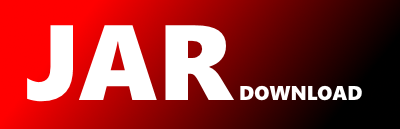
com.kotlinnlp.simplednn.core.layers.BackwardHelper.kt Maven / Gradle / Ivy
Go to download
Show more of this group Show more artifacts with this name
Show all versions of simplednn Show documentation
Show all versions of simplednn Show documentation
SimpleDNN is a machine learning lightweight open-source library written in Kotlin whose purpose is to
support the development of feed-forward and recurrent Artificial Neural Networks.
/* Copyright 2016-present The KotlinNLP Authors. All Rights Reserved.
*
* This Source Code Form is subject to the terms of the Mozilla Public
* License, v. 2.0. If a copy of the MPL was not distributed with this
* file, you can obtain one at http://mozilla.org/MPL/2.0/.
* ------------------------------------------------------------------*/
package com.kotlinnlp.simplednn.core.layers
import com.kotlinnlp.simplednn.core.arrays.AugmentedArray
import com.kotlinnlp.simplednn.simplemath.ndarray.NDArray
import com.kotlinnlp.simplednn.simplemath.ndarray.NDArrayMask
import com.kotlinnlp.simplednn.simplemath.ndarray.dense.DenseNDArray
import java.util.*
/**
* The helper which executes the backward on a [layer].
*/
interface BackwardHelper> {
/**
* The [LayerStructure] in which the backward is executed.
*/
val layer: LayerStructure
/**
* Executes the backward calculating the errors of the parameters and eventually of the input through the SGD
* algorithm, starting from the preset errors of the output array.
*
* @param paramsErrors the errors of the parameters which will be filled
* @param propagateToInput whether to propagate the errors to the input array
* @param mePropK the k factor of the 'meProp' algorithm to propagate from the k (in percentage) output nodes with
* the top errors (ignored if null, the default)
*/
fun backward(paramsErrors: LayerParameters<*>, propagateToInput: Boolean = false, mePropK: Double?)
/**
* Build the 'meProp' mask of this array.
*
* @param k the factor of the 'meProp' algorithm to extract the k (in percentage) array elements with the top errors
*
* @return the mask of the k elements with the top errors
*/
fun AugmentedArray.getMePropMask(k: Double): NDArrayMask {
val gy: DenseNDArray = this.errors
val nTopElements: Int = Math.round(k * gy.length).toInt()
val minHeap = PriorityQueue>(gy.length, Comparator({ a, b ->
val diff: Double = a.second - b.second // compare errors values
if (diff > 0.0) -1 else if (diff < 0.0) 1 else 0 // descending order
}))
(0 until gy.length).forEach { i -> minHeap.add(Pair(i, Math.abs(gy[i]))) } // pairs of
return NDArrayMask(
dim1 = Array(size = nTopElements, init = { minHeap.remove().first }).sortedArray(), // get top errors indices
dim2 = Array(size = nTopElements, init = { 0 }))
}
}
© 2015 - 2025 Weber Informatics LLC | Privacy Policy