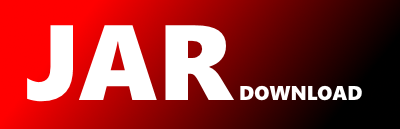
com.kotlinnlp.hanclassifier.MultiLevelHANModel.kt Maven / Gradle / Ivy
Go to download
Show more of this group Show more artifacts with this name
Show all versions of hanclassifier Show documentation
Show all versions of hanclassifier Show documentation
HANClassifier is a very simple to use text classifier which uses the Hierarchical Attention Networks (HAN)
from the SimpleDNN library.
Please wait ...