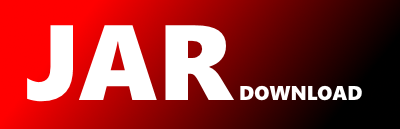
com.kotlinnlp.simplednn.deeplearning.treernn.TreeRNN.kt Maven / Gradle / Ivy
Go to download
Show more of this group Show more artifacts with this name
Show all versions of simplednn Show documentation
Show all versions of simplednn Show documentation
SimpleDNN is a machine learning lightweight open-source library written in Kotlin whose purpose is to
support the development of feed-forward and recurrent Artificial Neural Networks.
/* Copyright 2016-present The KotlinNLP Authors. All Rights Reserved.
*
* This Source Code Form is subject to the terms of the Mozilla Public
* License, v. 2.0. If a copy of the MPL was not distributed with this
* file, you can obtain one at http://mozilla.org/MPL/2.0/.
* ------------------------------------------------------------------*/
package com.kotlinnlp.simplednn.deeplearning.treernn
import com.kotlinnlp.simplednn.core.functionalities.activations.Tanh
import com.kotlinnlp.simplednn.core.functionalities.initializers.GlorotInitializer
import com.kotlinnlp.simplednn.core.functionalities.initializers.Initializer
import com.kotlinnlp.simplednn.core.layers.LayerConfiguration
import com.kotlinnlp.simplednn.core.layers.LayerType
import com.kotlinnlp.simplednn.core.neuralnetwork.NeuralNetwork
import java.io.Serializable
/**
* The TreeRNN treats the children of a node as a sequence, and encode this sequence using
* an RNN. The left-children and right-children are encoded using two separate RNNs:
* the first RNN [leftRNN] encodes the sequence of left-children from the head outwards,
* and the second RNN [rightRNN] the sequence of right-children from the head outwards.
*
* The output of the [leftRNN] and the [rightRNN] are concatenated, then the [concatNetwork]
* is used to reduced the output back to d-dimensions using a linear transformation
* followed by the non-linear activation function. This means that the TreeRNN output layer
* has the same size of the input layer.
*
* Note: the number of recurrent layers of the TreeRNN is fixed to one.
*
* @property inputLayerSize size of the input layer
* @property hiddenLayerSize size of the hidden layer
* @property hiddenLayerConnectionType type of the recurrent layer
* @param weightsInitializer the initializer of the weights (zeros if null, default: Glorot)
* @param biasesInitializer the initializer of the biases (zeros if null, default: Glorot)
*/
class TreeRNN(
val inputLayerSize: Int,
val hiddenLayerSize: Int,
val hiddenLayerConnectionType: LayerType.Connection = LayerType.Connection.GRU,
weightsInitializer: Initializer? = GlorotInitializer(),
biasesInitializer: Initializer? = GlorotInitializer()
) : Serializable {
companion object {
/**
* Private val used to serialize the class (needed from Serializable).
*/
@Suppress("unused")
private const val serialVersionUID: Long = 1L
}
/**
* The size of the output layer
*/
val outputLayerSize: Int = this.inputLayerSize
/**
* The Recurrent Neural Network to encode the left-children sequence
*/
val leftRNN = NeuralNetwork(
LayerConfiguration(size = this.inputLayerSize),
LayerConfiguration(size = this.hiddenLayerSize,
activationFunction = Tanh(), // fixed
connectionType = this.hiddenLayerConnectionType),
weightsInitializer = weightsInitializer,
biasesInitializer = biasesInitializer
)
/**
* The Recurrent Neural Network to encode the right-children sequence
*/
val rightRNN = NeuralNetwork(
LayerConfiguration(size = this.inputLayerSize),
LayerConfiguration(size = this.hiddenLayerSize,
activationFunction = Tanh(), // fixed
connectionType = this.hiddenLayerConnectionType),
weightsInitializer = weightsInitializer,
biasesInitializer = biasesInitializer
)
/**
* The output of the [leftRNN] and the [rightRNN] are concatenated, resulting in a 2d-dimensional vector,
* then the [concatNetwork] is used to reduced the output back to d-dimensions using a linear transformation
* followed by the non-linear activation function.
*/
val concatNetwork = NeuralNetwork(
LayerConfiguration(size = this.hiddenLayerSize * 2),
LayerConfiguration(size = this.outputLayerSize,
activationFunction = Tanh(), // fixed
connectionType = LayerType.Connection.Feedforward),
weightsInitializer = weightsInitializer,
biasesInitializer = biasesInitializer
)
/**
* The model of this [TreeRNN] containing its parameters.
*/
val model = TreeRNNParameters(
leftRNN = this.leftRNN.model,
rightRNN = this.rightRNN.model,
concatNetwork = this.concatNetwork.model
)
/**
* Check hidden layer connection type.
*/
init {
require(hiddenLayerConnectionType.property == LayerType.Property.Recurrent) {
"required hiddenLayerConnectionType with Recurrent property"
}
}
}