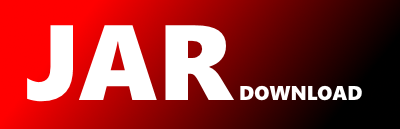
com.kotlinnlp.simplednn.deeplearning.sequencelabeling.SWSLNetwork.kt Maven / Gradle / Ivy
Go to download
Show more of this group Show more artifacts with this name
Show all versions of simplednn Show documentation
Show all versions of simplednn Show documentation
SimpleDNN is a machine learning lightweight open-source library written in Kotlin whose purpose is to
support the development of feed-forward and recurrent Artificial Neural Networks.
/* Copyright 2016-present The KotlinNLP Authors. All Rights Reserved.
*
* This Source Code Form is subject to the terms of the Mozilla Public
* License, v. 2.0. If a copy of the MPL was not distributed with this
* file, you can obtain one at http://mozilla.org/MPL/2.0/.
* ------------------------------------------------------------------*/
package com.kotlinnlp.simplednn.deeplearning.sequencelabeling
import com.kotlinnlp.simplednn.core.functionalities.activations.ActivationFunction
import com.kotlinnlp.simplednn.core.functionalities.activations.Softmax
import com.kotlinnlp.simplednn.core.functionalities.initializers.GlorotInitializer
import com.kotlinnlp.simplednn.core.functionalities.initializers.Initializer
import com.kotlinnlp.simplednn.core.layers.LayerType
import com.kotlinnlp.simplednn.core.neuralnetwork.preset.FeedforwardNeuralNetwork
import com.kotlinnlp.simplednn.deeplearning.embeddings.EmbeddingsMap
import com.kotlinnlp.simplednn.simplemath.ndarray.Shape
import com.kotlinnlp.simplednn.simplemath.ndarray.dense.DenseNDArrayFactory
import java.io.Serializable
/**
* The Neural Network for the Sliding-Window Sequence Labeling.
*
* The network, which consists of a simple feed-forward network with a final
* softmax prediction, is used as a classifier to label each element of a sequence.
*
* It classifies each element independently but uses as input features the information
* about the surrounding elements (sliding window), defined by the [leftContextSize]
* and the [rightContextSize].
* This implementation allows also to consider past network decisions at the current
* processing step: the output labels included in the [leftContextSize] are first
* transformed into trainable embeddings, then used as auxiliary input.
*
* @property elementSize the size of the dense-representation of a sequence element
* @property hiddenLayerSize size of the hidden layer
* @property hiddenLayerActivation the activation function of the hidden layer
* @property numberOfLabels the number of possible labels (the size of the output layer)
* @property leftContextSize the number of elements used to create the left context (default 3)
* @property rightContextSize the number of elements used to create the right context (default 3)
* @property labelEmbeddingSize the size of the dense-representation of a label (default 25)
* @property dropout the probability of dropout (default 0.0). If applying it, the usual value is 0.25.
* @param weightsInitializer the initializer of the weights (zeros if null, default: Glorot)
* @param biasesInitializer the initializer of the biases (zeros if null, default: Glorot)
*/
class SWSLNetwork(
val elementSize: Int,
val hiddenLayerSize: Int,
val hiddenLayerActivation: ActivationFunction?,
val numberOfLabels: Int,
val leftContextSize: Int = 3,
val rightContextSize: Int = 3,
val labelEmbeddingSize: Int = 25,
val dropout: Double = 0.0,
weightsInitializer: Initializer? = GlorotInitializer(),
biasesInitializer: Initializer? = GlorotInitializer()
) : Serializable {
companion object {
/**
* Private val used to serialize the class (needed from Serializable)
*/
@Suppress("unused")
private const val serialVersionUID: Long = 1L
}
/**
* The size of the labels embeddings representation (labelEmbeddingSize * leftContextSize)
*/
val labelsEmbeddingsSize = this.labelEmbeddingSize * this.leftContextSize
/**
* The size of input-layer, which is the concatenation of the focus element plus its context
*/
val featuresSize = this.elementSize * (this.leftContextSize + this.rightContextSize + 1) + this.labelsEmbeddingsSize
/**
* An empty vector of the size of a single label embedding representation
*/
val emptyLabelVector = DenseNDArrayFactory.zeros(Shape(this.labelEmbeddingSize))
/**
* An empty vector of the size of a single element
*/
val emptyVector = DenseNDArrayFactory.zeros(Shape(this.elementSize))
/**
* The Neural Network to process the sequence left-to-right
*/
val classifier = FeedforwardNeuralNetwork(
inputSize = this.featuresSize,
inputType = LayerType.Input.Dense,
hiddenSize = this.hiddenLayerSize,
hiddenActivation = this.hiddenLayerActivation,
outputSize = this.numberOfLabels,
outputActivation = Softmax(),
hiddenDropout = this.dropout,
weightsInitializer = weightsInitializer,
biasesInitializer = biasesInitializer
)
/**
* The embeddings associated to each output label
*/
val labelsEmbeddings = EmbeddingsMap(size = this.labelEmbeddingSize)
}