationName = concatenateNames(name, "activation-step-$indexStep")
tanhLayer(shortTermActivationName, hiddenDimension, 1).buildForCpu()
}
return ShortTermResponse(shortTermForgetting, shortTermMemoryWeighting, shortTermInputWeighting, shortTermAdditions, shortTermBias, shortTermActivations)
}
© 2015 - 2025 Weber Informatics LLC | Privacy Policy