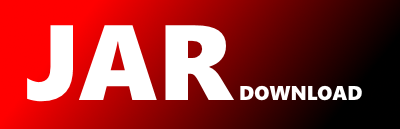
com.kotlinnlp.simplednn.deeplearning.attentiverecurrentnetwork.ForwardHelper.kt Maven / Gradle / Ivy
Go to download
Show more of this group Show more artifacts with this name
Show all versions of simplednn Show documentation
Show all versions of simplednn Show documentation
SimpleDNN is a machine learning lightweight open-source library written in Kotlin whose purpose is to
support the development of feed-forward and recurrent Artificial Neural Networks.
/* Copyright 2016-present The KotlinNLP Authors. All Rights Reserved.
*
* This Source Code Form is subject to the terms of the Mozilla Public
* License, v. 2.0. If a copy of the MPL was not distributed with this
* file, you can obtain one at http://mozilla.org/MPL/2.0/.
* ------------------------------------------------------------------*/
package com.kotlinnlp.simplednn.deeplearning.attentiverecurrentnetwork
import com.kotlinnlp.simplednn.core.arrays.AugmentedArray
import com.kotlinnlp.simplednn.core.layers.feedforward.FeedforwardLayerStructure
import com.kotlinnlp.simplednn.core.neuralprocessor.feedforward.FeedforwardNeuralProcessor
import com.kotlinnlp.simplednn.deeplearning.attentionnetwork.AttentionNetwork
import com.kotlinnlp.simplednn.simplemath.concatVectorsV
import com.kotlinnlp.simplednn.simplemath.ndarray.Shape
import com.kotlinnlp.simplednn.simplemath.ndarray.dense.DenseNDArray
import com.kotlinnlp.simplednn.simplemath.ndarray.dense.DenseNDArrayFactory
/**
* The forward helper of the [AttentiveRecurrentNetwork].
*
* @property network the attentive recurrent network of this helper
*/
class ForwardHelper(private val network: AttentiveRecurrentNetwork) {
/**
* The recurrent context vector used in first state.
*/
private val initRecurrentContext: DenseNDArray =
DenseNDArrayFactory.zeros(Shape(this.network.model.recurrentContextSize))
/**
* A boolean indicating if the current is the first state of recursion.
*/
private var firstRecurrentState: Boolean = true
/**
* @param inputSequence the input sequence
* @param lastPredictionLabel the context label vector used to encode the memory of the last prediction (can be null
* if it is the first state)
* @param firstState a boolean indicating if this is the first state
* @param initHidden the initial hidden array (null by default)
*/
fun forward(inputSequence: List,
lastPredictionLabel: DenseNDArray?,
firstState: Boolean,
initHidden: DenseNDArray? = null): DenseNDArray {
require(firstState || lastPredictionLabel != null) {
"The last prediction label cannot be null for states after the first."
}
if (firstState) this.resetHistory()
val recurrentContext: DenseNDArray = if (firstState)
initHidden ?: this.initRecurrentContext
else
this.forwardRecurrentContext(lastPredictionLabel = lastPredictionLabel!!, initHidden = initHidden)
val stateEncoding: DenseNDArray = this.encodeState(sequence = inputSequence, recurrentContext = recurrentContext)
return this.getOutputProcessor().forward(concatVectorsV(stateEncoding, recurrentContext))
}
/**
* Reset the recurrent history of the network.
*/
private fun resetHistory() {
this.firstRecurrentState = true
this.network.transformLayersPool.releaseAll()
this.network.usedTransformLayers.clear()
this.network.attentionNetworksPool.releaseAll()
this.network.usedAttentionNetworks.clear()
this.network.outputNetworkPool.releaseAll()
this.network.usedOutputProcessors.clear()
}
/***
* @param lastPredictionLabel the context label vector used to encode the memory of the last prediction
* @param initHidden the initial hidden array (can be null)
*
* @return the recurrent context for the current state
*/
private fun forwardRecurrentContext(lastPredictionLabel: DenseNDArray, initHidden: DenseNDArray?): DenseNDArray {
val output: DenseNDArray = this.network.recurrentContextProcessor.forward(
featuresArray = concatVectorsV(this.getLastStateEncoding(), lastPredictionLabel),
initHiddenArrays = if (this.firstRecurrentState) listOf(initHidden) else null,
firstState = this.firstRecurrentState)
this.firstRecurrentState = false
return output
}
/**
* Encode the current state.
*
* @param sequence the sequence to decode
* @param recurrentContext the recurrent context
*
* @return the state encoding as result of the [AttentionNetwork]
*/
private fun encodeState(sequence: List, recurrentContext: DenseNDArray): DenseNDArray {
val attentionNetwork = this.getAttentionNetwork()
return attentionNetwork.forward(
inputSequence = ArrayList(sequence.map { AugmentedArray(values = it) }),
attentionSequence = this.buildAttentionSequence(sequence = sequence, recurrentContext = recurrentContext))
}
/**
* @param sequence the input sequence
* @param recurrentContext the recurrent context
*
* @return the sequence of attention arrays
*/
private fun buildAttentionSequence(sequence: List,
recurrentContext: DenseNDArray): ArrayList {
val transformLayers = this.getTransformLayers(size = sequence.size)
return ArrayList(transformLayers.zip(sequence).map { (layer, inputArray) ->
layer.setInput(concatVectorsV(inputArray, recurrentContext))
layer.forward()
layer.outputArray.values
})
}
/**
* Get an available group of transform layers, adding it into the usedTransformLayers list.
*
* @param size the number of transform layer to build
*
* @return an available transform layer
*/
private fun getTransformLayers(size: Int): List> {
this.network.usedTransformLayers.add(
List(size = size, init = { this.network.transformLayersPool.getItem() })
)
return this.network.usedTransformLayers.last()
}
/**
* Get an available Attention Network, adding it into the usedAttentionNetworks list.
*
* @return an available Attention Network
*/
private fun getAttentionNetwork(): AttentionNetwork {
this.network.usedAttentionNetworks.add(this.network.attentionNetworksPool.getItem())
return this.network.usedAttentionNetworks.last()
}
/**
* Get an available output processor, adding it into the usedOutputProcessors list.
*
* @return an available output processor
*/
private fun getOutputProcessor(): FeedforwardNeuralProcessor {
this.network.usedOutputProcessors.add(this.network.outputNetworkPool.getItem())
return this.network.usedOutputProcessors.last()
}
/**
* @return the last state encoding
*/
private fun getLastStateEncoding(): DenseNDArray = this.network.usedAttentionNetworks.last().getOutput()
}
© 2015 - 2025 Weber Informatics LLC | Privacy Policy