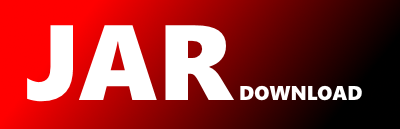
com.komputation.cuda.workflow.CudaTrainer.kt Maven / Gradle / Ivy
Go to download
Show more of this group Show more artifacts with this name
Show all versions of komputation Show documentation
Show all versions of komputation Show documentation
Komputation is a neural network framework for the JVM written in the Kotlin programming language.
package com.komputation.cuda.workflow
import jcuda.Pointer
import com.komputation.cuda.network.CudaBackwardPropagator
import com.komputation.cuda.network.CudaForwardPropagator
import com.komputation.cuda.loss.CudaLossFunction
import com.komputation.cuda.memory.InputMemory
import com.komputation.cuda.memory.TargetMemory
import com.komputation.matrix.Matrix
import com.komputation.matrix.partitionIndices
import com.komputation.optimization.Optimizable
class CudaTrainer(
private val forwardPropagator : CudaForwardPropagator,
private val backwardPropagator : CudaBackwardPropagator,
private val optimizables : Array,
private val inputs : Array,
private val targets: Array,
private val numberIterations : Int,
private val maximumBatchSize : Int,
private val lossFunction : CudaLossFunction,
private val afterEachIteration : ((index : Int, loss : Float) -> Unit)? = null) {
private val numberExamples = this.inputs.size
private val batches = partitionIndices(this.numberExamples, this.maximumBatchSize)
private val inputMemory = InputMemory()
private val targetMemory = TargetMemory(this.targets.first().size)
init {
this.lossFunction.acquire(this.maximumBatchSize)
}
fun free() {
this.lossFunction.release()
this.inputMemory.free()
this.targetMemory.free()
}
fun run(): Long {
val trackLoss = this.afterEachIteration != null
val start = System.currentTimeMillis()
repeat(this.numberIterations) { indexIteration ->
var iterationLoss = if(trackLoss) 0f else Float.NaN
for ((batchId, batch) in this.batches.withIndex()) {
val currentBatchSize = batch.size
val devicePredictions = this.forwardPropagator.forward(batchId, currentBatchSize, batch, this.inputs, this.inputMemory,true)
val pointerToDevicePredictions = Pointer.to(devicePredictions)
val pointerToTargets = this.targetMemory.get(batchId, currentBatchSize, batch, this.targets)
if (trackLoss) {
this.lossFunction.accumulate(pointerToDevicePredictions, pointerToTargets, currentBatchSize)
}
val backwardLoss = this.lossFunction.backward(pointerToDevicePredictions, pointerToTargets, currentBatchSize)
this.backwardPropagator.backward(backwardLoss, currentBatchSize)
for (optimizable in this.optimizables) {
optimizable.optimize(currentBatchSize)
}
if (trackLoss) {
val batchLoss = this.lossFunction.accessAccumulation()
iterationLoss += batchLoss
}
}
this.afterEachIteration?.invoke(indexIteration, iterationLoss)
}
val stop = System.currentTimeMillis()
val time = stop - start
return time
}
}
© 2015 - 2025 Weber Informatics LLC | Privacy Policy